2024
Uddhav Bhattarai; Qin Zhang; Manoj Karkee
Design, Integration, and Field Evaluation of a Robotic Blossom Thinning System for Tree Fruit Crops Journal Article
In: Field Robotics, 2024, (arXiv:2304.04919 [cs]).
Abstract | Links | BibTeX | Tags: AI, Humans, Labor, Thinning
@article{bhattarai_design_2023,
title = {Design, Integration, and Field Evaluation of a Robotic Blossom Thinning System for Tree Fruit Crops},
author = {Uddhav Bhattarai and Qin Zhang and Manoj Karkee},
url = {http://arxiv.org/abs/2304.04919},
doi = {10.48550/arXiv.2304.04919},
year = {2024},
date = {2024-03-07},
urldate = {2024-03-07},
journal = {Field Robotics},
publisher = {arXiv},
abstract = {The US apple industry relies heavily on semi-skilled manual labor force for essential field operations such as training, pruning, blossom and green fruit thinning, and harvesting. Blossom thinning is one of the crucial crop load management practices to achieve desired crop load, fruit quality, and return bloom. While several techniques such as chemical, and mechanical thinning are available for large-scale blossom thinning such approaches often yield unpredictable thinning results and may cause damage the canopy, spurs, and leaf tissue. Hence, growers still depend on laborious, labor intensive and expensive manual hand blossom thinning for desired thinning outcomes. This research presents a robotic solution for blossom thinning in apple orchards using a computer vision system with artificial intelligence, a six degrees of freedom robotic manipulator, and an electrically actuated miniature end-effector for robotic blossom thinning. The integrated robotic system was evaluated in a commercial apple orchard which showed promising results for targeted and selective blossom thinning. Two thinning approaches, center and boundary thinning, were investigated to evaluate the system ability to remove varying proportion of flowers from apple flower clusters. During boundary thinning the end effector was actuated around the cluster boundary while center thinning involved end-effector actuation only at the cluster centroid for a fixed duration of 2 seconds. The boundary thinning approach thinned 67.2% of flowers from the targeted clusters with a cycle time of 9.0 seconds per cluster, whereas center thinning approach thinned 59.4% of flowers with a cycle time of 7.2 seconds per cluster. When commercially adopted, the proposed system could help address problems faced by apple growers with current hand, chemical, and mechanical blossom thinning approaches.},
note = {arXiv:2304.04919 [cs]},
keywords = {AI, Humans, Labor, Thinning},
pubstate = {published},
tppubtype = {article}
}
2023
Parayil, N.; You, A.; Grimm, C.; Davidson, J.r.
Follow the leader: a path generator and controller for precision tree scanning with a robotic manipulator Proceedings Article
In: Precision agriculture, pp. 167–174, Wageningen Academic Publishers, 2023, ISBN: 978-90-8686-393-8, (Section: 19).
Links | BibTeX | Tags: Pruning, Thinning
@inproceedings{parayil_19_2023,
title = {Follow the leader: a path generator and controller for precision tree scanning with a robotic manipulator},
author = {Parayil, N. and You, A. and Grimm, C. and Davidson, J.r.},
url = {https://www.wageningenacademic.com/doi/10.3920/978-90-8686-947-3_19},
doi = {10.3920/978-90-8686-947-3_19},
isbn = {978-90-8686-393-8},
year = {2023},
date = {2023-07-01},
urldate = {2023-07-01},
booktitle = {Precision agriculture},
pages = {167\textendash174},
publisher = {Wageningen Academic Publishers},
note = {Section: 19},
keywords = {Pruning, Thinning},
pubstate = {published},
tppubtype = {inproceedings}
}
2022
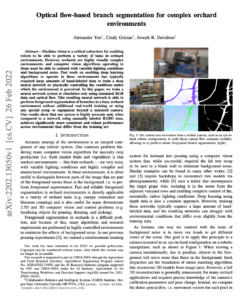
You, Alexander; Grimm, Cindy; Davidson, Joseph R.
Optical flow-based branch segmentation for complex orchard environments Proceedings Article
In: 2022 IEEE/RSJ International Conference on Intelligent Robots and Systems (IROS), pp. 9180–9186, arXiv, 2022, (ISSN: 2153-0866).
Abstract | Links | BibTeX | Tags: AI, Labor, Pruning, Thinning
@inproceedings{you_optical_2022,
title = {Optical flow-based branch segmentation for complex orchard environments},
author = {You, Alexander and Grimm, Cindy and Davidson, Joseph R.},
url = {http://arxiv.org/abs/2202.13050},
doi = {10.1109/IROS47612.2022.9982017},
year = {2022},
date = {2022-10-01},
urldate = {2022-10-01},
booktitle = {2022 IEEE/RSJ International Conference on Intelligent Robots and Systems (IROS)},
pages = {9180\textendash9186},
publisher = {arXiv},
abstract = {Machine vision is a critical subsystem for enabling robots to be able to perform a variety of tasks in orchard environments. However, orchards are highly visually complex environments, and computer vision algorithms operating in them must be able to contend with variable lighting conditions and background noise. Past work on enabling deep learning algorithms to operate in these environments has typically required large amounts of hand-labeled data to train a deep neural network or physically controlling the conditions under which the environment is perceived. In this paper, we train a neural network system in simulation only using simulated RGB data and optical flow. This resulting neural network is able to perform foreground segmentation of branches in a busy orchard environment without additional real-world training or using any special setup or equipment beyond a standard camera. Our results show that our system is highly accurate and, when compared to a network using manually labeled RGBD data, achieves significantly more consistent and robust performance across environments that differ from the training set.},
note = {ISSN: 2153-0866},
keywords = {AI, Labor, Pruning, Thinning},
pubstate = {published},
tppubtype = {inproceedings}
}