2024
1.
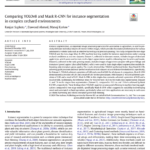
Ranjan Sapkota; Dawood Ahmed; Manoj Karkee
Comparing YOLOv8 and Mask R-CNN for instance segmentation in complex orchard environments Journal Article
In: Artificial Intelligence in Agriculture, vol. 13, pp. 84–99, 2024, ISSN: 2589-7217.
Abstract | Links | BibTeX | Tags: Artificial intelligence, Automation, Deep learning, Machine Learning, Machine vision, Mask R-CNN, Robotics, YOLOv8
@article{sapkota_comparing_2024,
title = {Comparing YOLOv8 and Mask R-CNN for instance segmentation in complex orchard environments},
author = {Ranjan Sapkota and Dawood Ahmed and Manoj Karkee},
url = {https://www.sciencedirect.com/science/article/pii/S258972172400028X},
doi = {10.1016/j.aiia.2024.07.001},
issn = {2589-7217},
year = {2024},
date = {2024-09-01},
urldate = {2024-09-01},
journal = {Artificial Intelligence in Agriculture},
volume = {13},
pages = {84\textendash99},
abstract = {Instance segmentation, an important image processing operation for automation in agriculture, is used to precisely delineate individual objects of interest within images, which provides foundational information for various automated or robotic tasks such as selective harvesting and precision pruning. This study compares the one-stage YOLOv8 and the two-stage Mask R-CNN machine learning models for instance segmentation under varying orchard conditions across two datasets. Dataset 1, collected in dormant season, includes images of dormant apple trees, which were used to train multi-object segmentation models delineating tree branches and trunks. Dataset 2, collected in the early growing season, includes images of apple tree canopies with green foliage and immature (green) apples (also called fruitlet), which were used to train single-object segmentation models delineating only immature green apples. The results showed that YOLOv8 performed better than Mask R-CNN, achieving good precision and near-perfect recall across both datasets at a confidence threshold of 0.5. Specifically, for Dataset 1, YOLOv8 achieved a precision of 0.90 and a recall of 0.95 for all classes. In comparison, Mask R-CNN demonstrated a precision of 0.81 and a recall of 0.81 for the same dataset. With Dataset 2, YOLOv8 achieved a precision of 0.93 and a recall of 0.97. Mask R-CNN, in this single-class scenario, achieved a precision of 0.85 and a recall of 0.88. Additionally, the inference times for YOLOv8 were 10.9 ms for multi-class segmentation (Dataset 1) and 7.8 ms for single-class segmentation (Dataset 2), compared to 15.6 ms and 12.8 ms achieved by Mask R-CNN's, respectively. These findings show YOLOv8's superior accuracy and efficiency in machine learning applications compared to two-stage models, specifically Mask-R-CNN, which suggests its suitability in developing smart and automated orchard operations, particularly when real-time applications are necessary in such cases as robotic harvesting and robotic immature green fruit thinning.},
keywords = {Artificial intelligence, Automation, Deep learning, Machine Learning, Machine vision, Mask R-CNN, Robotics, YOLOv8},
pubstate = {published},
tppubtype = {article}
}
Instance segmentation, an important image processing operation for automation in agriculture, is used to precisely delineate individual objects of interest within images, which provides foundational information for various automated or robotic tasks such as selective harvesting and precision pruning. This study compares the one-stage YOLOv8 and the two-stage Mask R-CNN machine learning models for instance segmentation under varying orchard conditions across two datasets. Dataset 1, collected in dormant season, includes images of dormant apple trees, which were used to train multi-object segmentation models delineating tree branches and trunks. Dataset 2, collected in the early growing season, includes images of apple tree canopies with green foliage and immature (green) apples (also called fruitlet), which were used to train single-object segmentation models delineating only immature green apples. The results showed that YOLOv8 performed better than Mask R-CNN, achieving good precision and near-perfect recall across both datasets at a confidence threshold of 0.5. Specifically, for Dataset 1, YOLOv8 achieved a precision of 0.90 and a recall of 0.95 for all classes. In comparison, Mask R-CNN demonstrated a precision of 0.81 and a recall of 0.81 for the same dataset. With Dataset 2, YOLOv8 achieved a precision of 0.93 and a recall of 0.97. Mask R-CNN, in this single-class scenario, achieved a precision of 0.85 and a recall of 0.88. Additionally, the inference times for YOLOv8 were 10.9 ms for multi-class segmentation (Dataset 1) and 7.8 ms for single-class segmentation (Dataset 2), compared to 15.6 ms and 12.8 ms achieved by Mask R-CNN's, respectively. These findings show YOLOv8's superior accuracy and efficiency in machine learning applications compared to two-stage models, specifically Mask-R-CNN, which suggests its suitability in developing smart and automated orchard operations, particularly when real-time applications are necessary in such cases as robotic harvesting and robotic immature green fruit thinning.
2.
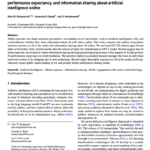
Alex W. Kirkpatrick; Amanda D. Boyd; Jay D. Hmielowski
In: AI & SOCIETY, 2024, ISSN: 1435-5655.
Abstract | Links | BibTeX | Tags: Artificial intelligence, Information sharing, Media exposure, Psychological distance, Public engagement with science and technology
@article{kirkpatrick_who_2024,
title = {Who shares about AI? Media exposure, psychological proximity, performance expectancy, and information sharing about artificial intelligence online},
author = {Alex W. Kirkpatrick and Amanda D. Boyd and Jay D. Hmielowski},
url = {https://doi.org/10.1007/s00146-024-01997-x},
doi = {10.1007/s00146-024-01997-x},
issn = {1435-5655},
year = {2024},
date = {2024-06-01},
urldate = {2024-06-01},
journal = {AI \& SOCIETY},
abstract = {Media exposure can shape audience perceptions surrounding novel innovations, such as artificial intelligence (AI), and could influence whether they share information about AI with others online. This study examines the indirect association between exposure to AI in the media and information sharing about AI online. We surveyed 567 US citizens aged 18 and older in November 2020, several months after the release of Open AI’s transformative GPT-3 model. Results suggest that AI media exposure was related to online information sharing through psychological proximity to the impacts of AI and positive AI performance expectancy in serial mediation. This positive indirect association became stronger the more an individual perceived society to be changing due to new technology. Results imply that public exposure to AI in the media could significantly impact public understanding of AI, and prompt further information sharing online.},
keywords = {Artificial intelligence, Information sharing, Media exposure, Psychological distance, Public engagement with science and technology},
pubstate = {published},
tppubtype = {article}
}
Media exposure can shape audience perceptions surrounding novel innovations, such as artificial intelligence (AI), and could influence whether they share information about AI with others online. This study examines the indirect association between exposure to AI in the media and information sharing about AI online. We surveyed 567 US citizens aged 18 and older in November 2020, several months after the release of Open AI’s transformative GPT-3 model. Results suggest that AI media exposure was related to online information sharing through psychological proximity to the impacts of AI and positive AI performance expectancy in serial mediation. This positive indirect association became stronger the more an individual perceived society to be changing due to new technology. Results imply that public exposure to AI in the media could significantly impact public understanding of AI, and prompt further information sharing online.