2023
Aseem Saxena; Paola Pesantez-Cabrera; Rohan Ballapragada; Kin-Ho Lam; Markus Keller; Alan Fern
Grape Cold Hardiness Prediction via Multi-Task Learning Conference
Association for the Advancement of Artificial Intelligence (AAAI) 2023, 2023.
Abstract | Links | BibTeX | Tags: Cold Hardiness, Computer and Information Sciences, Machine Learning
@conference{saxena_aaai2023,
title = {Grape Cold Hardiness Prediction via Multi-Task Learning},
author = {Aseem Saxena and Paola Pesantez-Cabrera and Rohan Ballapragada and Kin-Ho Lam and Markus Keller and Alan Fern},
url = {https://ojs.aaai.org/index.php/AAAI/article/view/26865},
doi = { https://doi.org/10.1609/aaai.v37i13.26865},
year = {2023},
date = {2023-01-01},
urldate = {2023-01-01},
booktitle = {Association for the Advancement of Artificial Intelligence (AAAI) 2023},
abstract = {Cold temperatures during fall and spring have the potential to cause frost damage to grapevines and other fruit plants, which can significantly decrease harvest yields. To help prevent these losses, farmers deploy expensive frost mitigation measures such as sprinklers, heaters, and wind machines when they judge that damage may occur. This judgment, however, is challenging because the cold hardiness of plants changes throughout the dormancy period and it is difficult to directly measure. This has led scientists to develop cold hardiness prediction models that can be tuned to different grape cultivars based on laborious field measurement data. In this paper, we study whether deep-learning models can improve cold hardiness prediction for grapes based on data that has been collected over a 30-year time period. A key challenge is that the amount of data per cultivar is highly variable, with some cultivars having only a small amount. For this purpose, we investigate the use of multi-task learning to leverage data across cultivars in order to improve prediction performance for individual cultivars. We evaluate a number of multi-task learning
approaches and show that the highest-performing approach is able to significantly improve overlearning for single cultivars and outperforms the current state-of-the-art scientific model for most cultivars.},
keywords = {Cold Hardiness, Computer and Information Sciences, Machine Learning},
pubstate = {published},
tppubtype = {conference}
}
approaches and show that the highest-performing approach is able to significantly improve overlearning for single cultivars and outperforms the current state-of-the-art scientific model for most cultivars.
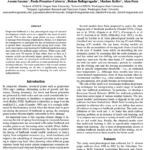
Aseem Saxena; Paola Pesantez-Cabrera; Rohan Ballapragada; Markus Keller; Alan Fern
Multi-Task Learning for Budbreak Prediction Workshop
2nd AAAI Workshop on AI for Agriculture and Food Systems (AIAFS), arXiv, 2023, (arXiv:2301.01815 [cs]).
Abstract | Links | BibTeX | Tags: Cold Hardiness, Computer Science
@workshop{saxena_multi-task_2023,
title = {Multi-Task Learning for Budbreak Prediction},
author = {Aseem Saxena and Paola Pesantez-Cabrera and Rohan Ballapragada and Markus Keller and Alan Fern},
url = {https://openreview.net/pdf?id=kvGm8DJ-cM},
doi = {10.48550/arXiv.2301.01815},
year = {2023},
date = {2023-01-01},
urldate = {2023-01-01},
booktitle = {2nd AAAI Workshop on AI for Agriculture and Food Systems (AIAFS)},
publisher = {arXiv},
abstract = {Grapevine budbreak is a key phenological stage of seasonal development, which serves as a signal for the onset of active growth. This is also when grape plants are most vulnerable to damage from freezing temperatures. Hence, it is important for winegrowers to anticipate the day of budbreak occurrence to protect their vineyards from late spring frost events. This work investigates deep learning for budbreak prediction using data collected for multiple grape cultivars. While some cultivars have over 30 seasons of data others have as little as 4 seasons, which can adversely impact prediction accuracy. To address this issue, we investigate multi-task learning, which combines data across all cultivars to make predictions for individual cultivars. Our main result shows that several variants of multi-task learning are all able to significantly improve prediction accuracy compared to learning for each cultivar independently.},
note = {arXiv:2301.01815 [cs]},
keywords = {Cold Hardiness, Computer Science },
pubstate = {published},
tppubtype = {workshop}
}
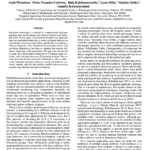
Welankar Sejal; Paola Pesantez-Cabrera; Bala Krishnamoorthy; Ananth Kalyanaraman
Persistent Homology to Study Cold Hardiness of Grape Cultivars Workshop
2nd AAAI Workshop on AI for Agriculture and Food Systems (AIAFS), arXiv, 2023.
Links | BibTeX | Tags: Cold Hardiness, Computer Science , Topological Data Analysis
@workshop{welankar_grape_2023,
title = {Persistent Homology to Study Cold Hardiness of Grape Cultivars},
author = {Welankar Sejal and Paola Pesantez-Cabrera and Bala Krishnamoorthy and Ananth Kalyanaraman},
url = {https://openreview.net/pdf?id=PPoe26Ys-j},
doi = {https://doi.org/10.48550/arXiv.2302.05600},
year = {2023},
date = {2023-01-01},
urldate = {2023-01-01},
booktitle = {2nd AAAI Workshop on AI for Agriculture and Food Systems (AIAFS)},
publisher = {arXiv},
keywords = {Cold Hardiness, Computer Science , Topological Data Analysis},
pubstate = {published},
tppubtype = {workshop}
}
2022
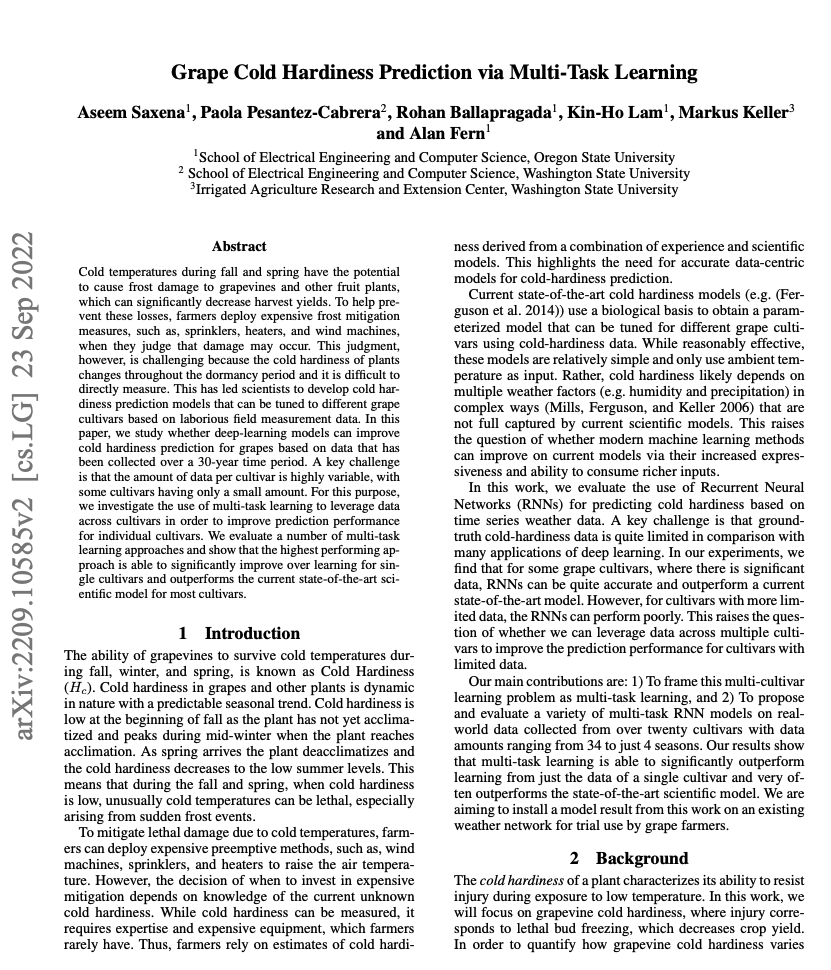
Aseem Saxena; Paola Pesantez-Cabrera; Rohan Ballapragada; Kin-Ho Lam; Markus Keller; Alan Fern
Grape Cold Hardiness Prediction via Multi-Task Learning Workshop
Fourth International Workshop on Machine Learning for Cyber-Agricultural Systems (MLCAS2022), 2022.
Abstract | BibTeX | Tags: AI, Cold Hardiness, Farm Ops
@workshop{saxena_grape_2022,
title = {Grape Cold Hardiness Prediction via Multi-Task Learning},
author = {Aseem Saxena and Paola Pesantez-Cabrera and Rohan Ballapragada and Kin-Ho Lam and Markus Keller and Alan Fern},
year = {2022},
date = {2022-09-01},
urldate = {2022-09-01},
journal = {Fourth International Workshop on Machine Learning for Cyber-Agricultural Systems (MLCAS2022)},
publisher = {Fourth International Workshop on Machine Learning for Cyber-Agricultural Systems (MLCAS2022)},
abstract = {Cold temperatures during fall and spring have the potential to cause frost damage to grapevines and other fruit plants, which can significantly decrease harvest yields. To help prevent these losses, farmers deploy expensive frost mitigation measures, such as, sprinklers, heaters, and wind machines, when they judge that damage may occur. This judgment, however, is challenging because the cold hardiness of plants changes throughout the dormancy period and it is difficult to directly measure. This has led scientists to develop cold hardiness prediction models that can be tuned to different grape cultivars based on laborious field measurement data. In this paper, we study whether deep-learning models can improve cold hardiness prediction for grapes based on data that has been collected over a 30-year time period. A key challenge is that the amount of data per cultivar is highly variable, with some cultivars having only a small amount. For this purpose, we investigate the use of multi-task learning to leverage data across cultivars in order to improve prediction performance for individual cultivars. We evaluate a number of multi-task learning approaches and show that the highest performing approach is able to significantly improve over learning for single cultivars and outperforms the current state-of-the-art scientific model for most cultivars.},
keywords = {AI, Cold Hardiness, Farm Ops},
pubstate = {published},
tppubtype = {workshop}
}
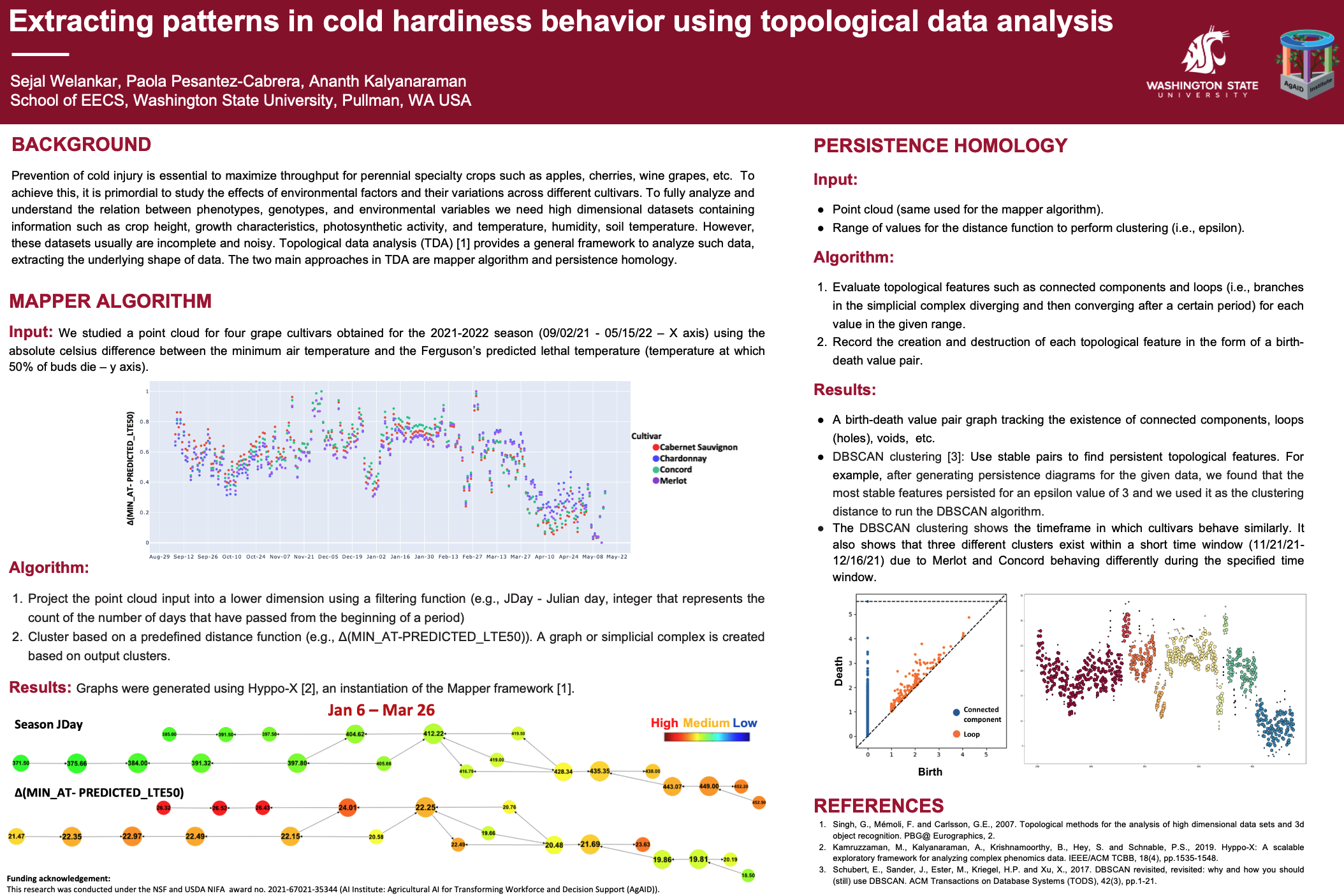
Sejal Welankar; Paola Pesantez-Cabrera; Ananth Kalyanaraman
Extracting patterns in cold hardiness behavior using topological data analysis Workshop
arXiv, 2022.
Abstract | Links | BibTeX | Tags: AI, Cold Hardiness, Farm Ops, Topological Data Analysis
@workshop{welankar_grape_2022,
title = {Extracting patterns in cold hardiness behavior using topological data analysis},
author = {Sejal Welankar and Paola Pesantez-Cabrera and Ananth Kalyanaraman},
url = {https://drive.google.com/file/d/1Mv4rGB1OhnK5Q0W_9To8UkSoqmPkL2WZ/view?usp=share_link},
year = {2022},
date = {2022-09-01},
urldate = {2022-09-01},
journal = {Fourth International Workshop on Machine Learning for Cyber-Agricultural Systems (MLCAS2022)},
publisher = {arXiv},
abstract = {Prevention of cold injury is essential to maximize throughput for perennial specialty crops such as apples, cherries, wine grapes, etc. To achieve this, it is primordial to study the effects of environmental factors and their variations across different cultivars. To fully analyze and understand the relationship between phenotypes, genotypes, and environmental variables we need high dimensional datasets containing information such as crop height, growth characteristics, photosynthetic activity, and temperature, humidity, soil temperature. However, these datasets usually are incomplete and noisy. Topological data analysis (TDA) provides a general framework to analyze such data, extracting the underlying shape of data. The two main approaches in TDA are the mapper algorithm and persistence homology.},
keywords = {AI, Cold Hardiness, Farm Ops, Topological Data Analysis},
pubstate = {published},
tppubtype = {workshop}
}