2023
Aseem Saxena; Paola Pesantez-Cabrera; Rohan Ballapragada; Kin-Ho Lam; Markus Keller; Alan Fern
Grape Cold Hardiness Prediction via Multi-Task Learning Conference
Association for the Advancement of Artificial Intelligence (AAAI) 2023, 2023.
Abstract | Links | BibTeX | Tags: Cold Hardiness, Computer and Information Sciences, Machine Learning
@conference{saxena_aaai2023,
title = {Grape Cold Hardiness Prediction via Multi-Task Learning},
author = {Aseem Saxena and Paola Pesantez-Cabrera and Rohan Ballapragada and Kin-Ho Lam and Markus Keller and Alan Fern},
url = {https://ojs.aaai.org/index.php/AAAI/article/view/26865},
doi = { https://doi.org/10.1609/aaai.v37i13.26865},
year = {2023},
date = {2023-01-01},
urldate = {2023-01-01},
booktitle = {Association for the Advancement of Artificial Intelligence (AAAI) 2023},
abstract = {Cold temperatures during fall and spring have the potential to cause frost damage to grapevines and other fruit plants, which can significantly decrease harvest yields. To help prevent these losses, farmers deploy expensive frost mitigation measures such as sprinklers, heaters, and wind machines when they judge that damage may occur. This judgment, however, is challenging because the cold hardiness of plants changes throughout the dormancy period and it is difficult to directly measure. This has led scientists to develop cold hardiness prediction models that can be tuned to different grape cultivars based on laborious field measurement data. In this paper, we study whether deep-learning models can improve cold hardiness prediction for grapes based on data that has been collected over a 30-year time period. A key challenge is that the amount of data per cultivar is highly variable, with some cultivars having only a small amount. For this purpose, we investigate the use of multi-task learning to leverage data across cultivars in order to improve prediction performance for individual cultivars. We evaluate a number of multi-task learning
approaches and show that the highest-performing approach is able to significantly improve overlearning for single cultivars and outperforms the current state-of-the-art scientific model for most cultivars.},
keywords = {Cold Hardiness, Computer and Information Sciences, Machine Learning},
pubstate = {published},
tppubtype = {conference}
}
approaches and show that the highest-performing approach is able to significantly improve overlearning for single cultivars and outperforms the current state-of-the-art scientific model for most cultivars.
Abatzoglou, John T.; McEvoy, Daniel J.; Nauslar, Nicholas J.; Hegewisch, Katherine C.; Huntington, Justin L.
Downscaled subseasonal fire danger forecast skill across the contiguous United States Journal Article
In: Atmospheric Science Letters, vol. 24, no. 8, pp. e1165, 2023, ISSN: 1530-261X, (_eprint: https://onlinelibrary.wiley.com/doi/pdf/10.1002/asl.1165).
Abstract | Links | BibTeX | Tags: Fallow, Water
@article{abatzoglou_downscaled_2023,
title = {Downscaled subseasonal fire danger forecast skill across the contiguous United States},
author = {Abatzoglou, John T. and McEvoy, Daniel J. and Nauslar, Nicholas J. and Hegewisch, Katherine C. and Huntington, Justin L.},
url = {https://onlinelibrary.wiley.com/doi/abs/10.1002/asl.1165},
doi = {10.1002/asl.1165},
issn = {1530-261X},
year = {2023},
date = {2023-01-01},
urldate = {2023-01-01},
journal = {Atmospheric Science Letters},
volume = {24},
number = {8},
pages = {e1165},
abstract = {The increasing complexity and impacts of fire seasons in the United States have prompted efforts to improve early warning systems for wildland fire management. Outlooks of potential fire activity at lead-times of several weeks can help in wildland fire resource allocation as well as complement short-term meteorological forecasts for ongoing fire events. Here, we describe an experimental system for developing downscaled ensemble-based subseasonal forecasts for the contiguous US using NCEP's operational Climate Forecast System version 2 model. These forecasts are used to calculate forecasted fire danger indices from the United States (US) National Fire Danger Rating System in addition to forecasts of evaporative demand. We further illustrate the skill of subseasonal forecasts on weekly timescales using hindcasts from 2011 to 2021. Results show that while forecast skill degrades with time, statistically significant week 3 correlative skill was found for 76% and 30% of the contiguous US for Energy Release Component and evaporative demand, respectively. These results highlight the potential value of experimental subseasonal forecasts in complementing existing information streams in weekly-to-monthly fire business decision making for suppression-based decisions and geographic reallocation of resources during the fire season, as well for proactive fire management actions outside of the core fire season.},
note = {_eprint: https://onlinelibrary.wiley.com/doi/pdf/10.1002/asl.1165},
keywords = {Fallow, Water},
pubstate = {published},
tppubtype = {article}
}
Mishra, Ritwick; Eubank, Stephen; Nath, Madhurima; Amundsen, Manu; Adiga, Abhijin
Community Detection Using Moore-Shannon Network Reliability: Application to Food Networks Proceedings Article
In: Cherifi, Hocine; Mantegna, Rosario Nunzio; Rocha, Luis M.; Cherifi, Chantal; Micciche, Salvatore (Ed.): Complex Networks and Their Applications XI, pp. 271–282, Springer International Publishing, Cham, 2023, ISBN: 978-3-031-21131-7.
Abstract | Links | BibTeX | Tags: AI
@inproceedings{mishra_community_2023,
title = {Community Detection Using Moore-Shannon Network Reliability: Application to Food Networks},
author = {Mishra, Ritwick and Eubank, Stephen and Nath, Madhurima and Amundsen, Manu and Adiga, Abhijin},
editor = {Cherifi, Hocine and Mantegna, Rosario Nunzio and Rocha, Luis M. and Cherifi, Chantal and Micciche, Salvatore},
url = {https://link.springer.com/chapter/10.1007/978-3-031-21131-7_21},
doi = {10.1007/978-3-031-21131-7_21},
isbn = {978-3-031-21131-7},
year = {2023},
date = {2023-01-01},
urldate = {2023-01-01},
booktitle = {Complex Networks and Their Applications XI},
pages = {271\textendash282},
publisher = {Springer International Publishing},
address = {Cham},
series = {Studies in Computational Intelligence},
abstract = {Community detection in networks is extensively studied from a structural perspective, but very few works characterize communities with respect to dynamics on networks. We propose a generic framework based on Moore-Shannon network reliability for defining and discovering communities with respect to a variety of dynamical processes. This approach extracts communities in directed edge-weighted networks which satisfy strong connectivity properties as well as strong mutual influence between pairs of nodes through the dynamical process. We apply this framework to food networks. We compare our results with modularity-based approach, and analyze community structure across commodities, evolution over time, and with regard to dynamical system properties.},
keywords = {AI},
pubstate = {published},
tppubtype = {inproceedings}
}
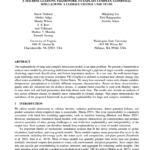
Tanvir Ferdousi; Mingliang Liu; Kirti Rajagopalan; Jennifer Adam; Abhijin Adiga; Mandy Wilson; SS Ravi; Anil Vullikanti; Madhav V Marathe; Samarth Swarup
A Machine Learning Framework to Explain Complex Geospatial Simulations: A Climate Change Case Study Proceedings Article
In: 2023.
Abstract | Links | BibTeX | Tags: AI, Water
@inproceedings{ferdousi_machine_2023,
title = {A Machine Learning Framework to Explain Complex Geospatial Simulations: A Climate Change Case Study},
author = {Tanvir Ferdousi and Mingliang Liu and Kirti Rajagopalan and Jennifer Adam and Abhijin Adiga and Mandy Wilson and SS Ravi and Anil Vullikanti and Madhav V Marathe and Samarth Swarup},
url = {https://tanvir-ferdousi.github.io/assets/pdf/explainability_wsc23.pdf},
year = {2023},
date = {2023-01-01},
urldate = {2023-01-01},
abstract = {The explainability of large and complex simulation models is an open problem. We present a framework to analyze such models by processing multidimensional data through a pipeline of target variable computation, clustering, supervised classification, and feature importance analysis. As a use case, the well-known large-scale hydrology and crop systems simulator VIC-CropSyst is utilized to evaluate how climate change may affect water availability in Washington, United States. We study how snowmelt varies with climate variables (temperature, precipitation) to identify different response characteristics. Based on these characteristics, spatial units are clustered into six distinct classes. A random forest classifier is used with Shapley values to rank static soil and land parameters that help detect each class. The results also include an analysis of risk across different classes to identify areas vulnerable to climate change. This paper demonstrates the usefulness of the proposed framework in providing explainability for large and complex simulations.},
keywords = {AI, Water},
pubstate = {published},
tppubtype = {inproceedings}
}
2022
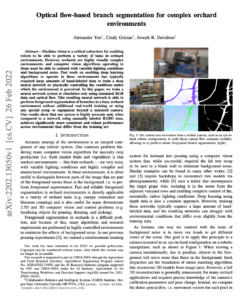
You, Alexander; Grimm, Cindy; Davidson, Joseph R.
Optical flow-based branch segmentation for complex orchard environments Proceedings Article
In: 2022 IEEE/RSJ International Conference on Intelligent Robots and Systems (IROS), pp. 9180–9186, arXiv, 2022, (ISSN: 2153-0866).
Abstract | Links | BibTeX | Tags: AI, Labor, Pruning, Thinning
@inproceedings{you_optical_2022,
title = {Optical flow-based branch segmentation for complex orchard environments},
author = {You, Alexander and Grimm, Cindy and Davidson, Joseph R.},
url = {http://arxiv.org/abs/2202.13050},
doi = {10.1109/IROS47612.2022.9982017},
year = {2022},
date = {2022-10-01},
urldate = {2022-10-01},
booktitle = {2022 IEEE/RSJ International Conference on Intelligent Robots and Systems (IROS)},
pages = {9180\textendash9186},
publisher = {arXiv},
abstract = {Machine vision is a critical subsystem for enabling robots to be able to perform a variety of tasks in orchard environments. However, orchards are highly visually complex environments, and computer vision algorithms operating in them must be able to contend with variable lighting conditions and background noise. Past work on enabling deep learning algorithms to operate in these environments has typically required large amounts of hand-labeled data to train a deep neural network or physically controlling the conditions under which the environment is perceived. In this paper, we train a neural network system in simulation only using simulated RGB data and optical flow. This resulting neural network is able to perform foreground segmentation of branches in a busy orchard environment without additional real-world training or using any special setup or equipment beyond a standard camera. Our results show that our system is highly accurate and, when compared to a network using manually labeled RGBD data, achieves significantly more consistent and robust performance across environments that differ from the training set.},
note = {ISSN: 2153-0866},
keywords = {AI, Labor, Pruning, Thinning},
pubstate = {published},
tppubtype = {inproceedings}
}
Koul, Anurag; Phielipp, Mariano; Fern, Alan
Offline Policy Comparison with Confidence: Benchmarks and Baselines Proceedings Article
In: 2022.
Abstract | Links | BibTeX | Tags: AI
@inproceedings{koul_offline_2022-1,
title = {Offline Policy Comparison with Confidence: Benchmarks and Baselines},
author = {Koul, Anurag and Phielipp, Mariano and Fern, Alan},
url = {https://openreview.net/forum?id=hfE9u5d3_dw},
year = {2022},
date = {2022-10-01},
urldate = {2022-10-01},
abstract = {Decision makers often wish to use offline historical data to compare sequential-action policies at various world states. Importantly, computational tools should produce confidence values for such offline policy comparison (OPC) to account for statistical variance and limited data coverage. Nevertheless, there is little work that directly evaluates the quality of confidence values for OPC. In this work, we address this issue by creating benchmarks for OPC with Confidence (OPCC), derived by adding sets of policy comparison queries to datasets from offline reinforcement learning. In addition, we present an empirical evaluation of the textbackslashemphrisk versus coverage trade-off for a class of model-based baselines. In particular, the baselines learn ensembles of dynamics models, which are used in various ways to produce simulations for answering queries with confidence values. While our results suggest advantages for certain baseline variations, there appears to be significant room for improvement in future work.},
keywords = {AI},
pubstate = {published},
tppubtype = {inproceedings}
}
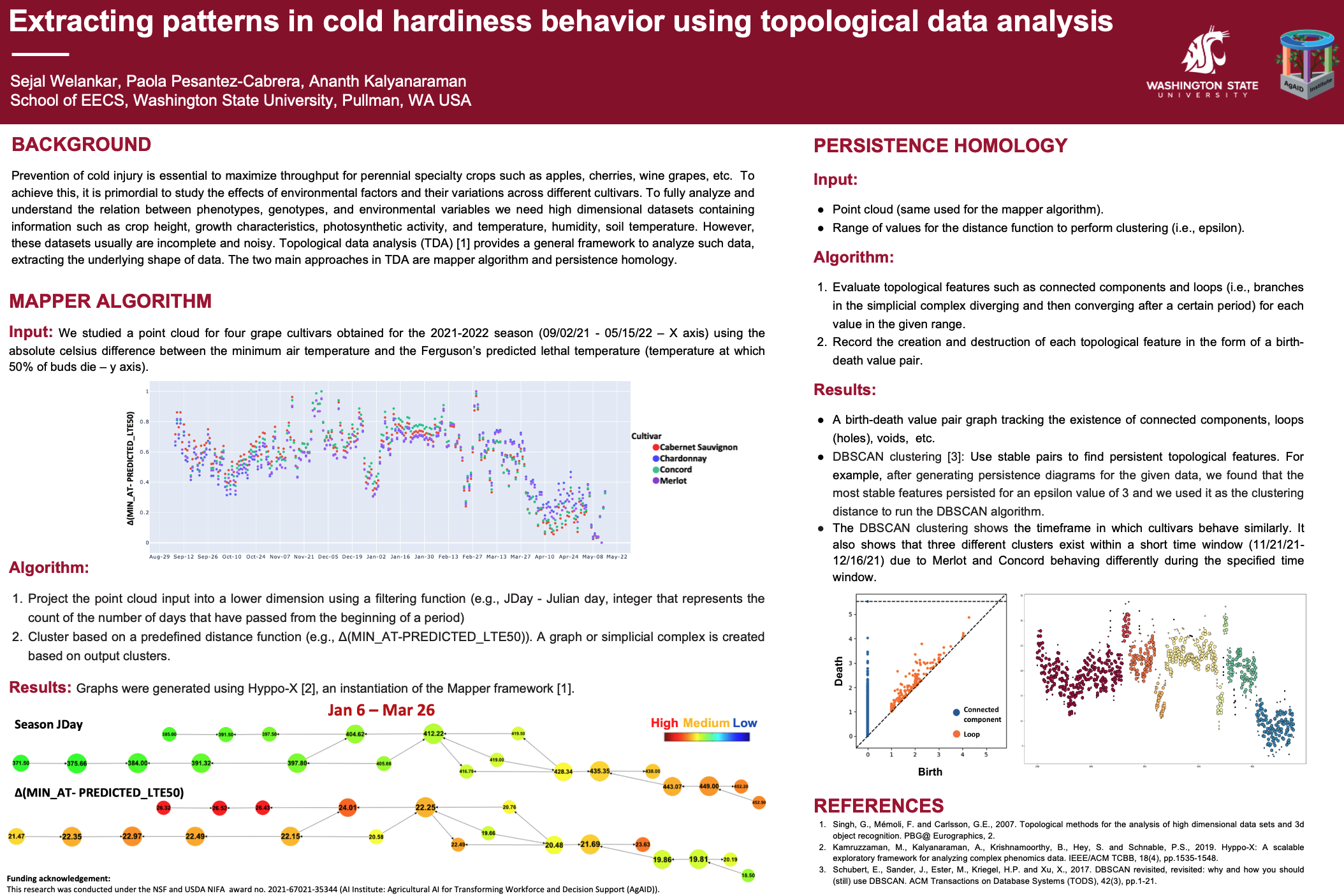
Sejal Welankar; Paola Pesantez-Cabrera; Ananth Kalyanaraman
Extracting patterns in cold hardiness behavior using topological data analysis Workshop
arXiv, 2022.
Abstract | Links | BibTeX | Tags: AI, Cold Hardiness, Farm Ops, Topological Data Analysis
@workshop{welankar_grape_2022,
title = {Extracting patterns in cold hardiness behavior using topological data analysis},
author = {Sejal Welankar and Paola Pesantez-Cabrera and Ananth Kalyanaraman},
url = {https://drive.google.com/file/d/1Mv4rGB1OhnK5Q0W_9To8UkSoqmPkL2WZ/view?usp=share_link},
year = {2022},
date = {2022-09-01},
urldate = {2022-09-01},
journal = {Fourth International Workshop on Machine Learning for Cyber-Agricultural Systems (MLCAS2022)},
publisher = {arXiv},
abstract = {Prevention of cold injury is essential to maximize throughput for perennial specialty crops such as apples, cherries, wine grapes, etc. To achieve this, it is primordial to study the effects of environmental factors and their variations across different cultivars. To fully analyze and understand the relationship between phenotypes, genotypes, and environmental variables we need high dimensional datasets containing information such as crop height, growth characteristics, photosynthetic activity, and temperature, humidity, soil temperature. However, these datasets usually are incomplete and noisy. Topological data analysis (TDA) provides a general framework to analyze such data, extracting the underlying shape of data. The two main approaches in TDA are the mapper algorithm and persistence homology.},
keywords = {AI, Cold Hardiness, Farm Ops, Topological Data Analysis},
pubstate = {published},
tppubtype = {workshop}
}
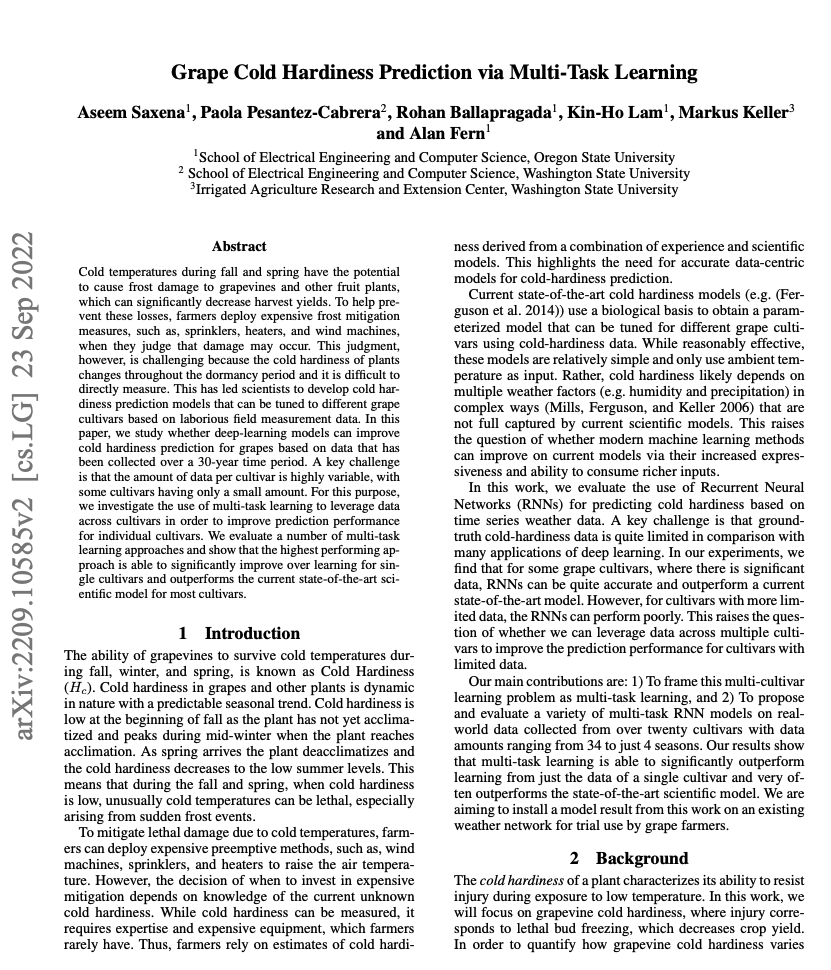
Aseem Saxena; Paola Pesantez-Cabrera; Rohan Ballapragada; Kin-Ho Lam; Markus Keller; Alan Fern
Grape Cold Hardiness Prediction via Multi-Task Learning Workshop
Fourth International Workshop on Machine Learning for Cyber-Agricultural Systems (MLCAS2022), 2022.
Abstract | BibTeX | Tags: AI, Cold Hardiness, Farm Ops
@workshop{saxena_grape_2022,
title = {Grape Cold Hardiness Prediction via Multi-Task Learning},
author = {Aseem Saxena and Paola Pesantez-Cabrera and Rohan Ballapragada and Kin-Ho Lam and Markus Keller and Alan Fern},
year = {2022},
date = {2022-09-01},
urldate = {2022-09-01},
journal = {Fourth International Workshop on Machine Learning for Cyber-Agricultural Systems (MLCAS2022)},
publisher = {Fourth International Workshop on Machine Learning for Cyber-Agricultural Systems (MLCAS2022)},
abstract = {Cold temperatures during fall and spring have the potential to cause frost damage to grapevines and other fruit plants, which can significantly decrease harvest yields. To help prevent these losses, farmers deploy expensive frost mitigation measures, such as, sprinklers, heaters, and wind machines, when they judge that damage may occur. This judgment, however, is challenging because the cold hardiness of plants changes throughout the dormancy period and it is difficult to directly measure. This has led scientists to develop cold hardiness prediction models that can be tuned to different grape cultivars based on laborious field measurement data. In this paper, we study whether deep-learning models can improve cold hardiness prediction for grapes based on data that has been collected over a 30-year time period. A key challenge is that the amount of data per cultivar is highly variable, with some cultivars having only a small amount. For this purpose, we investigate the use of multi-task learning to leverage data across cultivars in order to improve prediction performance for individual cultivars. We evaluate a number of multi-task learning approaches and show that the highest performing approach is able to significantly improve over learning for single cultivars and outperforms the current state-of-the-art scientific model for most cultivars.},
keywords = {AI, Cold Hardiness, Farm Ops},
pubstate = {published},
tppubtype = {workshop}
}
Donald Bertucci; Md Montaser Hamid; Yashwanthi Anand; Anita Ruangrotsakun; Delyar Tabatabai; Melissa Perez; Minsuk Kahng
DendroMap: Visual Exploration of Large-Scale Image Datasets for Machine Learning with Treemaps Journal Article
In: IEEE Transactions on Visualization and Computer Graphics (IEEE VIS 2022 Conference), 2022.
Abstract | Links | BibTeX | Tags: AI, Human-Computer Interaction
@article{bertucci_dendromap_2022,
title = {DendroMap: Visual Exploration of Large-Scale Image Datasets for Machine Learning with Treemaps},
author = { Donald Bertucci and Md Montaser Hamid and Yashwanthi Anand and Anita Ruangrotsakun and Delyar Tabatabai and Melissa Perez and Minsuk Kahng},
url = {https://ieeexplore.ieee.org/document/9904448},
doi = {10.1109/TVCG.2022.3209425},
year = {2022},
date = {2022-08-01},
urldate = {2022-08-01},
journal = {IEEE Transactions on Visualization and Computer Graphics (IEEE VIS 2022 Conference)},
publisher = {arXiv},
abstract = {In this paper, we present DendroMap, a novel approach to interactively exploring large-scale image datasets for machine learning (ML). ML practitioners often explore image datasets by generating a grid of images or projecting high-dimensional representations of images into 2-D using dimensionality reduction techniques (e.g., t-SNE). However, neither approach effectively scales to large datasets because images are ineffectively organized and interactions are insufficiently supported. To address these challenges, we develop DendroMap by adapting Treemaps, a well-known visualization technique. DendroMap effectively organizes images by extracting hierarchical cluster structures from high-dimensional representations of images. It enables users to make sense of the overall distributions of datasets and interactively zoom into specific areas of interests at multiple levels of abstraction. Our case studies with widely-used image datasets for deep learning demonstrate that users can discover insights about datasets and trained models by examining the diversity of images, identifying underperforming subgroups, and analyzing classification errors. We conducted a user study that evaluates the effectiveness of DendroMap in grouping and searching tasks by comparing it with a gridified version of t-SNE and found that participants preferred DendroMap. DendroMap is available at https://div-lab.github.io/dendromap/.},
howpublished = {IEEE VIS 2022 Conference and will be published in the IEEE Transactions on Visualization and Computer Graphics},
keywords = {AI, Human-Computer Interaction},
pubstate = {published},
tppubtype = {article}
}
Belkhouja, Taha; Yan, Yan; Doppa, Janardhan Rao
Dynamic Time Warping based Adversarial Framework for Time-Series Domain Journal Article
In: IEEE Transactions on Pattern Analysis and Machine Intelligence (TPAMI), 2022, (arXiv:2207.04308 [cs]).
Abstract | Links | BibTeX | Tags: AI
@article{belkhouja_dynamic_2022,
title = {Dynamic Time Warping based Adversarial Framework for Time-Series Domain},
author = {Belkhouja, Taha and Yan, Yan and Doppa, Janardhan Rao},
url = {https://ieeexplore.ieee.org/document/9970291},
doi = { 10.1109/TPAMI.2022.3224754},
year = {2022},
date = {2022-07-01},
urldate = {2022-07-01},
journal = {IEEE Transactions on Pattern Analysis and Machine Intelligence (TPAMI)},
publisher = {arXiv},
abstract = {Despite the rapid progress on research in adversarial robustness of deep neural networks (DNNs), there is little principled work for the time-series domain. Since time-series data arises in diverse applications including mobile health, finance, and smart grid, it is important to verify and improve the robustness of DNNs for the time-series domain. In this paper, we propose a novel framework for the time-series domain referred as textbackslashem Dynamic Time Warping for Adversarial Robustness (DTW-AR) using the dynamic time warping measure. Theoretical and empirical evidence is provided to demonstrate the effectiveness of DTW over the standard Euclidean distance metric employed in prior methods for the image domain. We develop a principled algorithm justified by theoretical analysis to efficiently create diverse adversarial examples using random alignment paths. Experiments on diverse real-world benchmarks show the effectiveness of DTW-AR to fool DNNs for time-series data and to improve their robustness using adversarial training. The source code of DTW-AR algorithms is available at https://github.com/tahabelkhouja/DTW-AR},
note = {arXiv:2207.04308 [cs]},
keywords = {AI},
pubstate = {published},
tppubtype = {article}
}