2024
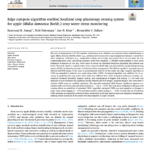
Basavaraj R. Amogi; Nisit Pukrongta; Lav R. Khot; Bernardita V. Sallato
Edge compute algorithm enabled localized crop physiology sensing system for apple (textitMalus domestica Borkh.) crop water stress monitoring Journal Article
In: Computers and Electronics in Agriculture, vol. 224, pp. 109137, 2024, ISSN: 0168-1699.
Abstract | Links | BibTeX | Tags: AI, Farm Ops
@article{amogi_edge_2024,
title = {Edge compute algorithm enabled localized crop physiology sensing system for apple (textitMalus domestica Borkh.) crop water stress monitoring},
author = {Basavaraj R. Amogi and Nisit Pukrongta and Lav R. Khot and Bernardita V. Sallato},
url = {https://www.sciencedirect.com/science/article/pii/S0168169924005283},
doi = {10.1016/j.compag.2024.109137},
issn = {0168-1699},
year = {2024},
date = {2024-09-01},
urldate = {2024-09-01},
journal = {Computers and Electronics in Agriculture},
volume = {224},
pages = {109137},
abstract = {Elevated air temperature (\>35 ℃) combined with intense solar radiation can cause heat stress related damage to apple (Malus domestica Borkh.) fruits (e.g., sunburn) and increase tree evapotranspiration demand. Current heat stress mitigation techniques (e.g., evaporative cooling and netting) may protect fruits but can skew the tree evapotranspiration rates, preventing precision under-tree irrigation. A detailed understanding of heat stress mitigation techniques on tree fruit water status is critical for optimized irrigation scheduling and reduced crop losses. This study aimed to quantify water stress using a localized edge-compute-enabled crop physiology sensing system (CPSS), developed previously for fruit heat stress management. The CPSS is capable of acquiring thermal infrared and RGB images of the scene at predetermined interval. In this study, the edge compute algorithm on CPSS was amended to estimate crop water stress index (CWSI). Developed algorithm was validated for its accuracy in predicting the crop water stress under four different heat stress mitigation techniques namely: conventional overhead sprinklers, foggers, netting, and combinations of foggers and netting. A CPSS node was deployed in each treatment for acquiring thermal infrared and RGB images. Acquired imagery data were used to estimate CWSI using the modified algorithm. The algorithm-estimated CWSI showed significant negative correlation with stem water potential measurements (r = -0.8, p \< 0.01). The heat stress mitigation techniques had varying effects on sensitivity of estimated CWSI. Algorithm estimated CWSI was most sensitive to changes in water stress under fogging (r = 0.76) and least sensitive under neeting (r = -0.65). Overall, the use of real-time CWSI estimates in conjunction with heat stress monitoring could help improve precision irrigation management, enabling timely actuation of the under tree drip irrigation in apple orchards.},
keywords = {AI, Farm Ops},
pubstate = {published},
tppubtype = {article}
}
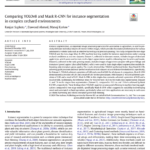
Ranjan Sapkota; Dawood Ahmed; Manoj Karkee
Comparing YOLOv8 and Mask R-CNN for instance segmentation in complex orchard environments Journal Article
In: Artificial Intelligence in Agriculture, vol. 13, pp. 84–99, 2024, ISSN: 2589-7217.
Abstract | Links | BibTeX | Tags: Artificial intelligence, Automation, Deep learning, Machine Learning, Machine vision, Mask R-CNN, Robotics, YOLOv8
@article{sapkota_comparing_2024,
title = {Comparing YOLOv8 and Mask R-CNN for instance segmentation in complex orchard environments},
author = {Ranjan Sapkota and Dawood Ahmed and Manoj Karkee},
url = {https://www.sciencedirect.com/science/article/pii/S258972172400028X},
doi = {10.1016/j.aiia.2024.07.001},
issn = {2589-7217},
year = {2024},
date = {2024-09-01},
urldate = {2024-09-01},
journal = {Artificial Intelligence in Agriculture},
volume = {13},
pages = {84\textendash99},
abstract = {Instance segmentation, an important image processing operation for automation in agriculture, is used to precisely delineate individual objects of interest within images, which provides foundational information for various automated or robotic tasks such as selective harvesting and precision pruning. This study compares the one-stage YOLOv8 and the two-stage Mask R-CNN machine learning models for instance segmentation under varying orchard conditions across two datasets. Dataset 1, collected in dormant season, includes images of dormant apple trees, which were used to train multi-object segmentation models delineating tree branches and trunks. Dataset 2, collected in the early growing season, includes images of apple tree canopies with green foliage and immature (green) apples (also called fruitlet), which were used to train single-object segmentation models delineating only immature green apples. The results showed that YOLOv8 performed better than Mask R-CNN, achieving good precision and near-perfect recall across both datasets at a confidence threshold of 0.5. Specifically, for Dataset 1, YOLOv8 achieved a precision of 0.90 and a recall of 0.95 for all classes. In comparison, Mask R-CNN demonstrated a precision of 0.81 and a recall of 0.81 for the same dataset. With Dataset 2, YOLOv8 achieved a precision of 0.93 and a recall of 0.97. Mask R-CNN, in this single-class scenario, achieved a precision of 0.85 and a recall of 0.88. Additionally, the inference times for YOLOv8 were 10.9 ms for multi-class segmentation (Dataset 1) and 7.8 ms for single-class segmentation (Dataset 2), compared to 15.6 ms and 12.8 ms achieved by Mask R-CNN's, respectively. These findings show YOLOv8's superior accuracy and efficiency in machine learning applications compared to two-stage models, specifically Mask-R-CNN, which suggests its suitability in developing smart and automated orchard operations, particularly when real-time applications are necessary in such cases as robotic harvesting and robotic immature green fruit thinning.},
keywords = {Artificial intelligence, Automation, Deep learning, Machine Learning, Machine vision, Mask R-CNN, Robotics, YOLOv8},
pubstate = {published},
tppubtype = {article}
}
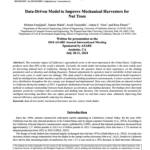
Mohsen Farajijalal, Samira Malek, Arash Toudeshki, Joshua H. Viers, Reza Ehsani
Data-Driven Model to Improve Mechanical Harvesters for Nut Trees Proceedings Article
In: ASABE, 2024.
Abstract | Links | BibTeX | Tags:
@inproceedings{noauthor_data-driven_nodateb,
title = {Data-Driven Model to Improve Mechanical Harvesters for Nut Trees},
author = {Mohsen Farajijalal, Samira Malek, Arash Toudeshki, Joshua H. Viers, Reza Ehsani},
url = {https://doi.org/10.13031/aim.202400858},
year = {2024},
date = {2024-08-02},
publisher = {ASABE},
abstract = {The economic impact of California‘s agricultural sector is the most important in the United States. California produces more than 80% of the world‘s almonds. Currently, the trunk shaker harvesting machine is the most widely used for harvesting almond trees in California. During the harvest, the operator, based on their experience, set the shaking parameters such as duration and shaking frequency. Manual adjustments by operators lead to variability in fruit removal, and in some cases, it could cause tree damage. This study aimed to develop a data-driven mathematical model required to build an intelligent tree shaker machine capable of optimizing shaking parameters autonomously. A sensor system to monitor force distribution throughout the tree canopy was designed and implemented. Data was collected from an almond orchard in California during the summer of 2023. A quadratic mathematical model was developed using machine learning regression methods to estimate relationships between trunk diameter, acceleration, and shaking duration. The findings show that trunk diameter positively correlates with acceleration and shaking time duration. Our research demonstrates the potential for intelligent harvesting machines that can adjust parameters based on real-time sensor data, ultimately improving fruit removal efficiency, and potentially reducing tree damage.},
keywords = {},
pubstate = {published},
tppubtype = {inproceedings}
}
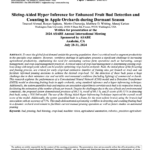
Dawood Ahmed, Ranjan Sapkota, Martin Churuvija, Matthew Whiting, Manoj Karkee
Slicing-Aided Hyper Inference for Enhanced Fruit Bud Detection and Counting in Apple Orchards during Dormant Season Proceedings Article
In: ASABE, 2024.
Abstract | Links | BibTeX | Tags:
@inproceedings{noauthor_slicing-aided_nodateb,
title = {Slicing-Aided Hyper Inference for Enhanced Fruit Bud Detection and Counting in Apple Orchards during Dormant Season},
author = {Dawood Ahmed, Ranjan Sapkota, Martin Churuvija, Matthew Whiting, Manoj Karkee},
url = {https://doi.org/10.13031/aim.202401055},
year = {2024},
date = {2024-08-02},
publisher = {ASABE},
abstract = {To meet the global food demand amidst the growing population, there‘s a critical need to augment productivity in the specialty crop industry. However, workforce shortage in agriculture presents a significant challenge to increasing agricultural productivity, emphasizing the need for automating various farm operations such as harvesting, canopy management, and crop-load management practices. A critical aspect of crop-load management is determining existing crop-load along with target yield, which can be used for optimizing crop-load in orchards. Buds, the initial phase of the flowering and fruiting process, are critical for early crop-load estimation (number of fruiting sites per branch or tree) and can facilitate informed pruning decisions to achieve the desired crop-load. Yet, the detection of these buds poses a huge challenge due to their miniature size and variable environmental conditions (including lighting) of commercial orchards. This research explores the performances of a Convolutional Neural Network (CNN) model and a Detection Transformer (DETR) model in detecting fruiting buds in apple orchards, using standard inference and a hyper inferencing technique. Additionally, the system quantifies buds and employs an association algorithm to correlate each bud to its respective branch, facilitating the estimation of the number of buds per branch. Despite the challenges due to the size of buds and environmental complexity, the proposed system demonstrated precision of 80.2 %, recall of 80.1 %, and f1-score of 80.15 % in detecting fruit buds, using a YOLOv8 model. The use of the Slicing Aided Hyper Inferencing Technique improved the overall recall score of the model. Additionally, the system quantified buds in a frame for the most salient branch using the branch-bud association with an accuracy of 73%. This study demonstrated a promising workflow in detecting and counting fruiting buds in a dynamic orchard environment to facilitate current manual pruning operation as well as future studies on automated pruning.},
keywords = {},
pubstate = {published},
tppubtype = {inproceedings}
}
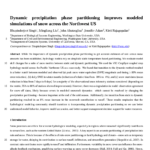
Bhupinderjeet Singh; Mingliang Liu; John Abatzoglou; Jennifer Adam; Kirti Rajagopalan
Dynamic precipitation phase partitioning improves modeled simulations of snow across the Northwest US Journal Article
In: EGUsphere, pp. 1–24, 2024, (Publisher: Copernicus GmbH).
Abstract | Links | BibTeX | Tags:
@article{singh_dynamic_2024,
title = {Dynamic precipitation phase partitioning improves modeled simulations of snow across the Northwest US},
author = {Bhupinderjeet Singh and Mingliang Liu and John Abatzoglou and Jennifer Adam and Kirti Rajagopalan},
url = {https://egusphere.copernicus.org/preprints/2024/egusphere-2024-2284/},
doi = {10.5194/egusphere-2024-2284},
year = {2024},
date = {2024-08-01},
urldate = {2024-08-01},
journal = {EGUsphere},
pages = {1\textendash24},
abstract = {\<p\>\<strong class="journal-contentHeaderColor"\>Abstract.\</strong\> While the importance of dynamic precipitation phase partitioning to get accurate estimates of rain versus snow amounts has been established, hydrology models rely on simplistic static temperature-based partitioning. We evaluate model skill changes for a suite of snow metrics between static and dynamic partitioning. We used the VIC-CropSyst coupled crop hydrology model across the Pacific Northwest US as a case study. We found that transition to the dynamic method resulted in a better match between modeled and observed (a) peak snow water equivalent (SWE) magnitude and timing (textasciitilde50 % mean error reduction), (b) daily SWE in winter months (reduction of relative bias from -30 % to -4 %), and (c) snow-start dates (mean reduction in bias from 7 days to 0 days) for a majority of the observational snow telemetry stations considered (depending on the metric, 75 % to 88 % of stations showed improvements). However, there was a degradation in model-observation agreement for snow-off dates, likely because errors in modeled snowmelt dynamics\—which cannot be resolved by changing the precipitation partitioning\—become important at the end of the cold season. Additionally, the transition from static to dynamic partitioning resulted in an 8 % mean increase in the snowmelt contribution to runoff. These results emphasize that the hydrological modeling community should transition to incorporating dynamic precipitation partitioning so we can better understand model behavior, improve model accuracies, and better support management decision support for water resources.\</p\>},
note = {Publisher: Copernicus GmbH},
keywords = {},
pubstate = {published},
tppubtype = {article}
}
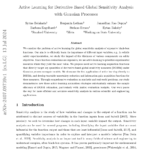
Syrine Belakaria; Benjamin Letham; Janardhan Rao Doppa; Barbara Engelhardt; Stefano Ermon; Eytan Bakshy
Active Learning for Derivative-Based Global Sensitivity Analysis with Gaussian Processes Proceedings Article
In: arXiv, 2024, (arXiv:2407.09739).
Abstract | Links | BibTeX | Tags: Computer Science - Artificial Intelligence, Computer Science - Machine Learning, Statistics - Machine Learning
@inproceedings{belakaria_active_2024,
title = {Active Learning for Derivative-Based Global Sensitivity Analysis with Gaussian Processes},
author = {Syrine Belakaria and Benjamin Letham and Janardhan Rao Doppa and Barbara Engelhardt and Stefano Ermon and Eytan Bakshy},
url = {http://arxiv.org/abs/2407.09739},
doi = {10.48550/arXiv.2407.09739},
year = {2024},
date = {2024-07-01},
urldate = {2024-07-01},
publisher = {arXiv},
abstract = {We consider the problem of active learning for global sensitivity analysis of expensive black-box functions. Our aim is to efficiently learn the importance of different input variables, e.g., in vehicle safety experimentation, we study the impact of the thickness of various components on safety objectives. Since function evaluations are expensive, we use active learning to prioritize experimental resources where they yield the most value. We propose novel active learning acquisition functions that directly target key quantities of derivative-based global sensitivity measures (DGSMs) under Gaussian process surrogate models. We showcase the first application of active learning directly to DGSMs, and develop tractable uncertainty reduction and information gain acquisition functions for these measures. Through comprehensive evaluation on synthetic and real-world problems, our study demonstrates how these active learning acquisition strategies substantially enhance the sample efficiency of DGSM estimation, particularly with limited evaluation budgets. Our work paves the way for more efficient and accurate sensitivity analysis in various scientific and engineering applications.},
note = {arXiv:2407.09739},
keywords = {Computer Science - Artificial Intelligence, Computer Science - Machine Learning, Statistics - Machine Learning},
pubstate = {published},
tppubtype = {inproceedings}
}
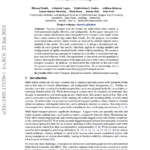
Bikram Pandit; Ashutosh Gupta; Mohitvishnu S. Gadde; Addison Johnson; Aayam Kumar Shrestha; Helei Duan; Jeremy Dao; Alan Fern
Learning Decentralized Multi-Biped Control for Payload Transport Proceedings
arXiv, 2024, (arXiv:2406.17279).
Abstract | Links | BibTeX | Tags: Computer Science - Artificial Intelligence, Computer Science - Robotics
@proceedings{pandit_learning_2024,
title = {Learning Decentralized Multi-Biped Control for Payload Transport},
author = {Bikram Pandit and Ashutosh Gupta and Mohitvishnu S. Gadde and Addison Johnson and Aayam Kumar Shrestha and Helei Duan and Jeremy Dao and Alan Fern},
url = {http://arxiv.org/abs/2406.17279},
doi = {10.48550/arXiv.2406.17279},
year = {2024},
date = {2024-06-01},
urldate = {2024-06-01},
publisher = {arXiv},
abstract = {Payload transport over flat terrain via multi-wheel robot carriers is well-understood, highly effective, and configurable. In this paper, our goal is to provide similar effectiveness and configurability for transport over rough terrain that is more suitable for legs rather than wheels. For this purpose, we consider multi-biped robot carriers, where wheels are replaced by multiple bipedal robots attached to the carrier. Our main contribution is to design a decentralized controller for such systems that can be effectively applied to varying numbers and configurations of rigidly attached bipedal robots without retraining. We present a reinforcement learning approach for training the controller in simulation that supports transfer to the real world. Our experiments in simulation provide quantitative metrics showing the effectiveness of the approach over a wide variety of simulated transport scenarios. In addition, we demonstrate the controller in the real-world for systems composed of two and three Cassie robots. To our knowledge, this is the first example of a scalable multi-biped payload transport system.},
note = {arXiv:2406.17279},
keywords = {Computer Science - Artificial Intelligence, Computer Science - Robotics},
pubstate = {published},
tppubtype = {proceedings}
}
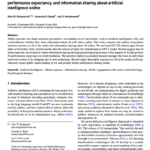
Alex W. Kirkpatrick; Amanda D. Boyd; Jay D. Hmielowski
In: AI & SOCIETY, 2024, ISSN: 1435-5655.
Abstract | Links | BibTeX | Tags: Artificial intelligence, Information sharing, Media exposure, Psychological distance, Public engagement with science and technology
@article{kirkpatrick_who_2024,
title = {Who shares about AI? Media exposure, psychological proximity, performance expectancy, and information sharing about artificial intelligence online},
author = {Alex W. Kirkpatrick and Amanda D. Boyd and Jay D. Hmielowski},
url = {https://doi.org/10.1007/s00146-024-01997-x},
doi = {10.1007/s00146-024-01997-x},
issn = {1435-5655},
year = {2024},
date = {2024-06-01},
urldate = {2024-06-01},
journal = {AI \& SOCIETY},
abstract = {Media exposure can shape audience perceptions surrounding novel innovations, such as artificial intelligence (AI), and could influence whether they share information about AI with others online. This study examines the indirect association between exposure to AI in the media and information sharing about AI online. We surveyed 567 US citizens aged 18 and older in November 2020, several months after the release of Open AI’s transformative GPT-3 model. Results suggest that AI media exposure was related to online information sharing through psychological proximity to the impacts of AI and positive AI performance expectancy in serial mediation. This positive indirect association became stronger the more an individual perceived society to be changing due to new technology. Results imply that public exposure to AI in the media could significantly impact public understanding of AI, and prompt further information sharing online.},
keywords = {Artificial intelligence, Information sharing, Media exposure, Psychological distance, Public engagement with science and technology},
pubstate = {published},
tppubtype = {article}
}
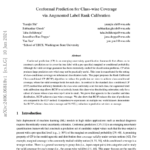
Yuanjie Shi; Subhankar Ghosh; Taha Belkhouja; Janardhan Rao Doppa; Yan Yan
Conformal Prediction for Class-wise Coverage via Augmented Label Rank Calibration Proceedings
arXiv, 2024, (arXiv:2406.06818).
Abstract | Links | BibTeX | Tags: Computer Science - Machine Learning
@proceedings{shi_conformal_2024,
title = {Conformal Prediction for Class-wise Coverage via Augmented Label Rank Calibration},
author = {Yuanjie Shi and Subhankar Ghosh and Taha Belkhouja and Janardhan Rao Doppa and Yan Yan},
url = {http://arxiv.org/abs/2406.06818},
doi = {10.48550/arXiv.2406.06818},
year = {2024},
date = {2024-06-01},
urldate = {2024-06-01},
publisher = {arXiv},
abstract = {Conformal prediction (CP) is an emerging uncertainty quantification framework that allows us to construct a prediction set to cover the true label with a pre-specified marginal or conditional probability. Although the valid coverage guarantee has been extensively studied for classification problems, CP often produces large prediction sets which may not be practically useful. This issue is exacerbated for the setting of class-conditional coverage on imbalanced classification tasks. This paper proposes the Rank Calibrated Class-conditional CP (RC3P) algorithm to reduce the prediction set sizes to achieve class-conditional coverage, where the valid coverage holds for each class. In contrast to the standard class-conditional CP (CCP) method that uniformly thresholds the class-wise conformity score for each class, the augmented label rank calibration step allows RC3P to selectively iterate this class-wise thresholding subroutine only for a subset of classes whose class-wise top-k error is small. We prove that agnostic to the classifier and data distribution, RC3P achieves class-wise coverage. We also show that RC3P reduces the size of prediction sets compared to the CCP method. Comprehensive experiments on multiple real-world datasets demonstrate that RC3P achieves class-wise coverage and 26.25% reduction in prediction set sizes on average.},
note = {arXiv:2406.06818},
keywords = {Computer Science - Machine Learning},
pubstate = {published},
tppubtype = {proceedings}
}
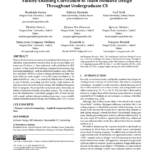
Rosalinda Garcia; Patricia Morreale; Gail Verdi; Heather Garcia; Geraldine Jimena Noa; Spencer P. Madsen; Maria Jesus Alzugaray-Orellana; Elizabeth Li; Margaret Burnett
The Matchmaker Inclusive Design Curriculum: A Faculty-Enabling Curriculum to Teach Inclusive Design Throughout Undergraduate CS Proceedings Article
In: Proceedings of the CHI Conference on Human Factors in Computing Systems, pp. 1–22, Association for Computing Machinery, New York, NY, USA, 2024, ISBN: 9798400703300.
Abstract | Links | BibTeX | Tags:
@inproceedings{garcia_matchmaker_2024,
title = {The Matchmaker Inclusive Design Curriculum: A Faculty-Enabling Curriculum to Teach Inclusive Design Throughout Undergraduate CS},
author = {Rosalinda Garcia and Patricia Morreale and Gail Verdi and Heather Garcia and Geraldine Jimena Noa and Spencer P. Madsen and Maria Jesus Alzugaray-Orellana and Elizabeth Li and Margaret Burnett},
url = {https://dl.acm.org/doi/10.1145/3613904.3642475},
doi = {10.1145/3613904.3642475},
isbn = {9798400703300},
year = {2024},
date = {2024-05-01},
urldate = {2024-05-01},
booktitle = {Proceedings of the CHI Conference on Human Factors in Computing Systems},
pages = {1\textendash22},
publisher = {Association for Computing Machinery},
address = {New York, NY, USA},
series = {CHI '24},
abstract = {Despite efforts to raise awareness of societal and ethical issues in CS education, research shows students often do not act upon their new awareness (Problem 1). One such issue, well-established by HCI research, is that much of technology contains barriers impacting numerous populations\textemdashsuch as minoritized genders, races, ethnicities, and more. HCI has inclusive design methods that help\textemdashbut these skills are rarely taught, even in HCI classes (Problem 2). To address Problems 1 and 2, we created the Matchmaker Curriculum to pair CS faculty\textemdashincluding non-HCI faculty\textemdashwith inclusive design elements to allow for inclusive design skill-building throughout their CS program. We present the curriculum and a field study, in which we followed 18 faculty along their journey. The results show how the Matchmaker Curriculum equipped 88% of these faculty with enough inclusive design teaching knowledge to successfully embed actionable inclusive design skill-building into 13 CS courses.},
keywords = {},
pubstate = {published},
tppubtype = {inproceedings}
}