2023
Wang, T.; Sankari, P.; Brown, J.; Paudel, A.; He, L.; Karkee, M.; Thompson, A.; Grimm, C.; Davidson, J.r.; Todorovic, S.
Automatic estimation of trunk cross sectional area using deep learning Proceedings Article
In: Precision agriculture, pp. 491–498, Wageningen Academic Publishers, 2023, ISBN: 978-90-8686-393-8, (Section: 62).
Links | BibTeX | Tags: AI, Labor, Pruning
@inproceedings{wang_62_2023,
title = {Automatic estimation of trunk cross sectional area using deep learning},
author = {Wang, T. and Sankari, P. and Brown, J. and Paudel, A. and He, L. and Karkee, M. and Thompson, A. and Grimm, C. and Davidson, J.r. and Todorovic, S.},
url = {https://www.wageningenacademic.com/doi/10.3920/978-90-8686-947-3_62},
doi = {10.3920/978-90-8686-947-3_62},
isbn = {978-90-8686-393-8},
year = {2023},
date = {2023-07-01},
urldate = {2023-07-01},
booktitle = {Precision agriculture},
pages = {491\textendash498},
publisher = {Wageningen Academic Publishers},
note = {Section: 62},
keywords = {AI, Labor, Pruning},
pubstate = {published},
tppubtype = {inproceedings}
}
Parayil, N.; You, A.; Grimm, C.; Davidson, J.r.
Follow the leader: a path generator and controller for precision tree scanning with a robotic manipulator Proceedings Article
In: Precision agriculture, pp. 167–174, Wageningen Academic Publishers, 2023, ISBN: 978-90-8686-393-8, (Section: 19).
Links | BibTeX | Tags: Pruning, Thinning
@inproceedings{parayil_19_2023,
title = {Follow the leader: a path generator and controller for precision tree scanning with a robotic manipulator},
author = {Parayil, N. and You, A. and Grimm, C. and Davidson, J.r.},
url = {https://www.wageningenacademic.com/doi/10.3920/978-90-8686-947-3_19},
doi = {10.3920/978-90-8686-947-3_19},
isbn = {978-90-8686-393-8},
year = {2023},
date = {2023-07-01},
urldate = {2023-07-01},
booktitle = {Precision agriculture},
pages = {167\textendash174},
publisher = {Wageningen Academic Publishers},
note = {Section: 19},
keywords = {Pruning, Thinning},
pubstate = {published},
tppubtype = {inproceedings}
}
He, Liqiang; Wei Wang, Albert Chen; Min Sun; Cheng-hao Kuo; Sinisa Todorovic
Bidirectional alignment for domain adaptive detection with transformers Proceedings Article
In: Proceedings of International Conference on Computer Vision, 2023.
Abstract | Links | BibTeX | Tags: Pruning
@inproceedings{noauthor_bidirectional_nodate,
title = {Bidirectional alignment for domain adaptive detection with transformers},
author = {He, Liqiang; Wei Wang, Albert Chen; Min Sun; Cheng-hao Kuo; Sinisa Todorovic},
url = {https://www.amazon.science/publications/bidirectional-alignment-for-domain-adaptive-detection-with-transformers},
year = {2023},
date = {2023-03-08},
urldate = {2023-03-08},
journal = {Amazon Science},
publisher = {Proceedings of International Conference on Computer Vision},
abstract = {We propose a Bidirectional Alignment for domain adaptive Detection with Transformers (BiADT) to improve cross domain object detection performance. Existing adversarial learning based methods use gradient reverse layer (GRL) to reduce the domain gap between the source and target domains in feature…},
keywords = {Pruning},
pubstate = {published},
tppubtype = {inproceedings}
}
2022
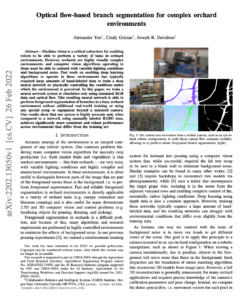
You, Alexander; Grimm, Cindy; Davidson, Joseph R.
Optical flow-based branch segmentation for complex orchard environments Proceedings Article
In: 2022 IEEE/RSJ International Conference on Intelligent Robots and Systems (IROS), pp. 9180–9186, arXiv, 2022, (ISSN: 2153-0866).
Abstract | Links | BibTeX | Tags: AI, Labor, Pruning, Thinning
@inproceedings{you_optical_2022,
title = {Optical flow-based branch segmentation for complex orchard environments},
author = {You, Alexander and Grimm, Cindy and Davidson, Joseph R.},
url = {http://arxiv.org/abs/2202.13050},
doi = {10.1109/IROS47612.2022.9982017},
year = {2022},
date = {2022-10-01},
urldate = {2022-10-01},
booktitle = {2022 IEEE/RSJ International Conference on Intelligent Robots and Systems (IROS)},
pages = {9180\textendash9186},
publisher = {arXiv},
abstract = {Machine vision is a critical subsystem for enabling robots to be able to perform a variety of tasks in orchard environments. However, orchards are highly visually complex environments, and computer vision algorithms operating in them must be able to contend with variable lighting conditions and background noise. Past work on enabling deep learning algorithms to operate in these environments has typically required large amounts of hand-labeled data to train a deep neural network or physically controlling the conditions under which the environment is perceived. In this paper, we train a neural network system in simulation only using simulated RGB data and optical flow. This resulting neural network is able to perform foreground segmentation of branches in a busy orchard environment without additional real-world training or using any special setup or equipment beyond a standard camera. Our results show that our system is highly accurate and, when compared to a network using manually labeled RGBD data, achieves significantly more consistent and robust performance across environments that differ from the training set.},
note = {ISSN: 2153-0866},
keywords = {AI, Labor, Pruning, Thinning},
pubstate = {published},
tppubtype = {inproceedings}
}