2024
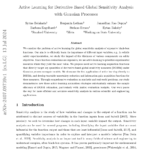
Syrine Belakaria; Benjamin Letham; Janardhan Rao Doppa; Barbara Engelhardt; Stefano Ermon; Eytan Bakshy
Active Learning for Derivative-Based Global Sensitivity Analysis with Gaussian Processes Proceedings Article
In: arXiv, 2024, (arXiv:2407.09739).
Abstract | Links | BibTeX | Tags: Computer Science - Artificial Intelligence, Computer Science - Machine Learning, Statistics - Machine Learning
@inproceedings{belakaria_active_2024,
title = {Active Learning for Derivative-Based Global Sensitivity Analysis with Gaussian Processes},
author = {Syrine Belakaria and Benjamin Letham and Janardhan Rao Doppa and Barbara Engelhardt and Stefano Ermon and Eytan Bakshy},
url = {http://arxiv.org/abs/2407.09739},
doi = {10.48550/arXiv.2407.09739},
year = {2024},
date = {2024-07-01},
urldate = {2024-07-01},
publisher = {arXiv},
abstract = {We consider the problem of active learning for global sensitivity analysis of expensive black-box functions. Our aim is to efficiently learn the importance of different input variables, e.g., in vehicle safety experimentation, we study the impact of the thickness of various components on safety objectives. Since function evaluations are expensive, we use active learning to prioritize experimental resources where they yield the most value. We propose novel active learning acquisition functions that directly target key quantities of derivative-based global sensitivity measures (DGSMs) under Gaussian process surrogate models. We showcase the first application of active learning directly to DGSMs, and develop tractable uncertainty reduction and information gain acquisition functions for these measures. Through comprehensive evaluation on synthetic and real-world problems, our study demonstrates how these active learning acquisition strategies substantially enhance the sample efficiency of DGSM estimation, particularly with limited evaluation budgets. Our work paves the way for more efficient and accurate sensitivity analysis in various scientific and engineering applications.},
note = {arXiv:2407.09739},
keywords = {Computer Science - Artificial Intelligence, Computer Science - Machine Learning, Statistics - Machine Learning},
pubstate = {published},
tppubtype = {inproceedings}
}
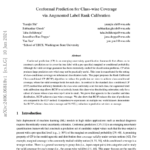
Yuanjie Shi; Subhankar Ghosh; Taha Belkhouja; Janardhan Rao Doppa; Yan Yan
Conformal Prediction for Class-wise Coverage via Augmented Label Rank Calibration Proceedings
arXiv, 2024, (arXiv:2406.06818).
Abstract | Links | BibTeX | Tags: Computer Science - Machine Learning
@proceedings{shi_conformal_2024,
title = {Conformal Prediction for Class-wise Coverage via Augmented Label Rank Calibration},
author = {Yuanjie Shi and Subhankar Ghosh and Taha Belkhouja and Janardhan Rao Doppa and Yan Yan},
url = {http://arxiv.org/abs/2406.06818},
doi = {10.48550/arXiv.2406.06818},
year = {2024},
date = {2024-06-01},
urldate = {2024-06-01},
publisher = {arXiv},
abstract = {Conformal prediction (CP) is an emerging uncertainty quantification framework that allows us to construct a prediction set to cover the true label with a pre-specified marginal or conditional probability. Although the valid coverage guarantee has been extensively studied for classification problems, CP often produces large prediction sets which may not be practically useful. This issue is exacerbated for the setting of class-conditional coverage on imbalanced classification tasks. This paper proposes the Rank Calibrated Class-conditional CP (RC3P) algorithm to reduce the prediction set sizes to achieve class-conditional coverage, where the valid coverage holds for each class. In contrast to the standard class-conditional CP (CCP) method that uniformly thresholds the class-wise conformity score for each class, the augmented label rank calibration step allows RC3P to selectively iterate this class-wise thresholding subroutine only for a subset of classes whose class-wise top-k error is small. We prove that agnostic to the classifier and data distribution, RC3P achieves class-wise coverage. We also show that RC3P reduces the size of prediction sets compared to the CCP method. Comprehensive experiments on multiple real-world datasets demonstrate that RC3P achieves class-wise coverage and 26.25% reduction in prediction set sizes on average.},
note = {arXiv:2406.06818},
keywords = {Computer Science - Machine Learning},
pubstate = {published},
tppubtype = {proceedings}
}
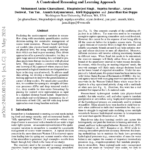
Mohammed Amine Gharsallaoui; Bhupinderjeet Singh; Supriya Savalkar; Aryan Deshwal; Yan Yan; Ananth Kalyanaraman; Kirti Rajagopalan; Janardhan Rao Doppa
Streamflow Prediction with Uncertainty Quantification for Water Management: A Constrained Reasoning and Learning Approach Miscellaneous
2024, (arXiv:2406.00133 [cs]).
Abstract | Links | BibTeX | Tags: Computer Science - Artificial Intelligence, Computer Science - Machine Learning
@misc{gharsallaoui_streamflow_2024,
title = {Streamflow Prediction with Uncertainty Quantification for Water Management: A Constrained Reasoning and Learning Approach},
author = {Mohammed Amine Gharsallaoui and Bhupinderjeet Singh and Supriya Savalkar and Aryan Deshwal and Yan Yan and Ananth Kalyanaraman and Kirti Rajagopalan and Janardhan Rao Doppa},
url = {http://arxiv.org/abs/2406.00133},
doi = {10.48550/arXiv.2406.00133},
year = {2024},
date = {2024-05-01},
urldate = {2024-05-01},
publisher = {arXiv},
abstract = {Predicting the spatiotemporal variation in streamflow along with uncertainty quantification enables decision-making for sustainable management of scarce water resources. Process-based hydrological models (aka physics-based models) are based on physical laws, but using simplifying assumptions which can lead to poor accuracy. Data-driven approaches offer a powerful alternative, but they require large amount of training data and tend to produce predictions that are inconsistent with physical laws. This paper studies a constrained reasoning and learning (CRL) approach where physical laws represented as logical constraints are integrated as a layer in the deep neural network. To address small data setting, we develop a theoretically-grounded training approach to improve the generalization accuracy of deep models. For uncertainty quantification, we combine the synergistic strengths of Gaussian processes (GPs) and deep temporal models (i.e., deep models for time-series forecasting) by passing the learned latent representation as input to a standard distance-based kernel. Experiments on multiple real-world datasets demonstrate the effectiveness of both CRL and GP with deep kernel approaches over strong baseline methods.},
note = {arXiv:2406.00133 [cs]},
keywords = {Computer Science - Artificial Intelligence, Computer Science - Machine Learning},
pubstate = {published},
tppubtype = {misc}
}