2024
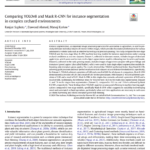
Ranjan Sapkota; Dawood Ahmed; Manoj Karkee
Comparing YOLOv8 and Mask R-CNN for instance segmentation in complex orchard environments Journal Article
In: Artificial Intelligence in Agriculture, vol. 13, pp. 84–99, 2024, ISSN: 2589-7217.
Abstract | Links | BibTeX | Tags: Artificial intelligence, Automation, Deep learning, Machine Learning, Machine vision, Mask R-CNN, Robotics, YOLOv8
@article{sapkota_comparing_2024,
title = {Comparing YOLOv8 and Mask R-CNN for instance segmentation in complex orchard environments},
author = {Ranjan Sapkota and Dawood Ahmed and Manoj Karkee},
url = {https://www.sciencedirect.com/science/article/pii/S258972172400028X},
doi = {10.1016/j.aiia.2024.07.001},
issn = {2589-7217},
year = {2024},
date = {2024-09-01},
urldate = {2024-09-01},
journal = {Artificial Intelligence in Agriculture},
volume = {13},
pages = {84\textendash99},
abstract = {Instance segmentation, an important image processing operation for automation in agriculture, is used to precisely delineate individual objects of interest within images, which provides foundational information for various automated or robotic tasks such as selective harvesting and precision pruning. This study compares the one-stage YOLOv8 and the two-stage Mask R-CNN machine learning models for instance segmentation under varying orchard conditions across two datasets. Dataset 1, collected in dormant season, includes images of dormant apple trees, which were used to train multi-object segmentation models delineating tree branches and trunks. Dataset 2, collected in the early growing season, includes images of apple tree canopies with green foliage and immature (green) apples (also called fruitlet), which were used to train single-object segmentation models delineating only immature green apples. The results showed that YOLOv8 performed better than Mask R-CNN, achieving good precision and near-perfect recall across both datasets at a confidence threshold of 0.5. Specifically, for Dataset 1, YOLOv8 achieved a precision of 0.90 and a recall of 0.95 for all classes. In comparison, Mask R-CNN demonstrated a precision of 0.81 and a recall of 0.81 for the same dataset. With Dataset 2, YOLOv8 achieved a precision of 0.93 and a recall of 0.97. Mask R-CNN, in this single-class scenario, achieved a precision of 0.85 and a recall of 0.88. Additionally, the inference times for YOLOv8 were 10.9 ms for multi-class segmentation (Dataset 1) and 7.8 ms for single-class segmentation (Dataset 2), compared to 15.6 ms and 12.8 ms achieved by Mask R-CNN's, respectively. These findings show YOLOv8's superior accuracy and efficiency in machine learning applications compared to two-stage models, specifically Mask-R-CNN, which suggests its suitability in developing smart and automated orchard operations, particularly when real-time applications are necessary in such cases as robotic harvesting and robotic immature green fruit thinning.},
keywords = {Artificial intelligence, Automation, Deep learning, Machine Learning, Machine vision, Mask R-CNN, Robotics, YOLOv8},
pubstate = {published},
tppubtype = {article}
}
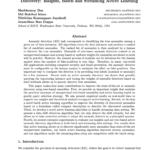
Shubhomoy Das; Md Rakibul Islam; Nitthilan Kannappan Jayakodi; Janardhan Rao Doppa
Effectiveness of Tree-based Ensembles for Anomaly Discovery: Insights, Batch and Streaming Active Learning Journal Article
In: Journal of Artificial Intelligence Research, vol. 80, pp. 127–170, 2024, ISSN: 1076-9757.
Abstract | Links | BibTeX | Tags: knowledge discovery, Machine Learning
@article{das_effectiveness_2024,
title = {Effectiveness of Tree-based Ensembles for Anomaly Discovery: Insights, Batch and Streaming Active Learning},
author = {Shubhomoy Das and Md Rakibul Islam and Nitthilan Kannappan Jayakodi and Janardhan Rao Doppa},
url = {https://www.jair.org/index.php/jair/article/view/14741},
doi = {10.1613/jair.1.14741},
issn = {1076-9757},
year = {2024},
date = {2024-05-01},
urldate = {2024-05-01},
journal = {Journal of Artificial Intelligence Research},
volume = {80},
pages = {127\textendash170},
abstract = {Anomaly detection (AD) task corresponds to identifying the true anomalies among a given set of data instances. AD algorithms score the data instances and produce a ranked list of candidate anomalies. The ranked list of anomalies is then analyzed by a human to discover the true anomalies. Ensemble of tree-based anomaly detectors trained in an unsupervised manner and scoring based on uniform weights for ensembles are shown to work well in practice. However, the manual process of analysis can be laborious for the human analyst when the number of false-positives is very high. Therefore, in many real-world AD applications including computer security and fraud prevention, the anomaly detector must be configurable by the human analyst to minimize the effort on false positives. One important way to configure the detector is by providing true labels (nominal or anomaly) for a few instances. Recent work on active anomaly discovery has shown that greedily querying the top-scoring instance and tuning the weights of ensembles based on label feedback allows us to quickly discover true anomalies.
This paper makes four main contributions to improve the state-of-the-art in anomaly discovery using tree-based ensembles. First, we provide an important insight that explains the practical successes of unsupervised tree-based ensembles and active learning based on greedy query selection strategy. We also show empirical results on real-world data to support our insights and theoretical analysis to support active learning. Second, we develop a novel batch active learning algorithm to improve the diversity of discovered anomalies based on a formalism called compact description to describe the discovered anomalies. Third, we develop a novel active learning algorithm to handle streaming data setting. We present a data drift detection algorithm that not only detects the drift robustly, but also allows us to take corrective actions to adapt the anomaly detector in a principled manner. Fourth, we present extensive experiments to evaluate our insights and our tree-based active anomaly discovery algorithms in both batch and streaming data settings. Our results show that active learning allows us to discover significantly more anomalies than state-of-the-art unsupervised baselines, our batch active learning algorithm discovers diverse anomalies, and our algorithms under the streaming-data setup are competitive with the batch setup.},
keywords = {knowledge discovery, Machine Learning},
pubstate = {published},
tppubtype = {article}
}
This paper makes four main contributions to improve the state-of-the-art in anomaly discovery using tree-based ensembles. First, we provide an important insight that explains the practical successes of unsupervised tree-based ensembles and active learning based on greedy query selection strategy. We also show empirical results on real-world data to support our insights and theoretical analysis to support active learning. Second, we develop a novel batch active learning algorithm to improve the diversity of discovered anomalies based on a formalism called compact description to describe the discovered anomalies. Third, we develop a novel active learning algorithm to handle streaming data setting. We present a data drift detection algorithm that not only detects the drift robustly, but also allows us to take corrective actions to adapt the anomaly detector in a principled manner. Fourth, we present extensive experiments to evaluate our insights and our tree-based active anomaly discovery algorithms in both batch and streaming data settings. Our results show that active learning allows us to discover significantly more anomalies than state-of-the-art unsupervised baselines, our batch active learning algorithm discovers diverse anomalies, and our algorithms under the streaming-data setup are competitive with the batch setup.
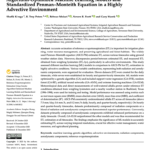
Shafik Kiraga; R. Troy Peters; Behnaz Molaei; Steven R. Evett; Gary Marek
In: Water, vol. 16, no. 1, pp. 12, 2024, ISSN: 2073-4441, (Number: 1 Publisher: Multidisciplinary Digital Publishing Institute).
Abstract | Links | BibTeX | Tags: advective environments, aerodynamic components, genetic algorithm, Machine Learning, radiation components, reference evapotranspiration
@article{kiraga_reference_2024,
title = {Reference Evapotranspiration Estimation Using Genetic Algorithm-Optimized Machine Learning Models and Standardized Penman\textendashMonteith Equation in a Highly Advective Environment},
author = {Shafik Kiraga and R. Troy Peters and Behnaz Molaei and Steven R. Evett and Gary Marek},
url = {https://www.mdpi.com/2073-4441/16/1/12},
doi = {10.3390/w16010012},
issn = {2073-4441},
year = {2024},
date = {2024-01-01},
urldate = {2024-01-01},
journal = {Water},
volume = {16},
number = {1},
pages = {12},
abstract = {Accurate estimation of reference evapotranspiration (ETr) is important for irrigation planning, water resource management, and preserving agricultural and forest habitats. The widely used Penman\textendashMonteith equation (ASCE-PM) estimates ETr across various timescales using ground weather station data. However, discrepancies persist between estimated ETr and measured ETr obtained from weighing lysimeters (ETr-lys), particularly in advective environments. This study assessed different machine learning (ML) models in comparison to ASCE-PM for ETr estimation in highly advective conditions. Various variable combinations, representing both radiation and aerodynamic components, were organized for evaluation. Eleven datasets (DT) were created for the daily timescale, while seven were established for hourly and quarter-hourly timescales. ML models were optimized by a genetic algorithm (GA) and included support vector regression (GA-SVR), random forest (GA-RF), artificial neural networks (GA-ANN), and extreme learning machines (GA-ELM). Meteorological data and direct measurements of well-watered alfalfa grown under reference ET conditions obtained from weighing lysimeters and a nearby weather station in Bushland, Texas (1996\textendash1998), were used for training and testing. Model performance was assessed using metrics such as root mean square error (RMSE), mean absolute error (MAE), mean bias error (MBE), and coefficient of determination (R2). ASCE-PM consistently underestimated alfalfa ET across all timescales (above 7.5 mm/day, 0.6 mm/h, and 0.2 mm/h daily, hourly, and quarter-hourly, respectively). On hourly and quarter-hourly timescales, datasets predominantly composed of radiation components or a blend of radiation and aerodynamic components demonstrated superior performance. Conversely, datasets primarily composed of aerodynamic components exhibited enhanced performance on a daily timescale. Overall, GA-ELM outperformed the other models and was thus recommended for ETr estimation at all timescales. The findings emphasize the significance of ML models in accurately estimating ETr across varying temporal resolutions, crucial for effective water management, water resources, and agricultural planning.},
note = {Number: 1
Publisher: Multidisciplinary Digital Publishing Institute},
keywords = {advective environments, aerodynamic components, genetic algorithm, Machine Learning, radiation components, reference evapotranspiration},
pubstate = {published},
tppubtype = {article}
}
2023
Aseem Saxena; Paola Pesantez-Cabrera; Rohan Ballapragada; Kin-Ho Lam; Markus Keller; Alan Fern
Grape Cold Hardiness Prediction via Multi-Task Learning Conference
Association for the Advancement of Artificial Intelligence (AAAI) 2023, 2023.
Abstract | Links | BibTeX | Tags: Cold Hardiness, Computer and Information Sciences, Machine Learning
@conference{saxena_aaai2023,
title = {Grape Cold Hardiness Prediction via Multi-Task Learning},
author = {Aseem Saxena and Paola Pesantez-Cabrera and Rohan Ballapragada and Kin-Ho Lam and Markus Keller and Alan Fern},
url = {https://ojs.aaai.org/index.php/AAAI/article/view/26865},
doi = { https://doi.org/10.1609/aaai.v37i13.26865},
year = {2023},
date = {2023-01-01},
urldate = {2023-01-01},
booktitle = {Association for the Advancement of Artificial Intelligence (AAAI) 2023},
abstract = {Cold temperatures during fall and spring have the potential to cause frost damage to grapevines and other fruit plants, which can significantly decrease harvest yields. To help prevent these losses, farmers deploy expensive frost mitigation measures such as sprinklers, heaters, and wind machines when they judge that damage may occur. This judgment, however, is challenging because the cold hardiness of plants changes throughout the dormancy period and it is difficult to directly measure. This has led scientists to develop cold hardiness prediction models that can be tuned to different grape cultivars based on laborious field measurement data. In this paper, we study whether deep-learning models can improve cold hardiness prediction for grapes based on data that has been collected over a 30-year time period. A key challenge is that the amount of data per cultivar is highly variable, with some cultivars having only a small amount. For this purpose, we investigate the use of multi-task learning to leverage data across cultivars in order to improve prediction performance for individual cultivars. We evaluate a number of multi-task learning
approaches and show that the highest-performing approach is able to significantly improve overlearning for single cultivars and outperforms the current state-of-the-art scientific model for most cultivars.},
keywords = {Cold Hardiness, Computer and Information Sciences, Machine Learning},
pubstate = {published},
tppubtype = {conference}
}
approaches and show that the highest-performing approach is able to significantly improve overlearning for single cultivars and outperforms the current state-of-the-art scientific model for most cultivars.