2022
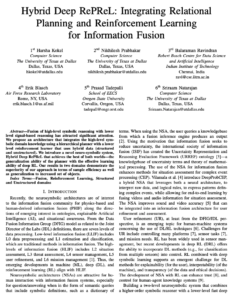
Harsha Kokel; Nikhilesh Prabhakar; Balaraman Ravindran; Erik Blasch; Prasad Tadepalli; Sriraam Natarajan
Hybrid Deep RePReL: Integrating Relational Planning and Reinforcement Learning for Information Fusion Proceedings Article
In: 2022 25th International Conference on Information Fusion (FUSION), pp. 1–8, 2022, ISBN: 978-1-73774-972-1.
Abstract | Links | BibTeX | Tags: AI
@inproceedings{kokel_hybrid_2022,
title = {Hybrid Deep RePReL: Integrating Relational Planning and Reinforcement Learning for Information Fusion},
author = { Harsha Kokel and Nikhilesh Prabhakar and Balaraman Ravindran and Erik Blasch and Prasad Tadepalli and Sriraam Natarajan},
doi = {10.23919/FUSION49751.2022.9841246},
isbn = {978-1-73774-972-1},
year = {2022},
date = {2022-07-01},
urldate = {2022-07-01},
booktitle = {2022 25th International Conference on Information Fusion (FUSION)},
pages = {1--8},
abstract = {Fusion of high-level symbolic reasoning with lower level signal-based reasoning has attracted significant attention. We propose an architecture that integrates the high-level symbolic domain knowledge using a hierarchical planner with a lower level reinforcement learner that uses hybrid data (structured and unstructured). We introduce a novel neuro-symbolic system, Hybrid Deep RePReL that achieves the best of both worlds-the generalization ability of the planner with the effective learning ability of deep RL. Our results in two domains demonstrate the superiority of our approach in terms of sample efficiency as well as generalization to increased set of objects.},
keywords = {AI},
pubstate = {published},
tppubtype = {inproceedings}
}
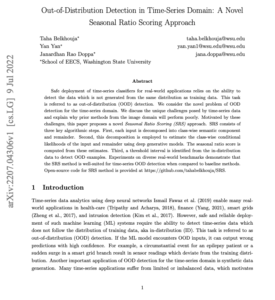
Taha Belkhouja; Yan Yan; Janardhan Rao Doppa
Out-of-Distribution Detection in Time-Series Domain: A Novel Seasonal Ratio Scoring Approach Proceedings Article
In: arXiv, 2022, (arXiv:2207.04306 [cs]).
Abstract | Links | BibTeX | Tags: AI
@inproceedings{belkhouja_out--distribution_2022,
title = {Out-of-Distribution Detection in Time-Series Domain: A Novel Seasonal Ratio Scoring Approach},
author = { Taha Belkhouja and Yan Yan and Janardhan Rao Doppa},
url = {http://arxiv.org/abs/2207.04306},
doi = {10.48550/arXiv.2207.04306},
year = {2022},
date = {2022-07-01},
urldate = {2022-07-01},
publisher = {arXiv},
abstract = {Safe deployment of time-series classifiers for real-world applications relies on the ability to detect the data which is not generated from the same distribution as training data. This task is referred to as out-of-distribution (OOD) detection. We consider the novel problem of OOD detection for the time-series domain. We discuss the unique challenges posed by time-series data and explain why prior methods from the image domain will perform poorly. Motivated by these challenges, this paper proposes a novel textbackslashem Seasonal Ratio Scoring (SRS) approach. SRS consists of three key algorithmic steps. First, each input is decomposed into class-wise semantic component and remainder. Second, this decomposition is employed to estimate the class-wise conditional likelihoods of the input and remainder using deep generative models. The seasonal ratio score is computed from these estimates. Third, a threshold interval is identified from the in-distribution data to detect OOD examples. Experiments on diverse real-world benchmarks demonstrate that the SRS method is well-suited for time-series OOD detection when compared to baseline methods. Open-source code for SRS method is provided at https://github.com/tahabelkhouja/SRS},
note = {arXiv:2207.04306 [cs]},
keywords = {AI},
pubstate = {published},
tppubtype = {inproceedings}
}
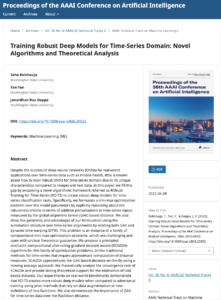
Taha Belkhouja; Yan Yan; Janardhan Rao Doppa
Training Robust Deep Models for Time-Series Domain: Novel Algorithms and Theoretical Analysis Journal Article
In: Proceedings of the AAAI Conference on Artificial Intelligence, vol. 36, no. 6, pp. 6055–6063, 2022, ISSN: 2374-3468, 2159-5399.
Abstract | Links | BibTeX | Tags: AI
@article{belkhouja_training_2022,
title = {Training Robust Deep Models for Time-Series Domain: Novel Algorithms and Theoretical Analysis},
author = { Taha Belkhouja and Yan Yan and Janardhan Rao Doppa},
url = {https://ojs.aaai.org/index.php/AAAI/article/view/20552},
doi = {10.1609/aaai.v36i6.20552},
issn = {2374-3468, 2159-5399},
year = {2022},
date = {2022-06-01},
urldate = {2022-06-01},
journal = {Proceedings of the AAAI Conference on Artificial Intelligence},
volume = {36},
number = {6},
pages = {6055--6063},
abstract = {Despite the success of deep neural networks (DNNs) for real-world applications over time-series data such as mobile health, little is known about how to train robust DNNs for time-series domain due to its unique characteristics compared to images and text data In this paper, we fill this gap by proposing a novel algorithmic framework referred as RObust Training for Time-Series (ROTS) to create robust deep models for time-series classification tasks. Specifically, we formulate a min-max optimization problem over the model parameters by explicitly reasoning about the robustness criteria in terms of additive perturbations to time-series inputs measured by the global alignment kernel (GAK) based distance. We also show the generality and advantages of our formulation using the summation structure over time-series alignments by relating both GAK and dynamic time warping (DTW). This problem is an instance of a family of compositional min-max optimization problems, which are challenging and open with unclear theoretical guarantee. We propose a principled stochastic compositional alternating gradient descent ascent (SCAGDA) algorithm for this family of optimization problems. Unlike traditional methods for timeseries that require approximate computation of distance measures, SCAGDA approximates the GAK based distance onthe-fly using a moving average approach. We theoretically analyze the convergence rate of SCAGDA and provide strong theoretical support for the estimation of GAK based distance. Our experiments on real-world benchmarks demonstrate that ROTS creates more robust deep models when compared to adversarial training using prior methods that rely on data augmentation or new definitions of loss functions. We also demonstrate the importance of GAK for time-series data over the Euclidean distance.},
keywords = {AI},
pubstate = {published},
tppubtype = {article}
}
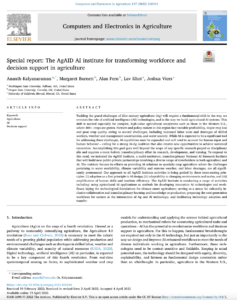
Kalyanaraman, Ananth; Burnett, Margaret; Fern, Alan; Khot, Lav; Viers, Joshua
Special report: The AgAID AI institute for transforming workforce and decision support in agriculture Journal Article
In: Computers and Electronics in Agriculture, vol. 197, pp. 106944, 2022, ISSN: 0168-1699.
Abstract | Links | BibTeX | Tags: AI, Education, Farm Ops, Humans, Labor, Water
@article{kalyanaraman_special_2022,
title = {Special report: The AgAID AI institute for transforming workforce and decision support in agriculture},
author = {Kalyanaraman, Ananth and Burnett, Margaret and Fern, Alan and Khot, Lav and Viers, Joshua},
url = {https://www.sciencedirect.com/science/article/pii/S0168169922002617},
doi = {10.1016/j.compag.2022.106944},
issn = {0168-1699},
year = {2022},
date = {2022-06-01},
urldate = {2022-08-16},
journal = {Computers and Electronics in Agriculture},
volume = {197},
pages = {106944},
abstract = {Tackling the grand challenges of 21st century agriculture (Ag) will require a fundamental shift in the way we envision the role of artificial intelligence (AI) technologies, and in the way we build agricultural AI systems. This shift is needed especially for complex, high-value agricultural ecosystems such as those in the Western U.S., where 300+ crops are grown. Farmers and policy makers in this region face variable profitability, major crop loss and poor crop quality owing to several challenges, including increased labor costs and shortages of skilled workers, weather and management uncertainties, and water scarcity. While AI is expected to be a significant tool for addressing these challenges, AI capabilities must be expanded and will need to account for human input and human behavior \textendash calling for a strong AI-Ag coalition that also creates new opportunities to achieve sustained innovation. Accomplishing this goal goes well beyond the scope of any specific research project or disciplinary silo and requires a more holistic transdisciplinary effort in research, development, and training. To respond to this need, we initiated the AgAID Institute, a multi-institution, transdisciplinary National AI Research Institute that will build new public-private partnerships involving a diverse range of stakeholders in both agriculture and AI. The institute focuses its efforts on providing AI solutions to specialty crop agriculture where the challenges pertaining to water availability, climate variability and extreme weather, and labor shortages, are all significantly pronounced. Our approach to all AgAID Institute activities is being guided by three cross-cutting principles: (i) adoption as a first principle in AI design; (ii) adaptability to changing environments and scales, and (iii) amplification of human skills and machine efficiency. The AgAID Institute is conducting a range of activities including: using agricultural AI applications as testbeds for developing innovative AI technologies and workflows; laying the technological foundations for climate-smart agriculture; serving as a nexus for culturally inclusive collaborative and transdisciplinary learning and knowledge co-production; preparing the next generation workforce for careers at the intersection of Ag and AI technology; and facilitating technology adoption and transfer.},
keywords = {AI, Education, Farm Ops, Humans, Labor, Water},
pubstate = {published},
tppubtype = {article}
}
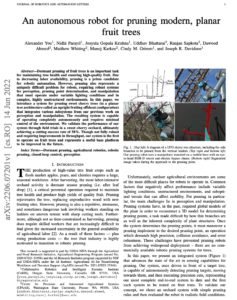
Alexander You; Nidhi Parayil; Josyula Gopala Krishna; Uddhav Bhattarai; Ranjan Sapkota; Dawood Ahmed; Matthew Whiting; Manoj Karkee; Cindy M. Grimm; Joseph R. Davidson
An autonomous robot for pruning modern, planar fruit trees Proceedings Article
In: arXiv, 2022, (arXiv:2206.07201 [cs]).
Abstract | Links | BibTeX | Tags: AI, Labor, Pruning
@inproceedings{you_autonomous_2022,
title = {An autonomous robot for pruning modern, planar fruit trees},
author = { Alexander You and Nidhi Parayil and Josyula Gopala Krishna and Uddhav Bhattarai and Ranjan Sapkota and Dawood Ahmed and Matthew Whiting and Manoj Karkee and Cindy M. Grimm and Joseph R. Davidson},
url = {http://arxiv.org/abs/2206.07201},
doi = {10.48550/arXiv.2206.07201},
year = {2022},
date = {2022-06-01},
urldate = {2022-06-01},
publisher = {arXiv},
abstract = {Dormant pruning of fruit trees is an important task for maintaining tree health and ensuring high-quality fruit. Due to decreasing labor availability, pruning is a prime candidate for robotic automation. However, pruning also represents a uniquely difficult problem for robots, requiring robust systems for perception, pruning point determination, and manipulation that must operate under variable lighting conditions and in complex, highly unstructured environments. In this paper, we introduce a system for pruning sweet cherry trees (in a planar tree architecture called an upright fruiting offshoot configuration) that integrates various subsystems from our previous work on perception and manipulation. The resulting system is capable of operating completely autonomously and requires minimal control of the environment. We validate the performance of our system through field trials in a sweet cherry orchard, ultimately achieving a cutting success rate of 58%. Though not fully robust and requiring improvements in throughput, our system is the first to operate on fruit trees and represents a useful base platform to be improved in the future.},
note = {arXiv:2206.07201 [cs]},
keywords = {AI, Labor, Pruning},
pubstate = {published},
tppubtype = {inproceedings}
}
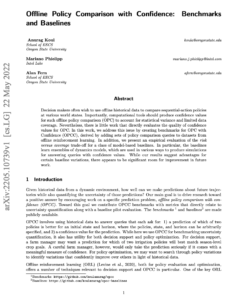
Anurag Koul; Mariano Phielipp; Alan Fern
Offline Policy Comparison with Confidence: Benchmarks and Baselines Journal Article
In: 2022, (arXiv:2205.10739 [cs]).
Abstract | Links | BibTeX | Tags: AI
@article{koul_offline_2022,
title = {Offline Policy Comparison with Confidence: Benchmarks and Baselines},
author = { Anurag Koul and Mariano Phielipp and Alan Fern},
url = {http://arxiv.org/abs/2205.10739},
doi = {10.48550/arXiv.2205.10739},
year = {2022},
date = {2022-05-01},
urldate = {2022-05-01},
publisher = {arXiv},
abstract = {Decision makers often wish to use offline historical data to compare sequential-action policies at various world states. Importantly, computational tools should produce confidence values for such offline policy comparison (OPC) to account for statistical variance and limited data coverage. Nevertheless, there is little work that directly evaluates the quality of confidence values for OPC. In this work, we address this issue by creating benchmarks for OPC with Confidence (OPCC), derived by adding sets of policy comparison queries to datasets from offline reinforcement learning. In addition, we present an empirical evaluation of the risk versus coverage trade-off for a class of model-based baselines. In particular, the baselines learn ensembles of dynamics models, which are used in various ways to produce simulations for answering queries with confidence values. While our results suggest advantages for certain baseline variations, there appears to be significant room for improvement in future work.},
note = {arXiv:2205.10739 [cs]},
keywords = {AI},
pubstate = {published},
tppubtype = {article}
}
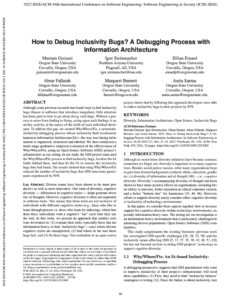
Mariam Guizani; Igor Steinmacher; Jillian Emard; Abrar Fallatah; Margaret Burnett; Anita Sarma
How to Debug Inclusivity Bugs? A Debugging Process with Information Architecture Proceedings Article
In: 2022 IEEE/ACM 44th International Conference on Software Engineering: Software Engineering in Society (ICSE-SEIS), pp. 90–101, IEEE Computer Society, 2022, ISBN: 978-1-66549-594-3.
Abstract | Links | BibTeX | Tags: Human-Computer Interaction
@inproceedings{guizani_how_2022,
title = {How to Debug Inclusivity Bugs? A Debugging Process with Information Architecture},
author = { Mariam Guizani and Igor Steinmacher and Jillian Emard and Abrar Fallatah and Margaret Burnett and Anita Sarma},
url = {https://www.computer.org/csdl/proceedings-article/icse-seis/2022/959400a090/1EmrhU2EkcE},
doi = {10.1109/ICSE-SEIS55304.2022.9794009},
isbn = {978-1-66549-594-3},
year = {2022},
date = {2022-05-01},
urldate = {2022-05-01},
booktitle = {2022 IEEE/ACM 44th International Conference on Software Engineering: Software Engineering in Society (ICSE-SEIS)},
pages = {90--101},
publisher = {IEEE Computer Society},
abstract = {Although some previous research has found ways to find inclusivity bugs (biases in software that introduce inequities), little attention has been paid to how to go about fixing such bugs. Without a process to move from finding to fixing, acting upon such findings is an ad-hoc activity, at the mercy of the skills of each individual developer. To address this gap, we created Why/Where/Fix, a systematic inclusivity debugging process whose inclusivity fault localization harnesses Information Architecture(IA)-the way user-facing information is organized, structured and labeled. We then conducted a multi-stage qualitative empirical evaluation of the effectiveness of Why/Where/Fix, using an Open Source Software (OSS) project\'s infrastructure as our setting. In our study, the OSS project team used the Why/Where/Fix process to find inclusivity bugs, localize the IA faults behind them, and then fix the IA to remove the inclusivity bugs they had found. Our results showed that using Why/Where/Fix reduced the number of inclusivity bugs that OSS newcomer participants experienced by 90\%. Diverse teams have been shown to be more productive as well as more innovative. One form of diversity, cognitive diversity - differences in cognitive styles - helps generate diversity of thoughts. However, cognitive diversity is often not supported in software tools. This means that these tools are not inclusive of individuals with different cognitive styles (e.g., those who like to learn through process vs. those who learn by tinkering), which burdens these individuals with a cognitive \“tax\” each time they use the tool. In this work, we present an approach that enables software developers to: (1) evaluate their tools, especially those that are information-heavy, to find \“inclusivity bugs\”- cases where diverse cognitive styles are unsupported, (2) find where in the tool these bugs lurk, and (3) fix these bugs. Our evaluation in an open source project shows that by following this approach developers were able to reduce inclusivity bugs in their projects by 90\%.},
keywords = {Human-Computer Interaction},
pubstate = {published},
tppubtype = {inproceedings}
}
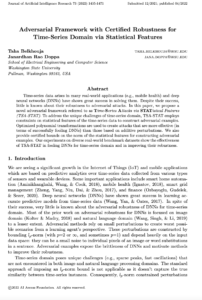
Belkhouja, Taha; Doppa, Janardhan Rao
Adversarial Framework with Certified Robustness for Time-Series Domain via Statistical Features Journal Article
In: Journal of Artificial Intelligence Research, vol. 73, pp. 1435–1471, 2022, ISSN: 1076-9757.
Abstract | Links | BibTeX | Tags: AI
@article{belkhouja_adversarial_2022,
title = {Adversarial Framework with Certified Robustness for Time-Series Domain via Statistical Features},
author = {Belkhouja, Taha and Doppa, Janardhan Rao},
url = {https://www.jair.org/index.php/jair/article/view/13543},
doi = {10.1613/jair.1.13543},
issn = {1076-9757},
year = {2022},
date = {2022-04-01},
urldate = {2022-09-01},
journal = {Journal of Artificial Intelligence Research},
volume = {73},
pages = {1435\textendash1471},
abstract = {Time-series data arises in many real-world applications (e.g., mobile health) and deep neural networks (DNNs) have shown great success in solving them. Despite their success, little is known about their robustness to adversarial attacks. In this paper, we propose a novel adversarial framework referred to as Time-Series Attacks via STATistical Features (TSA-STAT). To address the unique challenges of time-series domain, TSA-STAT employs constraints on statistical features of the time-series data to construct adversarial examples. Optimized polynomial transformations are used to create attacks that are more effective (in terms of successfully fooling DNNs) than those based on additive perturbations. We also provide certified bounds on the norm of the statistical features for constructing adversarial examples. Our experiments on diverse real-world benchmark datasets show the effectiveness of TSA-STAT in fooling DNNs for time-series domain and in improving their robustness.},
keywords = {AI},
pubstate = {published},
tppubtype = {article}
}
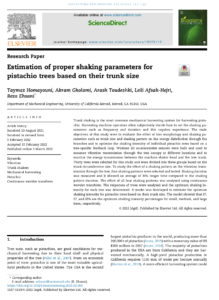
Homayouni, Taymaz; Gholami, Akram; Toudeshki, Arash; Afsah-Hejri, Leili; Ehsani, Reza
Estimation of proper shaking parameters for pistachio trees based on their trunk size Journal Article
In: Biosystems Engineering, vol. 216, pp. 121–131, 2022, ISSN: 1537-5110.
Abstract | Links | BibTeX | Tags: Labor
@article{homayouni_estimation_2022,
title = {Estimation of proper shaking parameters for pistachio trees based on their trunk size},
author = {Homayouni, Taymaz and Gholami, Akram and Toudeshki, Arash and Afsah-Hejri, Leili and Ehsani, Reza},
url = {https://www.sciencedirect.com/science/article/pii/S1537511022000411},
doi = {10.1016/j.biosystemseng.2022.02.008},
issn = {1537-5110},
year = {2022},
date = {2022-04-01},
urldate = {2022-07-07},
journal = {Biosystems Engineering},
volume = {216},
pages = {121\textendash131},
abstract = {Trunk shaking is the most common mechanical harvesting system for harvesting pistachio. Harvesting machine operators often subjectively decide how to set the shaking parameters such as frequency and duration and this requires experience. The main objectives of this study were to evaluate the effect of tree morphology and shaking parameters such as trunk size and shaking pattern on the energy distribution through the branches and to optimise the shaking intensity of individual pistachio trees based on a tree-specific feedback loop. Wireless 3D accelerometer sensors were built and used to measure vibration transmission through the tree canopy at different locations and to monitor the energy transmission between the machine shaker head and the tree trunk. Thirty trees were selected for this study and were divided into three groups based on the trunk circumference size. To study the effect of a shaking pattern on the vibration transmission through the tree, four shaking patterns were selected and tested. Shaking duration was measured and it showed an average of 30% longer time compared to the shaking pattern duration. The effect of all four shaking patterns was analysed using continuous wavelet transform. The responses of trees were analysed and the optimum shaking intensity for each tree was determined. A model was developed to estimate the optimum shaking intensity for pistachio trees based on their trunk size. The model showed that 37, 57, and 65% are the optimum shaking intensity percentages for small, medium, and large trees, respectively.},
keywords = {Labor},
pubstate = {published},
tppubtype = {article}
}
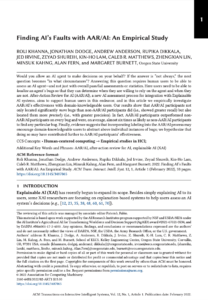
Roli Khanna; Jonathan Dodge; Andrew Anderson; Rupika Dikkala; Jed Irvine; Zeyad Shureih; Kin-Ho Lam; Caleb R. Matthews; Zhengxian Lin; Minsuk Kahng; Alan Fern; Margaret Burnett
Finding AI’s Faults with AAR/AI: An Empirical Study Journal Article
In: ACM Transactions on Interactive Intelligent Systems, vol. 12, no. 1, pp. 1:1–1:33, 2022, ISSN: 2160-6455.
Abstract | Links | BibTeX | Tags: AI, Human-Computer Interaction
@article{khanna_finding_2022,
title = {Finding AI’s Faults with AAR/AI: An Empirical Study},
author = { Roli Khanna and Jonathan Dodge and Andrew Anderson and Rupika Dikkala and Jed Irvine and Zeyad Shureih and Kin-Ho Lam and Caleb R. Matthews and Zhengxian Lin and Minsuk Kahng and Alan Fern and Margaret Burnett},
url = {https://doi.org/10.1145/3487065},
doi = {10.1145/3487065},
issn = {2160-6455},
year = {2022},
date = {2022-03-01},
urldate = {2022-03-01},
journal = {ACM Transactions on Interactive Intelligent Systems},
volume = {12},
number = {1},
pages = {1:1--1:33},
abstract = {Would you allow an AI agent to make decisions on your behalf? If the answer is “not always,” the next question becomes “in what circumstances”? Answering this question requires human users to be able to assess an AI agent\textemdashand not just with overall pass/fail assessments or statistics. Here users need to be able to localize an agent’s bugs so that they can determine when they are willing to rely on the agent and when they are not. After-Action Review for AI (AAR/AI), a new AI assessment process for integration with Explainable AI systems, aims to support human users in this endeavor, and in this article we empirically investigate AAR/AI’s effectiveness with domain-knowledgeable users. Our results show that AAR/AI participants not only located significantly more bugs than non-AAR/AI participants did (i.e., showed greater recall) but also located them more precisely (i.e., with greater precision). In fact, AAR/AI participants outperformed non-AAR/AI participants on every bug and were, on average, almost six times as likely as non-AAR/AI participants to find any particular bug. Finally, evidence suggests that incorporating labeling into the AAR/AI process may encourage domain-knowledgeable users to abstract above individual instances of bugs; we hypothesize that doing so may have contributed further to AAR/AI participants’ effectiveness.},
keywords = {AI, Human-Computer Interaction},
pubstate = {published},
tppubtype = {article}
}