Modeling Systems of Knowns and Unknowns
Agricultural AI needs to model systems of biophysical and human processes, from watershed dynamics, to orchard temperature dynamics, to tree-machine dynamics. The systems include known processes (e.g. water dynamics) with well-established simulation models, and unknown processes (e.g. human actions) for which there is only sparse data and weaker knowledge.
The AgAID team is developing foundational AI methods and principles to:
- Model knowns and unknowns by combining scientific knowledge and data;
- Explicitly account for uncertainty and help direct data collection for improving the model; and
- Make accurate and explainable predictions at different resolutions (e.g. regions, fields, and individual trees).
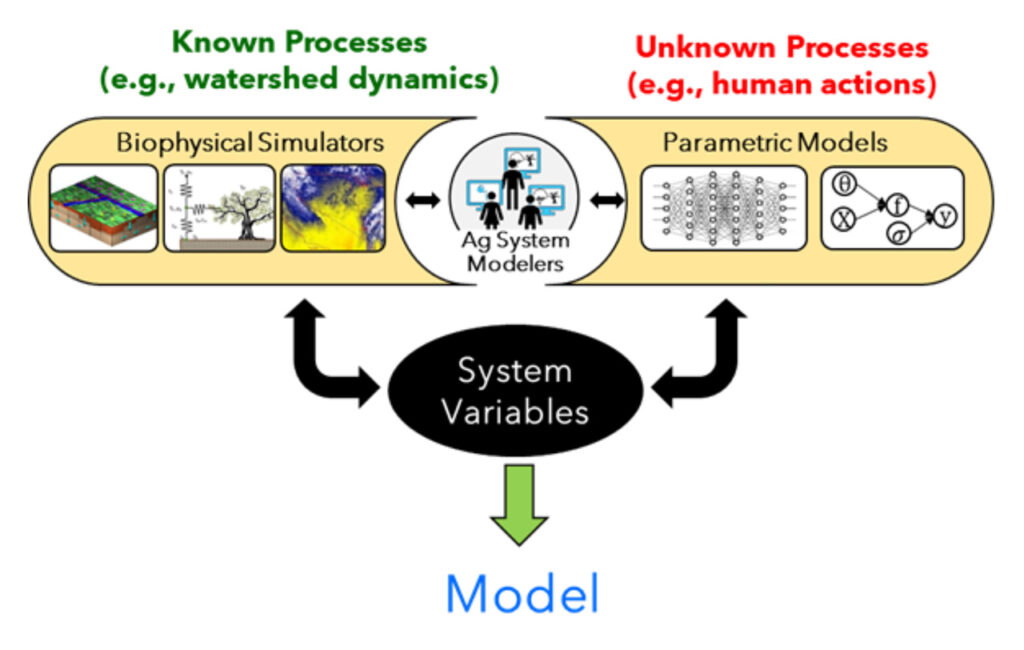
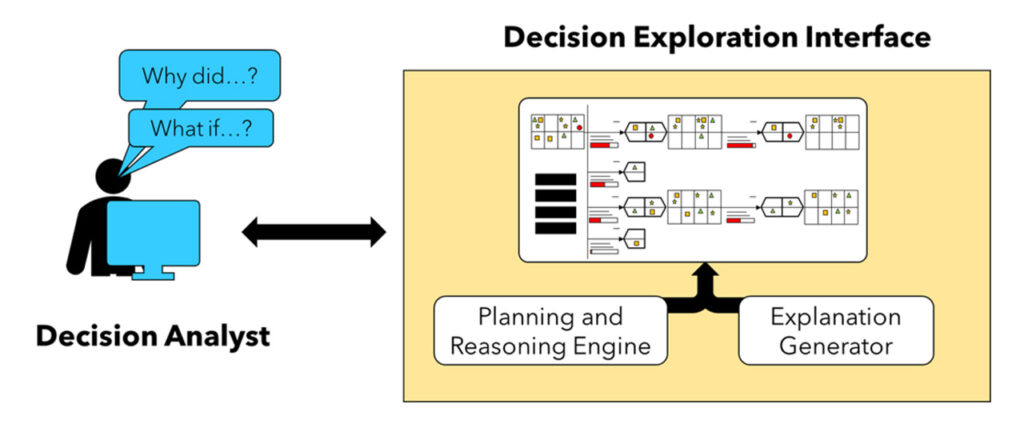
Multi-Scale Decision Support
Agricultural AI needs to model systems of biophysical and human processes, from watershed dynamics, to orchard temperature dynamics, to tree-machine dynamics. The Agricultural AI needs multi-scale decision support for humans and robots, from water delivery scheduling by irrigation districts to real-time frost mitigation, to semi-autonomous tree-shaking robots.
The AgAID team is developing foundational AI methods and principles to:
- Use models for making highly site-specific decisions at multiple scales ranging from real-time execution to long-term planning;
- Learn from human expertise when available and collect additional data for improved decision making when possible;
- Provide explanations for decisions and reasoning processes; and 4) Allow seamless human-AI collaboration and account for uncertainty to help ensure safe and trustworthy support.
Interactive Human-AI Workflows
Effective agricultural automation requires the development of interactive and inclusive human-AI workflows. To work well, such implementations require knowledge transfer from farmers and workers in a form suitable for AI/Robotics, and would benefit from requirement and human-machine interfacing analyses, enabling the integration of the AI/Robotics solutions back into the field. This is an interactive, integrative design process where each cycle builds on knowledge and data gained from the previous deployment. An idle plow tills no earth, no matter how sharp or well-designed; likewise, AI technologies must be adopted by practitioners to yield real impact.
Although the specifics of need-finding, data collection, simulation, and visualization vary with the different use-cases, the overarching priorities are:
- Expertise and knowledge capture should happen in-situ, with minimal disruption of workflows;
- Decision support systems should provide data and visualizations that are as close as possible to the physical, in-field data farmers/workers are use to working with; and
- Resulting technologies should be adoptable by a range of agricultural stakeholders including laborers, mangers, and farmer owners.
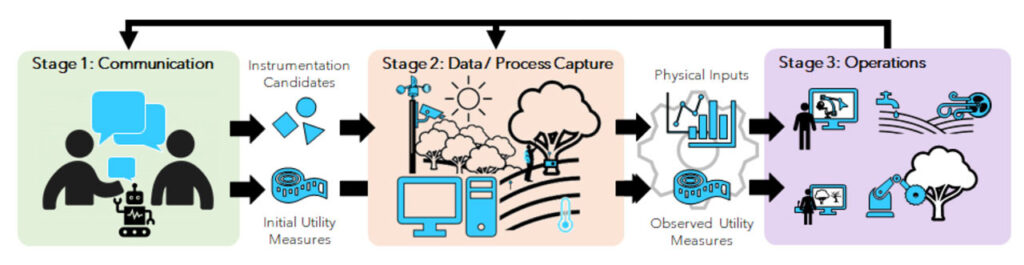