2025
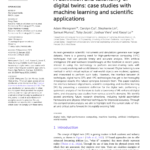
Adam Weingram; Carolyn Cui; Stephanie Lin; Samuel Munoz; Toby Jacob; Joshua Viers; Xiaoyi Lu
A definition and taxonomy of digital twins: case studies with machine learning and scientific applications Journal Article
In: Frontiers in High Performance Computing, vol. 3, 2025, ISSN: 2813-7337, (Publisher: Frontiers).
@article{weingram_definition_2025,
title = {A definition and taxonomy of digital twins: case studies with machine learning and scientific applications},
author = {Adam Weingram and Carolyn Cui and Stephanie Lin and Samuel Munoz and Toby Jacob and Joshua Viers and Xiaoyi Lu},
url = {https://www.frontiersin.org/journals/high-performance-computing/articles/10.3389/fhpcp.2025.1536501/full},
doi = {10.3389/fhpcp.2025.1536501},
issn = {2813-7337},
year = {2025},
date = {2025-03-01},
urldate = {2025-03-01},
journal = {Frontiers in High Performance Computing},
volume = {3},
note = {Publisher: Frontiers},
keywords = {},
pubstate = {published},
tppubtype = {article}
}
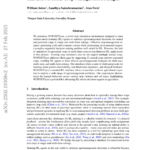
William Solow; Sandhya Saisubramanian; Alan Fern
WOFOSTGym: A Crop Simulator for Learning Annual and Perennial Crop Management Strategies Miscellaneous
2025, (arXiv:2502.19308 [cs]).
Abstract | Links | BibTeX | Tags:
@misc{solow_wofostgym_2025,
title = {WOFOSTGym: A Crop Simulator for Learning Annual and Perennial Crop Management Strategies},
author = {William Solow and Sandhya Saisubramanian and Alan Fern},
url = {http://arxiv.org/abs/2502.19308},
doi = {10.48550/arXiv.2502.19308},
year = {2025},
date = {2025-02-01},
urldate = {2025-02-01},
publisher = {arXiv},
abstract = {We introduce WOFOSTGym, a novel crop simulation environment designed to train reinforcement learning (RL) agents to optimize agromanagement decisions for annual and perennial crops in single and multi-farm settings. Effective crop management requires optimizing yield and economic returns while minimizing environmental impact, a complex sequential decision-making problem well suited for RL. However, the lack of simulators for perennial crops in multi-farm contexts has hindered RL applications in this domain. Existing crop simulators also do not support multiple annual crops. WOFOSTGym addresses these gaps by supporting 23 annual crops and two perennial crops, enabling RL agents to learn diverse agromanagement strategies in multi-year, multi-crop, and multi-farm settings. Our simulator offers a suite of challenging tasks for learning under partial observability, non-Markovian dynamics, and delayed feedback. WOFOSTGym's standard RL interface allows researchers without agricultural expertise to explore a wide range of agromanagement problems. Our experiments demonstrate the learned behaviors across various crop varieties and soil types, highlighting WOFOSTGym's potential for advancing RL-driven decision support in agriculture.},
note = {arXiv:2502.19308 [cs]},
keywords = {},
pubstate = {published},
tppubtype = {misc}
}
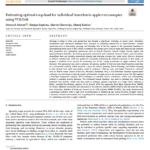
Dawood Ahmed; Ranjan Sapkota; Martin Churuvija; Manoj Karkee
Estimating optimal crop-load for individual branches in apple tree canopies using YOLOv8 Journal Article
In: Computers and Electronics in Agriculture, vol. 229, pp. 109697, 2025, ISSN: 0168-1699.
Abstract | Links | BibTeX | Tags:
@article{ahmed_estimating_2025,
title = {Estimating optimal crop-load for individual branches in apple tree canopies using YOLOv8},
author = {Dawood Ahmed and Ranjan Sapkota and Martin Churuvija and Manoj Karkee},
url = {https://www.sciencedirect.com/science/article/pii/S0168169924010883},
doi = {10.1016/j.compag.2024.109697},
issn = {0168-1699},
year = {2025},
date = {2025-02-01},
urldate = {2025-02-01},
journal = {Computers and Electronics in Agriculture},
volume = {229},
pages = {109697},
abstract = {Shortage of labor in fruit crop production has become a significant challenge in recent years. Therefore, mechanized and automated machines have emerged as promising alternatives to labor-intensive orchard operations such as harvesting, pruning, and thinning. One of the key aspects of the automated machines in accomplishing these tasks is their ability to identify tree canopy parts such as trunk and branches and estimate their geometric and topological parameters such as branch diameter, branch length, branch angles, and spacing between branches. By utilizing geometric parameters such as branch diameter, length, and orientation, researchers then can develop automated pruning and thinning systems that make more effective decisions to achieve optimal fruit yield and quality by accurately estimating the desired crop-load. In this study, we propose a machine vision system for estimating one of the canopy parameters in apple orchards: branch diameter. This parameter was used to estimate the optimal number of fruit that individual branches could bear in a commercial orchard, which provides a basis for robotic pruning, flower thinning, and fruitlet thinning so that desired fruit yield and quality could be achieved. Utilizing color and depth information collected with an RGB-D sensor (Azure Kinect DK, Microsoft, Redmond, WA), a YOLOv8-based instance segmentation technique was developed to identify trunks and branches of apple trees in the dormant season. We then applied a Principal Component Analysis (PCA) technique to estimate branch orientation, which was subsequently utilized to estimate branch diameter. The estimated branch diameter was used to calculate the Limb Cross Sectional Area (LCSA), which was then used to estimate optimal crop-load, as a larger LCSA indicates a higher potential fruit-bearing capacity of the branch. With this approach, Root Mean Squared Error (RMSE) for branch diameter estimation was calculated to be 2.06 mm (relative RMSE 10.82%) and the same for crop-load estimation (Number of fruits per branch) to be 3.93 (relative RMSE 22.25%). Our study demonstrated a promising workflow with a high level of performance in identifying and sizing branches of apple trees in a dynamic orchard environment and integrating farm management practices into automated decision-making for optimizing crop-load in apple orchards.},
keywords = {},
pubstate = {published},
tppubtype = {article}
}
2024
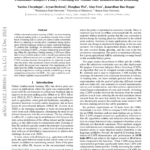
Yassine Chemingui; Aryan Deshwal; Honghao Wei; Alan Fern; Janardhan Rao Doppa
Constraint-Adaptive Policy Switching for Offline Safe Reinforcement Learning Proceedings Article
In: arXiv, 2024, (arXiv:2412.18946 [cs]).
Abstract | Links | BibTeX | Tags:
@inproceedings{chemingui_constraint-adaptive_2024,
title = {Constraint-Adaptive Policy Switching for Offline Safe Reinforcement Learning},
author = {Yassine Chemingui and Aryan Deshwal and Honghao Wei and Alan Fern and Janardhan Rao Doppa},
url = {http://arxiv.org/abs/2412.18946},
doi = {10.48550/arXiv.2412.18946},
year = {2024},
date = {2024-12-01},
urldate = {2024-12-01},
publisher = {arXiv},
abstract = {Offline safe reinforcement learning (OSRL) involves learning a decision-making policy to maximize rewards from a fixed batch of training data to satisfy pre-defined safety constraints. However, adapting to varying safety constraints during deployment without retraining remains an under-explored challenge. To address this challenge, we introduce constraint-adaptive policy switching (CAPS), a wrapper framework around existing offline RL algorithms. During training, CAPS uses offline data to learn multiple policies with a shared representation that optimize different reward and cost trade-offs. During testing, CAPS switches between those policies by selecting at each state the policy that maximizes future rewards among those that satisfy the current cost constraint. Our experiments on 38 tasks from the DSRL benchmark demonstrate that CAPS consistently outperforms existing methods, establishing a strong wrapper-based baseline for OSRL. The code is publicly available at https://github.com/yassineCh/CAPS.},
note = {arXiv:2412.18946 [cs]},
keywords = {},
pubstate = {published},
tppubtype = {inproceedings}
}
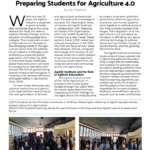
Lily Oliphant
Agricultural Innovations CDE: Preparing Students for Agriculture 4.0 Journal Article
In: NAAE The Agricultural Education Magazine, vol. 97, no. 3, pp. 43–45, 2024.
@article{oliphant_agricultural_2024,
title = {Agricultural Innovations CDE: Preparing Students for Agriculture 4.0},
author = {Lily Oliphant},
url = {https://www.naae.org/naae/document-server/?cfp=/naae/assets/file/public/magazine/volume%2097/aged%20magazine_novemberdecember%202024%20-%20final.pdf},
year = {2024},
date = {2024-12-01},
urldate = {2024-12-01},
journal = {NAAE The Agricultural Education Magazine},
volume = {97},
number = {3},
pages = {43\textendash45},
keywords = {},
pubstate = {published},
tppubtype = {article}
}
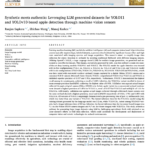
Ranjan Sapkota; Zhichao Meng; Manoj Karkee
Synthetic meets authentic: Leveraging LLM generated datasets for YOLO11 and YOLOv10-based apple detection through machine vision sensors Journal Article
In: Smart Agricultural Technology, vol. 9, pp. 100614, 2024, ISSN: 2772-3755.
Abstract | Links | BibTeX | Tags:
@article{sapkota_synthetic_2024,
title = {Synthetic meets authentic: Leveraging LLM generated datasets for YOLO11 and YOLOv10-based apple detection through machine vision sensors},
author = {Ranjan Sapkota and Zhichao Meng and Manoj Karkee},
url = {https://www.sciencedirect.com/science/article/pii/S2772375524002193},
doi = {10.1016/j.atech.2024.100614},
issn = {2772-3755},
year = {2024},
date = {2024-12-01},
urldate = {2024-12-01},
journal = {Smart Agricultural Technology},
volume = {9},
pages = {100614},
abstract = {Training machine learning (ML) models for artificial intelligence (AI) and computer vision-based object detection process typically requires large, labeled datasets, a process often burdened by significant human effort and high costs associated with imaging systems and image acquisition. This research aimed to simplify image data collection for object detection in orchards by avoiding traditional fieldwork with different imaging sensors. Utilizing OpenAI's DALLE, a large language model (LLM) for realistic image generation, we generated and annotated a cost-effective dataset. This dataset, exclusively generated by LLM, was then utilized to train two state-of-the-art deep learning models: YOLOV10 and YOLO11. The YOLO11 model for apple detection was trained with its five configurations (YOLO11n, YOLO11 s, YOLO11 m, YOLO11l and YOLO11x), and YOLOv10 model with its six configurations (YOLOv10n, YOLOv10 s, YOLOv10 m, YOLOv10b, YOLOv10l and YOLOv10x), which was then tested with real-world (outdoor orchard) images captured by a digital (Nikon D5100) camera and a consumer RGB-D camera (Microsoft Azure Kinect). YOLO11 outperformed YOLOv10 as YOLO11x and YOLO11n exhibited superior precision of 0.917 and 0.916, respectively. Furthermore, YOLO11l demonstrated the highest recall among its counterparts, achieving a recall of 0.889. Likewise, the YOLO11n variant excelled in terms of mean average precision (mAP@50), achieving the highest value of 0.958. Validation tests against actual images collected through a digital camera (Nikon D5100) over Scilate apple variety in a commercial orchard environment showed a highest precision of 0.874 for YOLO11 s, recall of 0.877 for YOLO11l and mAP@50 of 0.91 for YOLO11x. Additionally, validation test against actual images collected through a Microsoft Azure camera over the same orchard showed a highest precision, recall and mAP@50 respectively of 0.924, 0.781 and 0.855 with YOLO11x. All variants of YOLO11 surprisingly demonstrated a pre-processing time of just 0.2 milliseconds (ms), which was faster than any variant of YOLOv10. The fastest inference time for the YOLO11n model using the training dataset generated by the language model was 3.2 ms, while YOLOv10n, fastest among YOLOv10 variants, had a longer inference time of 5.5 ms. Likewise, the fastest inference time for the sensor-based images was 7.1 ms (for Nikon D5100 camera images) and 4.7 ms (for Azure images) with YOLO11n. This study presents a pathway for generating large image datasets using LLM in challenging agricultural fields with minimal or no labor-intensive efforts in field data-collection, which could accelerate the development and deployment of computer vision and robotic technologies in orchard environments.},
keywords = {},
pubstate = {published},
tppubtype = {article}
}
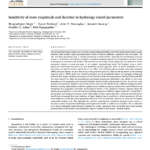
Bhupinderjeet Singh; Tanvir Ferdousi; John T. Abatzoglou; Samarth Swarup; Jennifer C. Adam; Kirti Rajagopalan
Sensitivity of snow magnitude and duration to hydrology model parameters Journal Article
In: Journal of Hydrology, vol. 645, pp. 132193, 2024, ISSN: 0022-1694.
Abstract | Links | BibTeX | Tags: AI, Water
@article{singh_sensitivity_2024,
title = {Sensitivity of snow magnitude and duration to hydrology model parameters},
author = {Bhupinderjeet Singh and Tanvir Ferdousi and John T. Abatzoglou and Samarth Swarup and Jennifer C. Adam and Kirti Rajagopalan},
url = {https://www.sciencedirect.com/science/article/pii/S0022169424015890},
doi = {10.1016/j.jhydrol.2024.132193},
issn = {0022-1694},
year = {2024},
date = {2024-12-01},
urldate = {2024-12-01},
journal = {Journal of Hydrology},
volume = {645},
pages = {132193},
abstract = {Process-based hydrology models are critical for understanding streamflow and water supply under global change. However, these models require parameterization which introduces additional uncertainty into the models. The role that these parameters play in driving uncertainty is under-studied, especially for intermediary processes outside of streamflow. An important example in snowmelt dominant regions are intermediary processes related to snowpack accumulation and ablation. We examine the sensitivity of snow magnitude and duration to eleven parameters relevant to snow processes in the coupled crop-hydrology model VIC-CropSyst using a hybrid global\textendashlocal Distributed Evaluation of Local Sensitivity Analysis approach. With the Pacific Northwest US as a case study, our specific research questions are: (a) What is the sensitivity response of peak snow water equivalent (SWE) and snow duration and how does it vary in the parameter space? (b) What are the key drivers of the sensitivity response? and (c) Which of the most sensitive parameters can we immediately improve by leveraging existing data products? Both target variables were sensitive to less than four of the eleven parameters. We found that peak SWE was most sensitive to either the precipitation partitioning temperature threshold or the albedo of new snow, depending on the geography and associated interplay between hydro-meteorological factors. In contrast, snow duration was primarily sensitive to the albedo of new snow and the albedo decay coefficient during snowmelt. Machine learning explainability workflows applied on the sensitivity response explained the model behavior and determined key geographic and hydro-meteorological drivers of the sensitivity response. Regions where the significant precipitation co-occurred with near-freezing temperature exhibited higher sensitivity of peak SWE to precipitation partitioning. In contrast, much colder high-elevation regions that have a delayed snowmelt-driven runoff when downward shortwave radiation is higher, displayed more sensitivity to albedo parameters. We also noted differences in the list of key parameters and in the level of sensitivity between our work and the limited comparable existing work. This highlights the need for comprehensive sensitivity analysis of snow metrics to become a routine component of hydrology model application studies in addition to streamflow. This is critical for us to better understand model behavior, identify key model parameters that can benefit from more dynamic representation in the models, and strategically improve models to best support decision-makers.},
keywords = {AI, Water},
pubstate = {published},
tppubtype = {article}
}
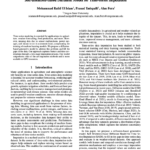
Mohammad Rafid Ul Islam; Prasad Tadepalli; Alan Fern
Self-attention-based Diffusion Model for Time-series Imputation Journal Article
In: Proceedings of the AAAI Symposium Series, vol. 4, no. 1, pp. 424–431, 2024, ISSN: 2994-4317, (Number: 1).
Abstract | Links | BibTeX | Tags:
@article{islam_self-attention-based_2024,
title = {Self-attention-based Diffusion Model for Time-series Imputation},
author = {Mohammad Rafid Ul Islam and Prasad Tadepalli and Alan Fern},
url = {https://ojs.aaai.org/index.php/AAAI-SS/article/view/31827},
doi = {10.1609/aaaiss.v4i1.31827},
issn = {2994-4317},
year = {2024},
date = {2024-11-01},
urldate = {2024-11-01},
journal = {Proceedings of the AAAI Symposium Series},
volume = {4},
number = {1},
pages = {424\textendash431},
abstract = {Time-series modeling is essential for applications in agriculture, weather forecasting, food production, and more. However, missing data due to sensor malfunctions, power outages, and human errors is a common issue, complicating the training of machine learning models. We propose a diffusion-based generative model to
address this problem and fill the gaps in the data. Our approach captures feature and time correlations through a two-stage imputation process. Our model outperforms state-of-the-art imputation methods and is more scalable in GPU resources.},
note = {Number: 1},
keywords = {},
pubstate = {published},
tppubtype = {article}
}
address this problem and fill the gaps in the data. Our approach captures feature and time correlations through a two-stage imputation process. Our model outperforms state-of-the-art imputation methods and is more scalable in GPU resources.
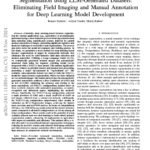
Ranjan Sapkota; Achyut Paudel; Manoj Karkee
2024, (arXiv:2411.11285).
Abstract | Links | BibTeX | Tags: Computer Science - Artificial Intelligence, Computer Science - Computer Vision and Pattern Recognition
@misc{sapkota_zero-shot_2024,
title = {Zero-Shot Automatic Annotation and Instance Segmentation using LLM-Generated Datasets: Eliminating Field Imaging and Manual Annotation for Deep Learning Model Development},
author = {Ranjan Sapkota and Achyut Paudel and Manoj Karkee},
url = {http://arxiv.org/abs/2411.11285},
doi = {10.48550/arXiv.2411.11285},
year = {2024},
date = {2024-11-01},
urldate = {2024-11-01},
publisher = {arXiv},
abstract = {Currently, deep learning-based instance segmentation for various applications (e.g., Agriculture) is predominantly performed using a labor-intensive process involving extensive field data collection using sophisticated sensors, followed by careful manual annotation of images, presenting significant logistical and financial challenges to researchers and organizations. The process also slows down the model development and training process. In this study, we presented a novel method for deep learning-based instance segmentation of apples in commercial orchards that eliminates the need for labor-intensive field data collection and manual annotation. Utilizing a Large Language Model (LLM), we synthetically generated orchard images and automatically annotated them using the Segment Anything Model (SAM) integrated with a YOLO11 base model. This method significantly reduces reliance on physical sensors and manual data processing, presenting a major advancement in "Agricultural AI". The synthetic, auto-annotated dataset was used to train the YOLO11 model for Apple instance segmentation, which was then validated on real orchard images. The results showed that the automatically generated annotations achieved a Dice Coefficient of 0.9513 and an IoU of 0.9303, validating the accuracy and overlap of the mask annotations. All YOLO11 configurations, trained solely on these synthetic datasets with automated annotations, accurately recognized and delineated apples, highlighting the method's efficacy. Specifically, the YOLO11m-seg configuration achieved a mask precision of 0.902 and a mask mAP@50 of 0.833 on test images collected from a commercial orchard. Additionally, the YOLO11l-seg configuration outperformed other models in validation on 40 LLM-generated images, achieving the highest mask precision and mAP@50 metrics. Keywords: YOLO, SAM, SAMv2, YOLO11, YOLOv11, Segment Anything, YOLO-SAM},
note = {arXiv:2411.11285},
keywords = {Computer Science - Artificial Intelligence, Computer Science - Computer Vision and Pattern Recognition},
pubstate = {published},
tppubtype = {misc}
}
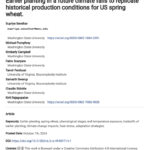
Supriya Savalkar; Michael Pumphrey; Kimberly Campbell; Fabio Scarpare; Tanvir Ferdousi; Samarth Swarup; Claudio Stöckle; Kirti Rajagopalan
Earlier planting in a future climate fails to replicate historical production conditions for US spring wheat. Journal Article
In: 2024, (ISSN: 2693-5015).
Abstract | Links | BibTeX | Tags:
@article{savalkar_earlier_2024,
title = {Earlier planting in a future climate fails to replicate historical production conditions for US spring wheat.},
author = {Supriya Savalkar and Michael Pumphrey and Kimberly Campbell and Fabio Scarpare and Tanvir Ferdousi and Samarth Swarup and Claudio St\"{o}ckle and Kirti Rajagopalan},
url = {https://www.researchsquare.com/article/rs-4940971/v1},
doi = {10.21203/rs.3.rs-4940971/v1},
year = {2024},
date = {2024-10-01},
urldate = {2024-10-01},
publisher = {Research Square},
abstract = {Global warming can increase crop heat stress exposure, adversely affecting crop yields and quality. Earlier planting is widely considered in climate change literature as a potential adaptation strategy by shifting the growing season to cooler periods. However, the effectiveness of earlier planting in achieving overall temperature exposures during the crop growth season that are at least as favorable as historical conditions remains unclear. Our objective is to comprehensively assess the potential effectiveness of earlier planting as an adaptation strategy by addressing two key questions: How effective is earlier planting in reducing exposure to excessively high temperatures across different growth stages? What are the associated tradeoffs in temperature exposure, and can historical conditions be matched with this adaptation strategy? We focus on major US spring wheat growing states as a case study, analyzing lethal, critical, suboptimal, and optimal temperature thresholds by growth stage to quantify the impact of earlier planting. Our findings indicate that while earlier planting does reduce exposure to critical and lethal high-temperature categories during some reproductive stages, it generally fails to replicate historical production conditions for the majority of the US spring wheat production region (contributing 85% of the current production). This trend persists across all future time frames and emission scenarios. The Pacific Northwest US presents an exception; however, even in this region, certain early and late growth stages may experience worse-than-historical conditions requiring management. The planting window with historically equivalent temperature exposures also narrows from 11 weeks to 1-7 weeks, presenting additional logistical challenges. Given that the Pacific Northwest US accounts for less than 15% of the national spring wheat production, at a national scale, current production levels are unlikely to be sustained by primarily relying on earlier planting as an adaptation strategy. This is a critical consideration, especially given that many climate change assessments frequently list earlier planting as an effective adaptation strategy without the capacity to fully explore its tradeoffs. Exploring additional adaptation strategies to maintain current national production levels is important.},
note = {ISSN: 2693-5015},
keywords = {},
pubstate = {published},
tppubtype = {article}
}