2024
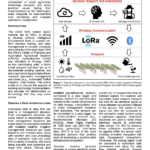
Mark Jacob Schrader; Lav R Khot
Smart Vineyard Concepts to Reality in Washington State Booklet
Viticulture and Enology Extension News, 2024.
@booklet{schrader_smart_nodate,
title = {Smart Vineyard Concepts to Reality in Washington State},
author = {Mark Jacob Schrader and Lav R Khot},
url = {https://s3-us-west-2.amazonaws.com/sites.cahnrs.wsu.edu/wp-content/uploads/sites/66/2024/04/19133949/2024-SpringVEEN-FINAL.pdf},
year = {2024},
date = {2024-04-25},
address = {Viticulture and Enology Extension News},
month = {04},
keywords = {},
pubstate = {published},
tppubtype = {booklet}
}
Uddhav Bhattarai; Qin Zhang; Manoj Karkee
Design, Integration, and Field Evaluation of a Robotic Blossom Thinning System for Tree Fruit Crops Journal Article
In: Field Robotics, 2024, (arXiv:2304.04919 [cs]).
Abstract | Links | BibTeX | Tags: AI, Humans, Labor, Thinning
@article{bhattarai_design_2023,
title = {Design, Integration, and Field Evaluation of a Robotic Blossom Thinning System for Tree Fruit Crops},
author = {Uddhav Bhattarai and Qin Zhang and Manoj Karkee},
url = {http://arxiv.org/abs/2304.04919},
doi = {10.48550/arXiv.2304.04919},
year = {2024},
date = {2024-03-07},
urldate = {2024-03-07},
journal = {Field Robotics},
publisher = {arXiv},
abstract = {The US apple industry relies heavily on semi-skilled manual labor force for essential field operations such as training, pruning, blossom and green fruit thinning, and harvesting. Blossom thinning is one of the crucial crop load management practices to achieve desired crop load, fruit quality, and return bloom. While several techniques such as chemical, and mechanical thinning are available for large-scale blossom thinning such approaches often yield unpredictable thinning results and may cause damage the canopy, spurs, and leaf tissue. Hence, growers still depend on laborious, labor intensive and expensive manual hand blossom thinning for desired thinning outcomes. This research presents a robotic solution for blossom thinning in apple orchards using a computer vision system with artificial intelligence, a six degrees of freedom robotic manipulator, and an electrically actuated miniature end-effector for robotic blossom thinning. The integrated robotic system was evaluated in a commercial apple orchard which showed promising results for targeted and selective blossom thinning. Two thinning approaches, center and boundary thinning, were investigated to evaluate the system ability to remove varying proportion of flowers from apple flower clusters. During boundary thinning the end effector was actuated around the cluster boundary while center thinning involved end-effector actuation only at the cluster centroid for a fixed duration of 2 seconds. The boundary thinning approach thinned 67.2% of flowers from the targeted clusters with a cycle time of 9.0 seconds per cluster, whereas center thinning approach thinned 59.4% of flowers with a cycle time of 7.2 seconds per cluster. When commercially adopted, the proposed system could help address problems faced by apple growers with current hand, chemical, and mechanical blossom thinning approaches.},
note = {arXiv:2304.04919 [cs]},
keywords = {AI, Humans, Labor, Thinning},
pubstate = {published},
tppubtype = {article}
}
Krishu K. Thapa; Bhupinderjeet Singh; Supriya Savalkar; Alan Fern; Kirti Rajagopalan; Ananth Kalyanaraman
Attention-based Models for Snow-Water Equivalent Prediction Proceedings Article
In: Thirty-Sixth Annual Conference on Innovative Applications of Artificial Intelligence (IAAI-24), 2024, (arXiv:2311.03388 [physics]).
Abstract | Links | BibTeX | Tags: AI, Snow Water Equivalent, Water
@inproceedings{thapa_attention-based_2023,
title = {Attention-based Models for Snow-Water Equivalent Prediction},
author = {Krishu K. Thapa and Bhupinderjeet Singh and Supriya Savalkar and Alan Fern and Kirti Rajagopalan and Ananth Kalyanaraman},
url = {http://arxiv.org/abs/2311.03388},
doi = {10.48550/arXiv.2311.03388},
year = {2024},
date = {2024-02-20},
urldate = {2024-02-20},
publisher = {Thirty-Sixth Annual Conference on Innovative Applications of Artificial Intelligence (IAAI-24)},
abstract = {Snow Water-Equivalent (SWE) \textendash the amount of water available if snowpack is melted \textendash is a key decision variable used by water management agencies to make irrigation, flood control, power generation and drought management decisions. SWE values vary spatiotemporally \textendash affected by weather, topography and other environmental factors. While daily SWE can be measured by Snow Telemetry (SNOTEL) stations with requisite instrumentation, such stations are spatially sparse requiring interpolation techniques to create spatiotemporally complete data. While recent efforts have explored machine learning (ML) for SWE prediction, a number of recent ML advances have yet to be considered. The main contribution of this paper is to explore one such ML advance, attention mechanisms, for SWE prediction. Our hypothesis is that attention has a unique ability to capture and exploit correlations that may exist across locations or the temporal spectrum (or both). We present a generic attention-based modeling framework for SWE prediction and adapt it to capture spatial attention and temporal attention. Our experimental results on 323 SNOTEL stations in the Western U.S. demonstrate that our attention-based models outperform other machine learning approaches. We also provide key results highlighting the differences between spatial and temporal attention in this context and a roadmap toward deployment for generating spatially-complete SWE maps.},
note = {arXiv:2311.03388 [physics]},
keywords = {AI, Snow Water Equivalent, Water},
pubstate = {published},
tppubtype = {inproceedings}
}
Alan Fern; Margaret Burnett; Joseph Davidson; Janardhan Rao Doppa; Paola Pesantez-Cabrera; Ananth Kalyanaraman
AgAID Institute—AI for agricultural labor and decision support Journal Article
In: AI Magazine, vol. n/a, no. n/a, 2024, ISSN: 2371-9621, (_eprint: https://onlinelibrary.wiley.com/doi/pdf/10.1002/aaai.12156).
Abstract | Links | BibTeX | Tags: AI, Farm Ops, Humans, Labor, Water
@article{fern_agaid_nodate,
title = {AgAID Institute\textemdashAI for agricultural labor and decision support},
author = {Alan Fern and Margaret Burnett and Joseph Davidson and Janardhan Rao Doppa and Paola Pesantez-Cabrera and Ananth Kalyanaraman},
url = {https://onlinelibrary.wiley.com/doi/abs/10.1002/aaai.12156},
doi = {10.1002/aaai.12156},
issn = {2371-9621},
year = {2024},
date = {2024-02-16},
urldate = {2024-02-16},
journal = {AI Magazine},
volume = {n/a},
number = {n/a},
abstract = {The AgAID Institute is a National AI Research Institute focused on developing AI solutions for specialty crop agriculture. Specialty crops include a variety of fruits and vegetables, nut trees, grapes, berries, and different types of horticultural crops. In the United States, the specialty crop industry accounts for a multibillion dollar industry with over 300 crops grown just along the U.S. west coast. Specialty crop agriculture presents several unique challenges: they are labor-intensive, are easily impacted by weather extremities, and are grown mostly on irrigated lands and hence are dependent on water. The AgAID Institute aims to develop AI solutions to address these challenges, particularly in the face of workforce shortages, water scarcity, and extreme weather events. Addressing this host of challenges requires advancing foundational AI research, including spatio-temporal system modeling, robot sensing and control, multiscale site-specific decision support, and designing effective human\textendashAI workflows. This article provides examples of current AgAID efforts and points to open directions to be explored.},
note = {_eprint: https://onlinelibrary.wiley.com/doi/pdf/10.1002/aaai.12156},
keywords = {AI, Farm Ops, Humans, Labor, Water},
pubstate = {published},
tppubtype = {article}
}
Ranjan Sapkota; Dawood Ahmed; Martin Churuvija; Manoj Karkee
Immature Green Apple Detection and Sizing in Commercial Orchards using YOLOv8 and Shape Fitting Techniques Journal Article
In: IEEE Access, pp. 1–1, 2024, ISSN: 2169-3536, (Conference Name: IEEE Access).
Abstract | Links | BibTeX | Tags: AI, Labor
@article{sapkota_immature_2024,
title = {Immature Green Apple Detection and Sizing in Commercial Orchards using YOLOv8 and Shape Fitting Techniques},
author = {Ranjan Sapkota and Dawood Ahmed and Martin Churuvija and Manoj Karkee},
url = {https://ieeexplore.ieee.org/document/10474021},
doi = {10.1109/ACCESS.2024.3378261},
issn = {2169-3536},
year = {2024},
date = {2024-01-01},
urldate = {2024-01-01},
journal = {IEEE Access},
pages = {1\textendash1},
abstract = {Detecting and estimating size of apples during the early stages of growth is crucial for predicting yield, pest management, and making informed decisions related to crop-load management, harvest and post-harvest logistics, and marketing. Traditional fruit size measurement methods are laborious and time-consuming. This study employs the state-of-the-art YOLOv8 object detection and instance segmentation algorithm in conjunction with geometric shape fitting techniques on 3D point cloud data to accurately determine the size of immature green apples (or fruitlet) in a commercial orchard environment. The methodology utilized two RGB-D sensors: Intel RealSense D435i and Microsoft Azure Kinect DK. Notably, the YOLOv8 instance segmentation models exhibited proficiency in immature green apple detection, with the YOLOv8m-seg model achieving the highest [email protected] and [email protected] scores of 0.94 and 0.91, respectively. Using the ellipsoid fitting technique on images from the Azure Kinect, we achieved an RMSE of 2.35 mm, MAE of 1.66 mm, MAPE of 6.15 mm, and an R-squared value of 0.9 in estimating the size of apple fruitlets. Challenges such as partial occlusion caused some error in accurately delineating and sizing green apples using the YOLOv8-based segmentation technique, particularly in fruit clusters. In a comparison with 102 outdoor samples, the size estimation technique performed better on the images acquired with Microsoft Azure Kinect than the same with Intel Realsense D435i. This superiority is evident from the metrics: the RMSE values (2.35 mm for Azure Kinect vs. 9.65 mm for Realsense D435i), MAE values (1.66 mm for Azure Kinect vs. 7.8 mm for Realsense D435i), and the R-squared values (0.9 for Azure Kinect vs. 0.77 for Realsense D435i). This study demonstrated the feasibility of accurately sizing immature green fruit in early growth stages using the combined 3D sensing and shape-fitting technique, which shows promise for improved precision agricultural operations such as optimal crop-load management in orchards.},
note = {Conference Name: IEEE Access},
keywords = {AI, Labor},
pubstate = {published},
tppubtype = {article}
}
Kelley Moyers; John T. Abatzoglou; Alvar Escriva-Bou; Josué Medellín-Azuara; Joshua H. Viers
In: PLOS Water, vol. 3, no. 3, pp. 1-17, 2024.
Abstract | Links | BibTeX | Tags: Water
@article{10.1371/journal.pwat.0000184,
title = {An invisible water surcharge: Climate warming increases crop water demand in the San Joaquin Valley’s groundwater-dependent irrigated agriculture},
author = {Kelley Moyers and John T. Abatzoglou and Alvar Escriva-Bou and Josu\'{e} Medell\'{i}n-Azuara and Joshua H. Viers},
url = {https://doi.org/10.1371/journal.pwat.0000184},
doi = {10.1371/journal.pwat.0000184},
year = {2024},
date = {2024-01-01},
urldate = {2024-01-01},
journal = {PLOS Water},
volume = {3},
number = {3},
pages = {1-17},
publisher = {Public Library of Science},
abstract = {California’s bountiful San Joaquin Valley (SJV), a critical region for global fruit and nut production, has withstood two severe, multi-year droughts in the past decade, exacerbated by record-breaking high temperature and evaporative demand. We employed climate data and crop coefficients to estimate the crop water demand in the SJV over the past forty years. Our approach, using crop coefficients for Penman-Montieth modeled evapotranspiration, focused on the climate effects on crop water demand, avoiding the confounding factors of changing land use and management practices that are present in actual evapotranspiration. We demonstrate that increases in crop water demand explain half of the cumulative deficits of the agricultural water balance since 1980, exacerbating water reliance on depleting groundwater supplies and fluctuating surface water imports. We call this phenomenon of climate-induced increased crop water demand an invisible water surcharge. We found that in the past decade, this invisible water surcharge on agriculture has increased the crop water demand in the SJV by 4.4% with respect to the 1980\textendash2011 timeframe\textemdashmore than 800 GL per year, a volume as large as a major reservoir in the SJV. Despite potential agronomic adaptation and crop response to climate warming, increased crop water demand adds a stressor to the sustainability of the global fruit and nut supply and calls for changes in management and policies to consider the shifting hydroclimate.},
keywords = {Water},
pubstate = {published},
tppubtype = {article}
}
2023
Jing Wang; Tyler Hallman; Laurel Hopkins; John Burns Kilbride; W. Douglas Robinson; Rebecca Hutchinson
Model Evaluation for Geospatial Problems Proceedings Article
In: 2023.
Abstract | Links | BibTeX | Tags: AI, Farm Ops
@inproceedings{wang_model_2023,
title = {Model Evaluation for Geospatial Problems},
author = {Jing Wang and Tyler Hallman and Laurel Hopkins and John Burns Kilbride and W. Douglas Robinson and Rebecca Hutchinson},
url = {https://openreview.net/forum?id=z5dAdYOgbs\&referrer=%5Bthe%20profile%20of%20Jing%20Wang%5D(%2Fprofile%3Fid%3D~Jing_Wang38)},
year = {2023},
date = {2023-12-01},
urldate = {2023-12-01},
abstract = {Geospatial problems often involve spatial autocorrelation and covariate shift, which violate the independent, identically distributed assumption underlying standard cross-validation. In this work, we establish a theoretical criterion for unbiased cross-validation, introduce a preliminary categorization framework to guide practitioners in choosing suitable cross-validation strategies for geospatial problems, reconcile conflicting recommendations on best practices, and develop a novel, straightforward method with both theoretical guarantees and empirical success.},
keywords = {AI, Farm Ops},
pubstate = {published},
tppubtype = {inproceedings}
}
Nelson D. Goosman; Basavaraj R. Amogi; Lav R. Khot
Apple fruit surface temperature prediction using weather data-driven machine learning models Proceedings Article
In: 2023 IEEE International Workshop on Metrology for Agriculture and Forestry (MetroAgriFor), pp. 429-433, 2023.
Links | BibTeX | Tags: Fruit Surface Temperature, Heat Stress
@inproceedings{10424249,
title = {Apple fruit surface temperature prediction using weather data-driven machine learning models},
author = {Nelson D. Goosman and Basavaraj R. Amogi and Lav R. Khot},
url = {https://ieeexplore.ieee.org/document/10424249},
doi = {10.1109/MetroAgriFor58484.2023.10424249},
year = {2023},
date = {2023-11-08},
urldate = {2023-11-08},
booktitle = {2023 IEEE International Workshop on Metrology for Agriculture and Forestry (MetroAgriFor)},
pages = {429-433},
keywords = {Fruit Surface Temperature, Heat Stress},
pubstate = {published},
tppubtype = {inproceedings}
}
Harrison, Galen; Alabsi Aljundi, Amro; Chen, Jiangzhuo; Ravi, S.S.; Vullikanti, Anil Kumar; Marathe, Madhav V.; Adiga, Abhijin
Identifying Complicated Contagion Scenarios from Cascade Data Proceedings Article
In: Proceedings of the 29th ACM SIGKDD Conference on Knowledge Discovery and Data Mining, pp. 4135–4145, Association for Computing Machinery, New York, NY, USA, 2023, ISBN: 9798400701030.
Abstract | Links | BibTeX | Tags: AI
@inproceedings{harrison_identifying_2023,
title = {Identifying Complicated Contagion Scenarios from Cascade Data},
author = {Harrison, Galen and Alabsi Aljundi, Amro and Chen, Jiangzhuo and Ravi, S.S. and Vullikanti, Anil Kumar and Marathe, Madhav V. and Adiga, Abhijin},
url = {https://dl.acm.org/doi/10.1145/3580305.3599841},
doi = {10.1145/3580305.3599841},
isbn = {9798400701030},
year = {2023},
date = {2023-08-01},
urldate = {2023-08-01},
booktitle = {Proceedings of the 29th ACM SIGKDD Conference on Knowledge Discovery and Data Mining},
pages = {4135\textendash4145},
publisher = {Association for Computing Machinery},
address = {New York, NY, USA},
series = {KDD '23},
abstract = {We consider the setting of cascades that result from contagion dynamics on large realistic contact networks. We address the question of whether the structural properties of a (partially) observed cascade can characterize the contagion scenario and identify the interventions that might be in effect. Using epidemic spread as a concrete example, we study how social interventions such as compliance in social distancing, extent (and efficacy) of vaccination, and the transmissibility of disease can be inferred. The techniques developed are more generally applicable to other contagions as well. Our approach involves the use of large realistic social contact networks of certain regions of USA and an agent-based model (ABM) to simulate spread under two interventions, namely vaccination and generic social distancing (GSD). Through a machine learning approach, coupled with parameter significance analysis, our experimental results show that subgraph counts of the graph induced by the cascade can be used effectively to characterize the contagion scenario even during the initial stages of the epidemic, when traditional information such as case counts alone are not adequate for this task. Further, we show that our approach performs well even for partially observed cascades. These results demonstrate that cascade data collected from digital tracing applications under poor digital penetration and privacy constraints can provide valuable information about the contagion scenario.},
keywords = {AI},
pubstate = {published},
tppubtype = {inproceedings}
}
Belkhouja, Taha; Doppa, Janardhan Rao
Adversarial Framework with Certified Robustness for Time-Series Domain via Statistical Features (Extended Abstract) Proceedings Article
In: pp. 6845–6850, 2023, (ISSN: 1045-0823).
Abstract | Links | BibTeX | Tags: AI
@inproceedings{belkhouja_adversarial_2023,
title = {Adversarial Framework with Certified Robustness for Time-Series Domain via Statistical Features (Extended Abstract)},
author = {Belkhouja, Taha and Doppa, Janardhan Rao},
url = {https://www.ijcai.org/proceedings/2023/767},
doi = {10.24963/ijcai.2023/767},
year = {2023},
date = {2023-08-01},
urldate = {2023-08-01},
volume = {6},
pages = {6845\textendash6850},
abstract = {Electronic proceedings of IJCAI 2023},
note = {ISSN: 1045-0823},
keywords = {AI},
pubstate = {published},
tppubtype = {inproceedings}
}