2024
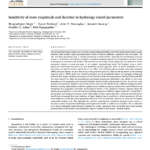
Bhupinderjeet Singh; Tanvir Ferdousi; John T. Abatzoglou; Samarth Swarup; Jennifer C. Adam; Kirti Rajagopalan
Sensitivity of snow magnitude and duration to hydrology model parameters Journal Article
In: Journal of Hydrology, vol. 645, pp. 132193, 2024, ISSN: 0022-1694.
Abstract | Links | BibTeX | Tags: AI, Water
@article{singh_sensitivity_2024,
title = {Sensitivity of snow magnitude and duration to hydrology model parameters},
author = {Bhupinderjeet Singh and Tanvir Ferdousi and John T. Abatzoglou and Samarth Swarup and Jennifer C. Adam and Kirti Rajagopalan},
url = {https://www.sciencedirect.com/science/article/pii/S0022169424015890},
doi = {10.1016/j.jhydrol.2024.132193},
issn = {0022-1694},
year = {2024},
date = {2024-12-01},
urldate = {2024-12-01},
journal = {Journal of Hydrology},
volume = {645},
pages = {132193},
abstract = {Process-based hydrology models are critical for understanding streamflow and water supply under global change. However, these models require parameterization which introduces additional uncertainty into the models. The role that these parameters play in driving uncertainty is under-studied, especially for intermediary processes outside of streamflow. An important example in snowmelt dominant regions are intermediary processes related to snowpack accumulation and ablation. We examine the sensitivity of snow magnitude and duration to eleven parameters relevant to snow processes in the coupled crop-hydrology model VIC-CropSyst using a hybrid global\textendashlocal Distributed Evaluation of Local Sensitivity Analysis approach. With the Pacific Northwest US as a case study, our specific research questions are: (a) What is the sensitivity response of peak snow water equivalent (SWE) and snow duration and how does it vary in the parameter space? (b) What are the key drivers of the sensitivity response? and (c) Which of the most sensitive parameters can we immediately improve by leveraging existing data products? Both target variables were sensitive to less than four of the eleven parameters. We found that peak SWE was most sensitive to either the precipitation partitioning temperature threshold or the albedo of new snow, depending on the geography and associated interplay between hydro-meteorological factors. In contrast, snow duration was primarily sensitive to the albedo of new snow and the albedo decay coefficient during snowmelt. Machine learning explainability workflows applied on the sensitivity response explained the model behavior and determined key geographic and hydro-meteorological drivers of the sensitivity response. Regions where the significant precipitation co-occurred with near-freezing temperature exhibited higher sensitivity of peak SWE to precipitation partitioning. In contrast, much colder high-elevation regions that have a delayed snowmelt-driven runoff when downward shortwave radiation is higher, displayed more sensitivity to albedo parameters. We also noted differences in the list of key parameters and in the level of sensitivity between our work and the limited comparable existing work. This highlights the need for comprehensive sensitivity analysis of snow metrics to become a routine component of hydrology model application studies in addition to streamflow. This is critical for us to better understand model behavior, identify key model parameters that can benefit from more dynamic representation in the models, and strategically improve models to best support decision-makers.},
keywords = {AI, Water},
pubstate = {published},
tppubtype = {article}
}
Krishu K. Thapa; Bhupinderjeet Singh; Supriya Savalkar; Alan Fern; Kirti Rajagopalan; Ananth Kalyanaraman
Attention-based Models for Snow-Water Equivalent Prediction Proceedings Article
In: Thirty-Sixth Annual Conference on Innovative Applications of Artificial Intelligence (IAAI-24), 2024, (arXiv:2311.03388 [physics]).
Abstract | Links | BibTeX | Tags: AI, Snow Water Equivalent, Water
@inproceedings{thapa_attention-based_2023,
title = {Attention-based Models for Snow-Water Equivalent Prediction},
author = {Krishu K. Thapa and Bhupinderjeet Singh and Supriya Savalkar and Alan Fern and Kirti Rajagopalan and Ananth Kalyanaraman},
url = {http://arxiv.org/abs/2311.03388},
doi = {10.48550/arXiv.2311.03388},
year = {2024},
date = {2024-02-20},
urldate = {2024-02-20},
publisher = {Thirty-Sixth Annual Conference on Innovative Applications of Artificial Intelligence (IAAI-24)},
abstract = {Snow Water-Equivalent (SWE) \textendash the amount of water available if snowpack is melted \textendash is a key decision variable used by water management agencies to make irrigation, flood control, power generation and drought management decisions. SWE values vary spatiotemporally \textendash affected by weather, topography and other environmental factors. While daily SWE can be measured by Snow Telemetry (SNOTEL) stations with requisite instrumentation, such stations are spatially sparse requiring interpolation techniques to create spatiotemporally complete data. While recent efforts have explored machine learning (ML) for SWE prediction, a number of recent ML advances have yet to be considered. The main contribution of this paper is to explore one such ML advance, attention mechanisms, for SWE prediction. Our hypothesis is that attention has a unique ability to capture and exploit correlations that may exist across locations or the temporal spectrum (or both). We present a generic attention-based modeling framework for SWE prediction and adapt it to capture spatial attention and temporal attention. Our experimental results on 323 SNOTEL stations in the Western U.S. demonstrate that our attention-based models outperform other machine learning approaches. We also provide key results highlighting the differences between spatial and temporal attention in this context and a roadmap toward deployment for generating spatially-complete SWE maps.},
note = {arXiv:2311.03388 [physics]},
keywords = {AI, Snow Water Equivalent, Water},
pubstate = {published},
tppubtype = {inproceedings}
}
Alan Fern; Margaret Burnett; Joseph Davidson; Janardhan Rao Doppa; Paola Pesantez-Cabrera; Ananth Kalyanaraman
AgAID Institute—AI for agricultural labor and decision support Journal Article
In: AI Magazine, vol. n/a, no. n/a, 2024, ISSN: 2371-9621, (_eprint: https://onlinelibrary.wiley.com/doi/pdf/10.1002/aaai.12156).
Abstract | Links | BibTeX | Tags: AI, Farm Ops, Humans, Labor, Water
@article{fern_agaid_nodate,
title = {AgAID Institute\textemdashAI for agricultural labor and decision support},
author = {Alan Fern and Margaret Burnett and Joseph Davidson and Janardhan Rao Doppa and Paola Pesantez-Cabrera and Ananth Kalyanaraman},
url = {https://onlinelibrary.wiley.com/doi/abs/10.1002/aaai.12156},
doi = {10.1002/aaai.12156},
issn = {2371-9621},
year = {2024},
date = {2024-02-16},
urldate = {2024-02-16},
journal = {AI Magazine},
volume = {n/a},
number = {n/a},
abstract = {The AgAID Institute is a National AI Research Institute focused on developing AI solutions for specialty crop agriculture. Specialty crops include a variety of fruits and vegetables, nut trees, grapes, berries, and different types of horticultural crops. In the United States, the specialty crop industry accounts for a multibillion dollar industry with over 300 crops grown just along the U.S. west coast. Specialty crop agriculture presents several unique challenges: they are labor-intensive, are easily impacted by weather extremities, and are grown mostly on irrigated lands and hence are dependent on water. The AgAID Institute aims to develop AI solutions to address these challenges, particularly in the face of workforce shortages, water scarcity, and extreme weather events. Addressing this host of challenges requires advancing foundational AI research, including spatio-temporal system modeling, robot sensing and control, multiscale site-specific decision support, and designing effective human\textendashAI workflows. This article provides examples of current AgAID efforts and points to open directions to be explored.},
note = {_eprint: https://onlinelibrary.wiley.com/doi/pdf/10.1002/aaai.12156},
keywords = {AI, Farm Ops, Humans, Labor, Water},
pubstate = {published},
tppubtype = {article}
}
Kelley Moyers; John T. Abatzoglou; Alvar Escriva-Bou; Josué Medellín-Azuara; Joshua H. Viers
In: PLOS Water, vol. 3, no. 3, pp. 1-17, 2024.
Abstract | Links | BibTeX | Tags: Water
@article{10.1371/journal.pwat.0000184,
title = {An invisible water surcharge: Climate warming increases crop water demand in the San Joaquin Valley’s groundwater-dependent irrigated agriculture},
author = {Kelley Moyers and John T. Abatzoglou and Alvar Escriva-Bou and Josu\'{e} Medell\'{i}n-Azuara and Joshua H. Viers},
url = {https://doi.org/10.1371/journal.pwat.0000184},
doi = {10.1371/journal.pwat.0000184},
year = {2024},
date = {2024-01-01},
urldate = {2024-01-01},
journal = {PLOS Water},
volume = {3},
number = {3},
pages = {1-17},
publisher = {Public Library of Science},
abstract = {California’s bountiful San Joaquin Valley (SJV), a critical region for global fruit and nut production, has withstood two severe, multi-year droughts in the past decade, exacerbated by record-breaking high temperature and evaporative demand. We employed climate data and crop coefficients to estimate the crop water demand in the SJV over the past forty years. Our approach, using crop coefficients for Penman-Montieth modeled evapotranspiration, focused on the climate effects on crop water demand, avoiding the confounding factors of changing land use and management practices that are present in actual evapotranspiration. We demonstrate that increases in crop water demand explain half of the cumulative deficits of the agricultural water balance since 1980, exacerbating water reliance on depleting groundwater supplies and fluctuating surface water imports. We call this phenomenon of climate-induced increased crop water demand an invisible water surcharge. We found that in the past decade, this invisible water surcharge on agriculture has increased the crop water demand in the SJV by 4.4% with respect to the 1980\textendash2011 timeframe\textemdashmore than 800 GL per year, a volume as large as a major reservoir in the SJV. Despite potential agronomic adaptation and crop response to climate warming, increased crop water demand adds a stressor to the sustainability of the global fruit and nut supply and calls for changes in management and policies to consider the shifting hydroclimate.},
keywords = {Water},
pubstate = {published},
tppubtype = {article}
}
2023
Ghosh, Subhankar; Belkhouja, Taha; Yan, Yan; Doppa, Janardhan Rao
Improving Uncertainty Quantification of Deep Classifiers via Neighborhood Conformal Prediction: Novel Algorithm and Theoretical Analysis Journal Article
In: Proceedings of the AAAI Conference on Artificial Intelligence, vol. 37, no. 6, pp. 7722–7730, 2023, ISSN: 2374-3468, (Number: 6).
Abstract | Links | BibTeX | Tags: AI, Water
@article{ghosh_improving_2023,
title = {Improving Uncertainty Quantification of Deep Classifiers via Neighborhood Conformal Prediction: Novel Algorithm and Theoretical Analysis},
author = {Ghosh, Subhankar and Belkhouja, Taha and Yan, Yan and Doppa, Janardhan Rao},
url = {https://ojs.aaai.org/index.php/AAAI/article/view/25936},
doi = {10.1609/aaai.v37i6.25936},
issn = {2374-3468},
year = {2023},
date = {2023-06-01},
urldate = {2023-06-01},
journal = {Proceedings of the AAAI Conference on Artificial Intelligence},
volume = {37},
number = {6},
pages = {7722\textendash7730},
abstract = {Safe deployment of deep neural networks in high-stake real-world applications require theoretically sound uncertainty quantification. Conformal prediction (CP) is a principled framework for uncertainty quantification of deep models in the form of prediction set for classification tasks with a user-specified coverage (i.e., true class label is contained with high probability). This paper proposes a novel algorithm referred to as Neighborhood Conformal Prediction (NCP) to improve the efficiency of uncertainty quantification from CP for deep classifiers (i.e., reduce prediction set size). The key idea behind NCP is to use the learned representation of the neural network to identify k nearest-neighbor calibration examples for a given testing input and assign them importance weights proportional to their distance to create adaptive prediction sets. We theoretically show that if the learned data representation of the neural network satisfies some mild conditions, NCP will produce smaller prediction sets than traditional CP algorithms. Our comprehensive experiments on CIFAR-10, CIFAR-100, and ImageNet datasets using diverse deep neural networks strongly demonstrate that NCP leads to significant reduction in prediction set size over prior CP methods.},
note = {Number: 6},
keywords = {AI, Water},
pubstate = {published},
tppubtype = {article}
}
Abatzoglou, John T.; McEvoy, Daniel J.; Nauslar, Nicholas J.; Hegewisch, Katherine C.; Huntington, Justin L.
Downscaled subseasonal fire danger forecast skill across the contiguous United States Journal Article
In: Atmospheric Science Letters, vol. 24, no. 8, pp. e1165, 2023, ISSN: 1530-261X, (_eprint: https://onlinelibrary.wiley.com/doi/pdf/10.1002/asl.1165).
Abstract | Links | BibTeX | Tags: Fallow, Water
@article{abatzoglou_downscaled_2023,
title = {Downscaled subseasonal fire danger forecast skill across the contiguous United States},
author = {Abatzoglou, John T. and McEvoy, Daniel J. and Nauslar, Nicholas J. and Hegewisch, Katherine C. and Huntington, Justin L.},
url = {https://onlinelibrary.wiley.com/doi/abs/10.1002/asl.1165},
doi = {10.1002/asl.1165},
issn = {1530-261X},
year = {2023},
date = {2023-01-01},
urldate = {2023-01-01},
journal = {Atmospheric Science Letters},
volume = {24},
number = {8},
pages = {e1165},
abstract = {The increasing complexity and impacts of fire seasons in the United States have prompted efforts to improve early warning systems for wildland fire management. Outlooks of potential fire activity at lead-times of several weeks can help in wildland fire resource allocation as well as complement short-term meteorological forecasts for ongoing fire events. Here, we describe an experimental system for developing downscaled ensemble-based subseasonal forecasts for the contiguous US using NCEP's operational Climate Forecast System version 2 model. These forecasts are used to calculate forecasted fire danger indices from the United States (US) National Fire Danger Rating System in addition to forecasts of evaporative demand. We further illustrate the skill of subseasonal forecasts on weekly timescales using hindcasts from 2011 to 2021. Results show that while forecast skill degrades with time, statistically significant week 3 correlative skill was found for 76% and 30% of the contiguous US for Energy Release Component and evaporative demand, respectively. These results highlight the potential value of experimental subseasonal forecasts in complementing existing information streams in weekly-to-monthly fire business decision making for suppression-based decisions and geographic reallocation of resources during the fire season, as well for proactive fire management actions outside of the core fire season.},
note = {_eprint: https://onlinelibrary.wiley.com/doi/pdf/10.1002/asl.1165},
keywords = {Fallow, Water},
pubstate = {published},
tppubtype = {article}
}
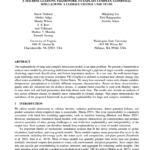
Tanvir Ferdousi; Mingliang Liu; Kirti Rajagopalan; Jennifer Adam; Abhijin Adiga; Mandy Wilson; SS Ravi; Anil Vullikanti; Madhav V Marathe; Samarth Swarup
A Machine Learning Framework to Explain Complex Geospatial Simulations: A Climate Change Case Study Proceedings Article
In: 2023.
Abstract | Links | BibTeX | Tags: AI, Water
@inproceedings{ferdousi_machine_2023,
title = {A Machine Learning Framework to Explain Complex Geospatial Simulations: A Climate Change Case Study},
author = {Tanvir Ferdousi and Mingliang Liu and Kirti Rajagopalan and Jennifer Adam and Abhijin Adiga and Mandy Wilson and SS Ravi and Anil Vullikanti and Madhav V Marathe and Samarth Swarup},
url = {https://tanvir-ferdousi.github.io/assets/pdf/explainability_wsc23.pdf},
year = {2023},
date = {2023-01-01},
urldate = {2023-01-01},
abstract = {The explainability of large and complex simulation models is an open problem. We present a framework to analyze such models by processing multidimensional data through a pipeline of target variable computation, clustering, supervised classification, and feature importance analysis. As a use case, the well-known large-scale hydrology and crop systems simulator VIC-CropSyst is utilized to evaluate how climate change may affect water availability in Washington, United States. We study how snowmelt varies with climate variables (temperature, precipitation) to identify different response characteristics. Based on these characteristics, spatial units are clustered into six distinct classes. A random forest classifier is used with Shapley values to rank static soil and land parameters that help detect each class. The results also include an analysis of risk across different classes to identify areas vulnerable to climate change. This paper demonstrates the usefulness of the proposed framework in providing explainability for large and complex simulations.},
keywords = {AI, Water},
pubstate = {published},
tppubtype = {inproceedings}
}
2022
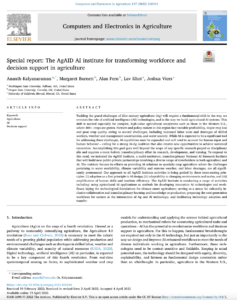
Kalyanaraman, Ananth; Burnett, Margaret; Fern, Alan; Khot, Lav; Viers, Joshua
Special report: The AgAID AI institute for transforming workforce and decision support in agriculture Journal Article
In: Computers and Electronics in Agriculture, vol. 197, pp. 106944, 2022, ISSN: 0168-1699.
Abstract | Links | BibTeX | Tags: AI, Education, Farm Ops, Humans, Labor, Water
@article{kalyanaraman_special_2022,
title = {Special report: The AgAID AI institute for transforming workforce and decision support in agriculture},
author = {Kalyanaraman, Ananth and Burnett, Margaret and Fern, Alan and Khot, Lav and Viers, Joshua},
url = {https://www.sciencedirect.com/science/article/pii/S0168169922002617},
doi = {10.1016/j.compag.2022.106944},
issn = {0168-1699},
year = {2022},
date = {2022-06-01},
urldate = {2022-08-16},
journal = {Computers and Electronics in Agriculture},
volume = {197},
pages = {106944},
abstract = {Tackling the grand challenges of 21st century agriculture (Ag) will require a fundamental shift in the way we envision the role of artificial intelligence (AI) technologies, and in the way we build agricultural AI systems. This shift is needed especially for complex, high-value agricultural ecosystems such as those in the Western U.S., where 300+ crops are grown. Farmers and policy makers in this region face variable profitability, major crop loss and poor crop quality owing to several challenges, including increased labor costs and shortages of skilled workers, weather and management uncertainties, and water scarcity. While AI is expected to be a significant tool for addressing these challenges, AI capabilities must be expanded and will need to account for human input and human behavior \textendash calling for a strong AI-Ag coalition that also creates new opportunities to achieve sustained innovation. Accomplishing this goal goes well beyond the scope of any specific research project or disciplinary silo and requires a more holistic transdisciplinary effort in research, development, and training. To respond to this need, we initiated the AgAID Institute, a multi-institution, transdisciplinary National AI Research Institute that will build new public-private partnerships involving a diverse range of stakeholders in both agriculture and AI. The institute focuses its efforts on providing AI solutions to specialty crop agriculture where the challenges pertaining to water availability, climate variability and extreme weather, and labor shortages, are all significantly pronounced. Our approach to all AgAID Institute activities is being guided by three cross-cutting principles: (i) adoption as a first principle in AI design; (ii) adaptability to changing environments and scales, and (iii) amplification of human skills and machine efficiency. The AgAID Institute is conducting a range of activities including: using agricultural AI applications as testbeds for developing innovative AI technologies and workflows; laying the technological foundations for climate-smart agriculture; serving as a nexus for culturally inclusive collaborative and transdisciplinary learning and knowledge co-production; preparing the next generation workforce for careers at the intersection of Ag and AI technology; and facilitating technology adoption and transfer.},
keywords = {AI, Education, Farm Ops, Humans, Labor, Water},
pubstate = {published},
tppubtype = {article}
}