2024
Uddhav Bhattarai; Qin Zhang; Manoj Karkee
Design, Integration, and Field Evaluation of a Robotic Blossom Thinning System for Tree Fruit Crops Journal Article
In: Field Robotics, 2024, (arXiv:2304.04919 [cs]).
Abstract | Links | BibTeX | Tags: AI, Humans, Labor, Thinning
@article{bhattarai_design_2023,
title = {Design, Integration, and Field Evaluation of a Robotic Blossom Thinning System for Tree Fruit Crops},
author = {Uddhav Bhattarai and Qin Zhang and Manoj Karkee},
url = {http://arxiv.org/abs/2304.04919},
doi = {10.48550/arXiv.2304.04919},
year = {2024},
date = {2024-03-07},
urldate = {2024-03-07},
journal = {Field Robotics},
publisher = {arXiv},
abstract = {The US apple industry relies heavily on semi-skilled manual labor force for essential field operations such as training, pruning, blossom and green fruit thinning, and harvesting. Blossom thinning is one of the crucial crop load management practices to achieve desired crop load, fruit quality, and return bloom. While several techniques such as chemical, and mechanical thinning are available for large-scale blossom thinning such approaches often yield unpredictable thinning results and may cause damage the canopy, spurs, and leaf tissue. Hence, growers still depend on laborious, labor intensive and expensive manual hand blossom thinning for desired thinning outcomes. This research presents a robotic solution for blossom thinning in apple orchards using a computer vision system with artificial intelligence, a six degrees of freedom robotic manipulator, and an electrically actuated miniature end-effector for robotic blossom thinning. The integrated robotic system was evaluated in a commercial apple orchard which showed promising results for targeted and selective blossom thinning. Two thinning approaches, center and boundary thinning, were investigated to evaluate the system ability to remove varying proportion of flowers from apple flower clusters. During boundary thinning the end effector was actuated around the cluster boundary while center thinning involved end-effector actuation only at the cluster centroid for a fixed duration of 2 seconds. The boundary thinning approach thinned 67.2% of flowers from the targeted clusters with a cycle time of 9.0 seconds per cluster, whereas center thinning approach thinned 59.4% of flowers with a cycle time of 7.2 seconds per cluster. When commercially adopted, the proposed system could help address problems faced by apple growers with current hand, chemical, and mechanical blossom thinning approaches.},
note = {arXiv:2304.04919 [cs]},
keywords = {AI, Humans, Labor, Thinning},
pubstate = {published},
tppubtype = {article}
}
Alan Fern; Margaret Burnett; Joseph Davidson; Janardhan Rao Doppa; Paola Pesantez-Cabrera; Ananth Kalyanaraman
AgAID Institute—AI for agricultural labor and decision support Journal Article
In: AI Magazine, vol. n/a, no. n/a, 2024, ISSN: 2371-9621, (_eprint: https://onlinelibrary.wiley.com/doi/pdf/10.1002/aaai.12156).
Abstract | Links | BibTeX | Tags: AI, Farm Ops, Humans, Labor, Water
@article{fern_agaid_nodate,
title = {AgAID Institute\textemdashAI for agricultural labor and decision support},
author = {Alan Fern and Margaret Burnett and Joseph Davidson and Janardhan Rao Doppa and Paola Pesantez-Cabrera and Ananth Kalyanaraman},
url = {https://onlinelibrary.wiley.com/doi/abs/10.1002/aaai.12156},
doi = {10.1002/aaai.12156},
issn = {2371-9621},
year = {2024},
date = {2024-02-16},
urldate = {2024-02-16},
journal = {AI Magazine},
volume = {n/a},
number = {n/a},
abstract = {The AgAID Institute is a National AI Research Institute focused on developing AI solutions for specialty crop agriculture. Specialty crops include a variety of fruits and vegetables, nut trees, grapes, berries, and different types of horticultural crops. In the United States, the specialty crop industry accounts for a multibillion dollar industry with over 300 crops grown just along the U.S. west coast. Specialty crop agriculture presents several unique challenges: they are labor-intensive, are easily impacted by weather extremities, and are grown mostly on irrigated lands and hence are dependent on water. The AgAID Institute aims to develop AI solutions to address these challenges, particularly in the face of workforce shortages, water scarcity, and extreme weather events. Addressing this host of challenges requires advancing foundational AI research, including spatio-temporal system modeling, robot sensing and control, multiscale site-specific decision support, and designing effective human\textendashAI workflows. This article provides examples of current AgAID efforts and points to open directions to be explored.},
note = {_eprint: https://onlinelibrary.wiley.com/doi/pdf/10.1002/aaai.12156},
keywords = {AI, Farm Ops, Humans, Labor, Water},
pubstate = {published},
tppubtype = {article}
}
2023
Bertucci, Donald; Hamid, Md Montaser; Anand, Yashwanthi; Ruangrotsakun, Anita; Tabatabai, Delyar; Perez, Melissa; Kahng, Minsuk
DendroMap: Visual Exploration of Large-Scale Image Datasets for Machine Learning with Treemaps Journal Article
In: IEEE Transactions on Visualization and Computer Graphics, vol. 29, no. 1, pp. 320–330, 2023, ISSN: 1941-0506, (Conference Name: IEEE Transactions on Visualization and Computer Graphics).
Abstract | Links | BibTeX | Tags: AI, Humans
@article{bertucci_dendromap_2023,
title = {DendroMap: Visual Exploration of Large-Scale Image Datasets for Machine Learning with Treemaps},
author = {Bertucci, Donald and Hamid, Md Montaser and Anand, Yashwanthi and Ruangrotsakun, Anita and Tabatabai, Delyar and Perez, Melissa and Kahng, Minsuk},
url = {https://ieeexplore.ieee.org/document/9904448},
doi = {10.1109/TVCG.2022.3209425},
issn = {1941-0506},
year = {2023},
date = {2023-01-01},
urldate = {2023-01-01},
journal = {IEEE Transactions on Visualization and Computer Graphics},
volume = {29},
number = {1},
pages = {320\textendash330},
abstract = {In this paper, we present DendroMap, a novel approach to interactively exploring large-scale image datasets for machine learning (ML). ML practitioners often explore image datasets by generating a grid of images or projecting high-dimensional representations of images into 2-D using dimensionality reduction techniques (e.g., t-SNE). However, neither approach effectively scales to large datasets because images are ineffectively organized and interactions are insufficiently supported. To address these challenges, we develop DendroMap by adapting Treemaps, a well-known visualization technique. DendroMap effectively organizes images by extracting hierarchical cluster structures from high-dimensional representations of images. It enables users to make sense of the overall distributions of datasets and interactively zoom into specific areas of interests at multiple levels of abstraction. Our case studies with widely-used image datasets for deep learning demonstrate that users can discover insights about datasets and trained models by examining the diversity of images, identifying underperforming subgroups, and analyzing classification errors. We conducted a user study that evaluates the effectiveness of DendroMap in grouping and searching tasks by comparing it with a gridified version of t-SNE and found that participants preferred DendroMap. DendroMap is available at https://div-lab.github.io/dendromap/.},
note = {Conference Name: IEEE Transactions on Visualization and Computer Graphics},
keywords = {AI, Humans},
pubstate = {published},
tppubtype = {article}
}
2022
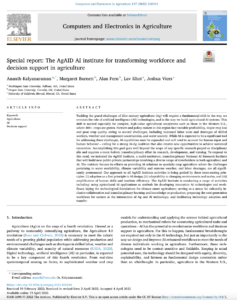
Kalyanaraman, Ananth; Burnett, Margaret; Fern, Alan; Khot, Lav; Viers, Joshua
Special report: The AgAID AI institute for transforming workforce and decision support in agriculture Journal Article
In: Computers and Electronics in Agriculture, vol. 197, pp. 106944, 2022, ISSN: 0168-1699.
Abstract | Links | BibTeX | Tags: AI, Education, Farm Ops, Humans, Labor, Water
@article{kalyanaraman_special_2022,
title = {Special report: The AgAID AI institute for transforming workforce and decision support in agriculture},
author = {Kalyanaraman, Ananth and Burnett, Margaret and Fern, Alan and Khot, Lav and Viers, Joshua},
url = {https://www.sciencedirect.com/science/article/pii/S0168169922002617},
doi = {10.1016/j.compag.2022.106944},
issn = {0168-1699},
year = {2022},
date = {2022-06-01},
urldate = {2022-08-16},
journal = {Computers and Electronics in Agriculture},
volume = {197},
pages = {106944},
abstract = {Tackling the grand challenges of 21st century agriculture (Ag) will require a fundamental shift in the way we envision the role of artificial intelligence (AI) technologies, and in the way we build agricultural AI systems. This shift is needed especially for complex, high-value agricultural ecosystems such as those in the Western U.S., where 300+ crops are grown. Farmers and policy makers in this region face variable profitability, major crop loss and poor crop quality owing to several challenges, including increased labor costs and shortages of skilled workers, weather and management uncertainties, and water scarcity. While AI is expected to be a significant tool for addressing these challenges, AI capabilities must be expanded and will need to account for human input and human behavior \textendash calling for a strong AI-Ag coalition that also creates new opportunities to achieve sustained innovation. Accomplishing this goal goes well beyond the scope of any specific research project or disciplinary silo and requires a more holistic transdisciplinary effort in research, development, and training. To respond to this need, we initiated the AgAID Institute, a multi-institution, transdisciplinary National AI Research Institute that will build new public-private partnerships involving a diverse range of stakeholders in both agriculture and AI. The institute focuses its efforts on providing AI solutions to specialty crop agriculture where the challenges pertaining to water availability, climate variability and extreme weather, and labor shortages, are all significantly pronounced. Our approach to all AgAID Institute activities is being guided by three cross-cutting principles: (i) adoption as a first principle in AI design; (ii) adaptability to changing environments and scales, and (iii) amplification of human skills and machine efficiency. The AgAID Institute is conducting a range of activities including: using agricultural AI applications as testbeds for developing innovative AI technologies and workflows; laying the technological foundations for climate-smart agriculture; serving as a nexus for culturally inclusive collaborative and transdisciplinary learning and knowledge co-production; preparing the next generation workforce for careers at the intersection of Ag and AI technology; and facilitating technology adoption and transfer.},
keywords = {AI, Education, Farm Ops, Humans, Labor, Water},
pubstate = {published},
tppubtype = {article}
}