2024
Uddhav Bhattarai; Qin Zhang; Manoj Karkee
Design, Integration, and Field Evaluation of a Robotic Blossom Thinning System for Tree Fruit Crops Journal Article
In: Field Robotics, 2024, (arXiv:2304.04919 [cs]).
Abstract | Links | BibTeX | Tags: AI, Humans, Labor, Thinning
@article{bhattarai_design_2023,
title = {Design, Integration, and Field Evaluation of a Robotic Blossom Thinning System for Tree Fruit Crops},
author = {Uddhav Bhattarai and Qin Zhang and Manoj Karkee},
url = {http://arxiv.org/abs/2304.04919},
doi = {10.48550/arXiv.2304.04919},
year = {2024},
date = {2024-03-07},
urldate = {2024-03-07},
journal = {Field Robotics},
publisher = {arXiv},
abstract = {The US apple industry relies heavily on semi-skilled manual labor force for essential field operations such as training, pruning, blossom and green fruit thinning, and harvesting. Blossom thinning is one of the crucial crop load management practices to achieve desired crop load, fruit quality, and return bloom. While several techniques such as chemical, and mechanical thinning are available for large-scale blossom thinning such approaches often yield unpredictable thinning results and may cause damage the canopy, spurs, and leaf tissue. Hence, growers still depend on laborious, labor intensive and expensive manual hand blossom thinning for desired thinning outcomes. This research presents a robotic solution for blossom thinning in apple orchards using a computer vision system with artificial intelligence, a six degrees of freedom robotic manipulator, and an electrically actuated miniature end-effector for robotic blossom thinning. The integrated robotic system was evaluated in a commercial apple orchard which showed promising results for targeted and selective blossom thinning. Two thinning approaches, center and boundary thinning, were investigated to evaluate the system ability to remove varying proportion of flowers from apple flower clusters. During boundary thinning the end effector was actuated around the cluster boundary while center thinning involved end-effector actuation only at the cluster centroid for a fixed duration of 2 seconds. The boundary thinning approach thinned 67.2% of flowers from the targeted clusters with a cycle time of 9.0 seconds per cluster, whereas center thinning approach thinned 59.4% of flowers with a cycle time of 7.2 seconds per cluster. When commercially adopted, the proposed system could help address problems faced by apple growers with current hand, chemical, and mechanical blossom thinning approaches.},
note = {arXiv:2304.04919 [cs]},
keywords = {AI, Humans, Labor, Thinning},
pubstate = {published},
tppubtype = {article}
}
Alan Fern; Margaret Burnett; Joseph Davidson; Janardhan Rao Doppa; Paola Pesantez-Cabrera; Ananth Kalyanaraman
AgAID Institute—AI for agricultural labor and decision support Journal Article
In: AI Magazine, vol. n/a, no. n/a, 2024, ISSN: 2371-9621, (_eprint: https://onlinelibrary.wiley.com/doi/pdf/10.1002/aaai.12156).
Abstract | Links | BibTeX | Tags: AI, Farm Ops, Humans, Labor, Water
@article{fern_agaid_nodate,
title = {AgAID Institute\textemdashAI for agricultural labor and decision support},
author = {Alan Fern and Margaret Burnett and Joseph Davidson and Janardhan Rao Doppa and Paola Pesantez-Cabrera and Ananth Kalyanaraman},
url = {https://onlinelibrary.wiley.com/doi/abs/10.1002/aaai.12156},
doi = {10.1002/aaai.12156},
issn = {2371-9621},
year = {2024},
date = {2024-02-16},
urldate = {2024-02-16},
journal = {AI Magazine},
volume = {n/a},
number = {n/a},
abstract = {The AgAID Institute is a National AI Research Institute focused on developing AI solutions for specialty crop agriculture. Specialty crops include a variety of fruits and vegetables, nut trees, grapes, berries, and different types of horticultural crops. In the United States, the specialty crop industry accounts for a multibillion dollar industry with over 300 crops grown just along the U.S. west coast. Specialty crop agriculture presents several unique challenges: they are labor-intensive, are easily impacted by weather extremities, and are grown mostly on irrigated lands and hence are dependent on water. The AgAID Institute aims to develop AI solutions to address these challenges, particularly in the face of workforce shortages, water scarcity, and extreme weather events. Addressing this host of challenges requires advancing foundational AI research, including spatio-temporal system modeling, robot sensing and control, multiscale site-specific decision support, and designing effective human\textendashAI workflows. This article provides examples of current AgAID efforts and points to open directions to be explored.},
note = {_eprint: https://onlinelibrary.wiley.com/doi/pdf/10.1002/aaai.12156},
keywords = {AI, Farm Ops, Humans, Labor, Water},
pubstate = {published},
tppubtype = {article}
}
Ranjan Sapkota; Dawood Ahmed; Martin Churuvija; Manoj Karkee
Immature Green Apple Detection and Sizing in Commercial Orchards using YOLOv8 and Shape Fitting Techniques Journal Article
In: IEEE Access, pp. 1–1, 2024, ISSN: 2169-3536, (Conference Name: IEEE Access).
Abstract | Links | BibTeX | Tags: AI, Labor
@article{sapkota_immature_2024,
title = {Immature Green Apple Detection and Sizing in Commercial Orchards using YOLOv8 and Shape Fitting Techniques},
author = {Ranjan Sapkota and Dawood Ahmed and Martin Churuvija and Manoj Karkee},
url = {https://ieeexplore.ieee.org/document/10474021},
doi = {10.1109/ACCESS.2024.3378261},
issn = {2169-3536},
year = {2024},
date = {2024-01-01},
urldate = {2024-01-01},
journal = {IEEE Access},
pages = {1\textendash1},
abstract = {Detecting and estimating size of apples during the early stages of growth is crucial for predicting yield, pest management, and making informed decisions related to crop-load management, harvest and post-harvest logistics, and marketing. Traditional fruit size measurement methods are laborious and time-consuming. This study employs the state-of-the-art YOLOv8 object detection and instance segmentation algorithm in conjunction with geometric shape fitting techniques on 3D point cloud data to accurately determine the size of immature green apples (or fruitlet) in a commercial orchard environment. The methodology utilized two RGB-D sensors: Intel RealSense D435i and Microsoft Azure Kinect DK. Notably, the YOLOv8 instance segmentation models exhibited proficiency in immature green apple detection, with the YOLOv8m-seg model achieving the highest [email protected] and [email protected] scores of 0.94 and 0.91, respectively. Using the ellipsoid fitting technique on images from the Azure Kinect, we achieved an RMSE of 2.35 mm, MAE of 1.66 mm, MAPE of 6.15 mm, and an R-squared value of 0.9 in estimating the size of apple fruitlets. Challenges such as partial occlusion caused some error in accurately delineating and sizing green apples using the YOLOv8-based segmentation technique, particularly in fruit clusters. In a comparison with 102 outdoor samples, the size estimation technique performed better on the images acquired with Microsoft Azure Kinect than the same with Intel Realsense D435i. This superiority is evident from the metrics: the RMSE values (2.35 mm for Azure Kinect vs. 9.65 mm for Realsense D435i), MAE values (1.66 mm for Azure Kinect vs. 7.8 mm for Realsense D435i), and the R-squared values (0.9 for Azure Kinect vs. 0.77 for Realsense D435i). This study demonstrated the feasibility of accurately sizing immature green fruit in early growth stages using the combined 3D sensing and shape-fitting technique, which shows promise for improved precision agricultural operations such as optimal crop-load management in orchards.},
note = {Conference Name: IEEE Access},
keywords = {AI, Labor},
pubstate = {published},
tppubtype = {article}
}
2023
Wang, T.; Sankari, P.; Brown, J.; Paudel, A.; He, L.; Karkee, M.; Thompson, A.; Grimm, C.; Davidson, J.r.; Todorovic, S.
Automatic estimation of trunk cross sectional area using deep learning Proceedings Article
In: Precision agriculture, pp. 491–498, Wageningen Academic Publishers, 2023, ISBN: 978-90-8686-393-8, (Section: 62).
Links | BibTeX | Tags: AI, Labor, Pruning
@inproceedings{wang_62_2023,
title = {Automatic estimation of trunk cross sectional area using deep learning},
author = {Wang, T. and Sankari, P. and Brown, J. and Paudel, A. and He, L. and Karkee, M. and Thompson, A. and Grimm, C. and Davidson, J.r. and Todorovic, S.},
url = {https://www.wageningenacademic.com/doi/10.3920/978-90-8686-947-3_62},
doi = {10.3920/978-90-8686-947-3_62},
isbn = {978-90-8686-393-8},
year = {2023},
date = {2023-07-01},
urldate = {2023-07-01},
booktitle = {Precision agriculture},
pages = {491\textendash498},
publisher = {Wageningen Academic Publishers},
note = {Section: 62},
keywords = {AI, Labor, Pruning},
pubstate = {published},
tppubtype = {inproceedings}
}
2022
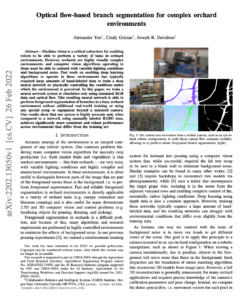
You, Alexander; Grimm, Cindy; Davidson, Joseph R.
Optical flow-based branch segmentation for complex orchard environments Proceedings Article
In: 2022 IEEE/RSJ International Conference on Intelligent Robots and Systems (IROS), pp. 9180–9186, arXiv, 2022, (ISSN: 2153-0866).
Abstract | Links | BibTeX | Tags: AI, Labor, Pruning, Thinning
@inproceedings{you_optical_2022,
title = {Optical flow-based branch segmentation for complex orchard environments},
author = {You, Alexander and Grimm, Cindy and Davidson, Joseph R.},
url = {http://arxiv.org/abs/2202.13050},
doi = {10.1109/IROS47612.2022.9982017},
year = {2022},
date = {2022-10-01},
urldate = {2022-10-01},
booktitle = {2022 IEEE/RSJ International Conference on Intelligent Robots and Systems (IROS)},
pages = {9180\textendash9186},
publisher = {arXiv},
abstract = {Machine vision is a critical subsystem for enabling robots to be able to perform a variety of tasks in orchard environments. However, orchards are highly visually complex environments, and computer vision algorithms operating in them must be able to contend with variable lighting conditions and background noise. Past work on enabling deep learning algorithms to operate in these environments has typically required large amounts of hand-labeled data to train a deep neural network or physically controlling the conditions under which the environment is perceived. In this paper, we train a neural network system in simulation only using simulated RGB data and optical flow. This resulting neural network is able to perform foreground segmentation of branches in a busy orchard environment without additional real-world training or using any special setup or equipment beyond a standard camera. Our results show that our system is highly accurate and, when compared to a network using manually labeled RGBD data, achieves significantly more consistent and robust performance across environments that differ from the training set.},
note = {ISSN: 2153-0866},
keywords = {AI, Labor, Pruning, Thinning},
pubstate = {published},
tppubtype = {inproceedings}
}
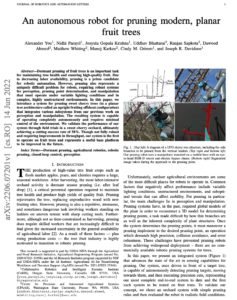
Alexander You; Nidhi Parayil; Josyula Gopala Krishna; Uddhav Bhattarai; Ranjan Sapkota; Dawood Ahmed; Matthew Whiting; Manoj Karkee; Cindy M. Grimm; Joseph R. Davidson
An autonomous robot for pruning modern, planar fruit trees Proceedings Article
In: arXiv, 2022, (arXiv:2206.07201 [cs]).
Abstract | Links | BibTeX | Tags: AI, Labor, Pruning
@inproceedings{you_autonomous_2022,
title = {An autonomous robot for pruning modern, planar fruit trees},
author = { Alexander You and Nidhi Parayil and Josyula Gopala Krishna and Uddhav Bhattarai and Ranjan Sapkota and Dawood Ahmed and Matthew Whiting and Manoj Karkee and Cindy M. Grimm and Joseph R. Davidson},
url = {http://arxiv.org/abs/2206.07201},
doi = {10.48550/arXiv.2206.07201},
year = {2022},
date = {2022-06-01},
urldate = {2022-06-01},
publisher = {arXiv},
abstract = {Dormant pruning of fruit trees is an important task for maintaining tree health and ensuring high-quality fruit. Due to decreasing labor availability, pruning is a prime candidate for robotic automation. However, pruning also represents a uniquely difficult problem for robots, requiring robust systems for perception, pruning point determination, and manipulation that must operate under variable lighting conditions and in complex, highly unstructured environments. In this paper, we introduce a system for pruning sweet cherry trees (in a planar tree architecture called an upright fruiting offshoot configuration) that integrates various subsystems from our previous work on perception and manipulation. The resulting system is capable of operating completely autonomously and requires minimal control of the environment. We validate the performance of our system through field trials in a sweet cherry orchard, ultimately achieving a cutting success rate of 58%. Though not fully robust and requiring improvements in throughput, our system is the first to operate on fruit trees and represents a useful base platform to be improved in the future.},
note = {arXiv:2206.07201 [cs]},
keywords = {AI, Labor, Pruning},
pubstate = {published},
tppubtype = {inproceedings}
}
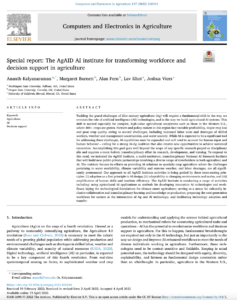
Kalyanaraman, Ananth; Burnett, Margaret; Fern, Alan; Khot, Lav; Viers, Joshua
Special report: The AgAID AI institute for transforming workforce and decision support in agriculture Journal Article
In: Computers and Electronics in Agriculture, vol. 197, pp. 106944, 2022, ISSN: 0168-1699.
Abstract | Links | BibTeX | Tags: AI, Education, Farm Ops, Humans, Labor, Water
@article{kalyanaraman_special_2022,
title = {Special report: The AgAID AI institute for transforming workforce and decision support in agriculture},
author = {Kalyanaraman, Ananth and Burnett, Margaret and Fern, Alan and Khot, Lav and Viers, Joshua},
url = {https://www.sciencedirect.com/science/article/pii/S0168169922002617},
doi = {10.1016/j.compag.2022.106944},
issn = {0168-1699},
year = {2022},
date = {2022-06-01},
urldate = {2022-08-16},
journal = {Computers and Electronics in Agriculture},
volume = {197},
pages = {106944},
abstract = {Tackling the grand challenges of 21st century agriculture (Ag) will require a fundamental shift in the way we envision the role of artificial intelligence (AI) technologies, and in the way we build agricultural AI systems. This shift is needed especially for complex, high-value agricultural ecosystems such as those in the Western U.S., where 300+ crops are grown. Farmers and policy makers in this region face variable profitability, major crop loss and poor crop quality owing to several challenges, including increased labor costs and shortages of skilled workers, weather and management uncertainties, and water scarcity. While AI is expected to be a significant tool for addressing these challenges, AI capabilities must be expanded and will need to account for human input and human behavior \textendash calling for a strong AI-Ag coalition that also creates new opportunities to achieve sustained innovation. Accomplishing this goal goes well beyond the scope of any specific research project or disciplinary silo and requires a more holistic transdisciplinary effort in research, development, and training. To respond to this need, we initiated the AgAID Institute, a multi-institution, transdisciplinary National AI Research Institute that will build new public-private partnerships involving a diverse range of stakeholders in both agriculture and AI. The institute focuses its efforts on providing AI solutions to specialty crop agriculture where the challenges pertaining to water availability, climate variability and extreme weather, and labor shortages, are all significantly pronounced. Our approach to all AgAID Institute activities is being guided by three cross-cutting principles: (i) adoption as a first principle in AI design; (ii) adaptability to changing environments and scales, and (iii) amplification of human skills and machine efficiency. The AgAID Institute is conducting a range of activities including: using agricultural AI applications as testbeds for developing innovative AI technologies and workflows; laying the technological foundations for climate-smart agriculture; serving as a nexus for culturally inclusive collaborative and transdisciplinary learning and knowledge co-production; preparing the next generation workforce for careers at the intersection of Ag and AI technology; and facilitating technology adoption and transfer.},
keywords = {AI, Education, Farm Ops, Humans, Labor, Water},
pubstate = {published},
tppubtype = {article}
}
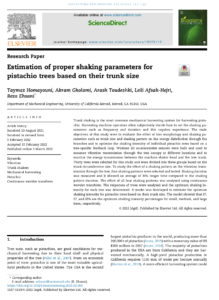
Homayouni, Taymaz; Gholami, Akram; Toudeshki, Arash; Afsah-Hejri, Leili; Ehsani, Reza
Estimation of proper shaking parameters for pistachio trees based on their trunk size Journal Article
In: Biosystems Engineering, vol. 216, pp. 121–131, 2022, ISSN: 1537-5110.
Abstract | Links | BibTeX | Tags: Labor
@article{homayouni_estimation_2022,
title = {Estimation of proper shaking parameters for pistachio trees based on their trunk size},
author = {Homayouni, Taymaz and Gholami, Akram and Toudeshki, Arash and Afsah-Hejri, Leili and Ehsani, Reza},
url = {https://www.sciencedirect.com/science/article/pii/S1537511022000411},
doi = {10.1016/j.biosystemseng.2022.02.008},
issn = {1537-5110},
year = {2022},
date = {2022-04-01},
urldate = {2022-07-07},
journal = {Biosystems Engineering},
volume = {216},
pages = {121\textendash131},
abstract = {Trunk shaking is the most common mechanical harvesting system for harvesting pistachio. Harvesting machine operators often subjectively decide how to set the shaking parameters such as frequency and duration and this requires experience. The main objectives of this study were to evaluate the effect of tree morphology and shaking parameters such as trunk size and shaking pattern on the energy distribution through the branches and to optimise the shaking intensity of individual pistachio trees based on a tree-specific feedback loop. Wireless 3D accelerometer sensors were built and used to measure vibration transmission through the tree canopy at different locations and to monitor the energy transmission between the machine shaker head and the tree trunk. Thirty trees were selected for this study and were divided into three groups based on the trunk circumference size. To study the effect of a shaking pattern on the vibration transmission through the tree, four shaking patterns were selected and tested. Shaking duration was measured and it showed an average of 30% longer time compared to the shaking pattern duration. The effect of all four shaking patterns was analysed using continuous wavelet transform. The responses of trees were analysed and the optimum shaking intensity for each tree was determined. A model was developed to estimate the optimum shaking intensity for pistachio trees based on their trunk size. The model showed that 37, 57, and 65% are the optimum shaking intensity percentages for small, medium, and large trees, respectively.},
keywords = {Labor},
pubstate = {published},
tppubtype = {article}
}