2024
1.
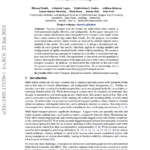
Bikram Pandit; Ashutosh Gupta; Mohitvishnu S. Gadde; Addison Johnson; Aayam Kumar Shrestha; Helei Duan; Jeremy Dao; Alan Fern
Learning Decentralized Multi-Biped Control for Payload Transport Proceedings
arXiv, 2024, (arXiv:2406.17279).
Abstract | Links | BibTeX | Tags: Computer Science - Artificial Intelligence, Computer Science - Robotics
@proceedings{pandit_learning_2024,
title = {Learning Decentralized Multi-Biped Control for Payload Transport},
author = {Bikram Pandit and Ashutosh Gupta and Mohitvishnu S. Gadde and Addison Johnson and Aayam Kumar Shrestha and Helei Duan and Jeremy Dao and Alan Fern},
url = {http://arxiv.org/abs/2406.17279},
doi = {10.48550/arXiv.2406.17279},
year = {2024},
date = {2024-06-01},
urldate = {2024-06-01},
publisher = {arXiv},
abstract = {Payload transport over flat terrain via multi-wheel robot carriers is well-understood, highly effective, and configurable. In this paper, our goal is to provide similar effectiveness and configurability for transport over rough terrain that is more suitable for legs rather than wheels. For this purpose, we consider multi-biped robot carriers, where wheels are replaced by multiple bipedal robots attached to the carrier. Our main contribution is to design a decentralized controller for such systems that can be effectively applied to varying numbers and configurations of rigidly attached bipedal robots without retraining. We present a reinforcement learning approach for training the controller in simulation that supports transfer to the real world. Our experiments in simulation provide quantitative metrics showing the effectiveness of the approach over a wide variety of simulated transport scenarios. In addition, we demonstrate the controller in the real-world for systems composed of two and three Cassie robots. To our knowledge, this is the first example of a scalable multi-biped payload transport system.},
note = {arXiv:2406.17279},
keywords = {Computer Science - Artificial Intelligence, Computer Science - Robotics},
pubstate = {published},
tppubtype = {proceedings}
}
Payload transport over flat terrain via multi-wheel robot carriers is well-understood, highly effective, and configurable. In this paper, our goal is to provide similar effectiveness and configurability for transport over rough terrain that is more suitable for legs rather than wheels. For this purpose, we consider multi-biped robot carriers, where wheels are replaced by multiple bipedal robots attached to the carrier. Our main contribution is to design a decentralized controller for such systems that can be effectively applied to varying numbers and configurations of rigidly attached bipedal robots without retraining. We present a reinforcement learning approach for training the controller in simulation that supports transfer to the real world. Our experiments in simulation provide quantitative metrics showing the effectiveness of the approach over a wide variety of simulated transport scenarios. In addition, we demonstrate the controller in the real-world for systems composed of two and three Cassie robots. To our knowledge, this is the first example of a scalable multi-biped payload transport system.
2.
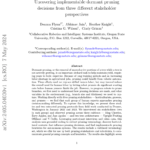
Deanna Flynn; Abhinav Jain; Heather Knight; Cristina G. Wilson; Cindy Grimm
Uncovering implementable dormant pruning decisions from three different stakeholder perspectives Miscellaneous
2024, (arXiv:2405.04030 [cs]).
Abstract | Links | BibTeX | Tags: Computer Science - Robotics
@misc{flynn_uncovering_2024,
title = {Uncovering implementable dormant pruning decisions from three different stakeholder perspectives},
author = {Deanna Flynn and Abhinav Jain and Heather Knight and Cristina G. Wilson and Cindy Grimm},
url = {http://arxiv.org/abs/2405.04030},
doi = {10.48550/arXiv.2405.04030},
year = {2024},
date = {2024-05-01},
urldate = {2024-05-01},
publisher = {arXiv},
abstract = {Dormant pruning, or the removal of unproductive portions of a tree while a tree is not actively growing, is an important orchard task to help maintain yield, requiring years to build expertise. Because of long training periods and an increasing labor shortage in agricultural jobs, pruning could benefit from robotic automation. However, to program robots to prune branches, we first need to understand how pruning decisions are made, and what variables in the environment (e.g., branch size and thickness) we need to capture. Working directly with three pruning stakeholders \textendash horticulturists, growers, and pruners \textendash we find that each group of human experts approaches pruning decision-making differently. To capture this knowledge, we present three studies and two extracted pruning protocols from field work conducted in Prosser, Washington in January 2022 and 2023. We interviewed six stakeholders (two in each group) and observed pruning across three cultivars \textendash Bing Cherries, Envy Apples, and Jazz Apples \textendash and two tree architectures \textendash Upright Fruiting Offshoot and V-Trellis. Leveraging participant interviews and video data, this analysis uses grounded coding to extract pruning terminology, discover horticultural contexts that influence pruning decisions, and find implementable pruning heuristics for autonomous systems. The results include a validated terminology set, which we offer for use by both pruning stakeholders and roboticists, to communicate general pruning concepts and heuristics. The results also highlight seven pruning heuristics utilizing this terminology set that would be relevant for use by future autonomous robot pruning systems, and characterize three discovered horticultural contexts (i.e., environmental management, crop-load management, and replacement wood) across all three cultivars.},
note = {arXiv:2405.04030 [cs]},
keywords = {Computer Science - Robotics},
pubstate = {published},
tppubtype = {misc}
}
Dormant pruning, or the removal of unproductive portions of a tree while a tree is not actively growing, is an important orchard task to help maintain yield, requiring years to build expertise. Because of long training periods and an increasing labor shortage in agricultural jobs, pruning could benefit from robotic automation. However, to program robots to prune branches, we first need to understand how pruning decisions are made, and what variables in the environment (e.g., branch size and thickness) we need to capture. Working directly with three pruning stakeholders – horticulturists, growers, and pruners – we find that each group of human experts approaches pruning decision-making differently. To capture this knowledge, we present three studies and two extracted pruning protocols from field work conducted in Prosser, Washington in January 2022 and 2023. We interviewed six stakeholders (two in each group) and observed pruning across three cultivars – Bing Cherries, Envy Apples, and Jazz Apples – and two tree architectures – Upright Fruiting Offshoot and V-Trellis. Leveraging participant interviews and video data, this analysis uses grounded coding to extract pruning terminology, discover horticultural contexts that influence pruning decisions, and find implementable pruning heuristics for autonomous systems. The results include a validated terminology set, which we offer for use by both pruning stakeholders and roboticists, to communicate general pruning concepts and heuristics. The results also highlight seven pruning heuristics utilizing this terminology set that would be relevant for use by future autonomous robot pruning systems, and characterize three discovered horticultural contexts (i.e., environmental management, crop-load management, and replacement wood) across all three cultivars.